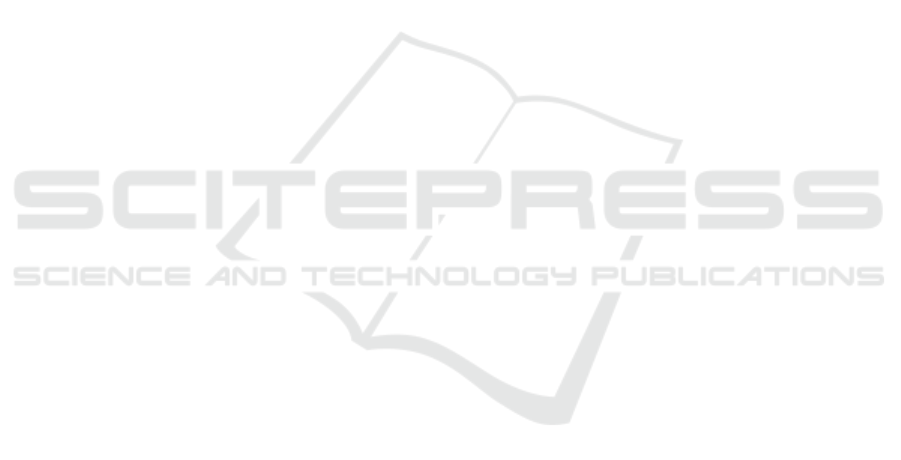
Boussik, A., Ben-Messaoud, W., Niar, S., and Taleb-
Ahmed, A. (2021). Railway obstacle detection using
unsupervised learning: An exploratory study. In 2021
IEEE Intelligent Vehicles Symposium (IV), pages 660–
667. IEEE.
Brucker, M., Cramariuc, A., Von Einem, C., Siegwart,
R., and Cadena, C. (2023). Local and global infor-
mation in obstacle detection on railway tracks. In
2023 IEEE/RSJ International Conference on Intelli-
gent Robots and Systems (IROS), pages 9049–9056.
IEEE.
Buslaev, A., Iglovikov, V. I., Khvedchenya, E., Parinov, A.,
Druzhinin, M., and Kalinin, A. A. (2020). Albumen-
tations: fast and flexible image augmentations. Infor-
mation, 11(2):125.
Cai, H., Lin, J., Lin, Y., Liu, Z., Tang, H., Wang, H., Zhu, L.,
and Han, S. (2022). Enable deep learning on mobile
devices: Methods, systems, and applications. ACM
Transactions on Design Automation of Electronic Sys-
tems (TODAES), 27(3):1–50.
Cimpoi, M., Maji, S., Kokkinos, I., Mohamed, S., and
Vedaldi, A. (2014). Describing textures in the wild.
In Proceedings of the IEEE conference on computer
vision and pattern recognition, pages 3606–3613.
Fedynyak, V., Romanus, Y., Hlovatskyi, B., Sydor, B.,
Dobosevych, O., Babin, I., and Riazantsev, R.
(2024). Devos: Flow-guided deformable transformer
for video object segmentation. In Proceedings of
the IEEE/CVF Winter Conference on Applications of
Computer Vision, pages 240–249.
Guo, Q., Chen, Y., and Liao, S. (2023). Enhancing mobile
privacy and security: A face skin patch-based anti-
spoofing approach. In 2023 IEEE International Con-
ference on Cloud Computing Technology and Science
(CloudCom), pages 52–57. IEEE.
Kingma, D. P. and Ba, J. (2014). Adam: A
method for stochastic optimization. arXiv preprint
arXiv:1412.6980.
Kirillov, A., Mintun, E., Ravi, N., Mao, H., Rolland, C.,
Gustafson, L., Xiao, T., Whitehead, S., Berg, A. C.,
Lo, W.-Y., et al. (2023). Segment anything. In Pro-
ceedings of the IEEE/CVF International Conference
on Computer Vision, pages 4015–4026.
Lis, K., Honari, S., Fua, P., and Salzmann, M. (2023). Per-
spective aware road obstacle detection. IEEE Robotics
and Automation Letters, 8(4):2150–2157.
Redmon, J., Divvala, S., Girshick, R., and Farhadi, A.
(2016). You only look once: Unified, real-time object
detection. In Proceedings of the IEEE conference on
computer vision and pattern recognition, pages 779–
788.
Sazara, C., Cetin, M., and Iftekharuddin, K. M. (2019). De-
tecting floodwater on roadways from image data with
handcrafted features and deep transfer learning. In
2019 IEEE intelligent transportation systems confer-
ence (ITSC), pages 804–809. IEEE.
Sevilla-Lara, L., Sun, D., Jampani, V., and Black, M. J.
(2016). Optical flow with semantic segmentation and
localized layers. In Proceedings of the IEEE con-
ference on computer vision and pattern recognition,
pages 3889–3898.
Shao, S., Li, Z., Zhang, T., Peng, C., Yu, G., Zhang, X.,
Li, J., and Sun, J. (2019). Objects365: A large-
scale, high-quality dataset for object detection. In Pro-
ceedings of the IEEE/CVF international conference
on computer vision, pages 8430–8439.
Sudre, C. H., Li, W., Vercauteren, T., Ourselin, S., and
Jorge Cardoso, M. (2017). Generalised dice over-
lap as a deep learning loss function for highly unbal-
anced segmentations. In Deep Learning in Medical
Image Analysis and Multimodal Learning for Clini-
cal Decision Support: Third International Workshop,
DLMIA 2017, and 7th International Workshop, ML-
CDS 2017, Held in Conjunction with MICCAI 2017,
Qu
´
ebec City, QC, Canada, September 14, Proceed-
ings 3, pages 240–248. Springer.
Teed, Z. and Deng, J. (2020). Raft: Recurrent all-pairs
field transforms for optical flow. In Computer Vision–
ECCV 2020: 16th European Conference, Glasgow,
UK, August 23–28, 2020, Proceedings, Part II 16,
pages 402–419. Springer.
Zhang, Q., Yan, F., Song, W., Wang, R., and Li, G. (2023).
Automatic obstacle detection method for the train
based on deep learning. Sustainability, 15(2):1184.
A Universal Railway Obstacle Detection System Based on Optical-Flow Guided Semi-Supervised Segmentation
265