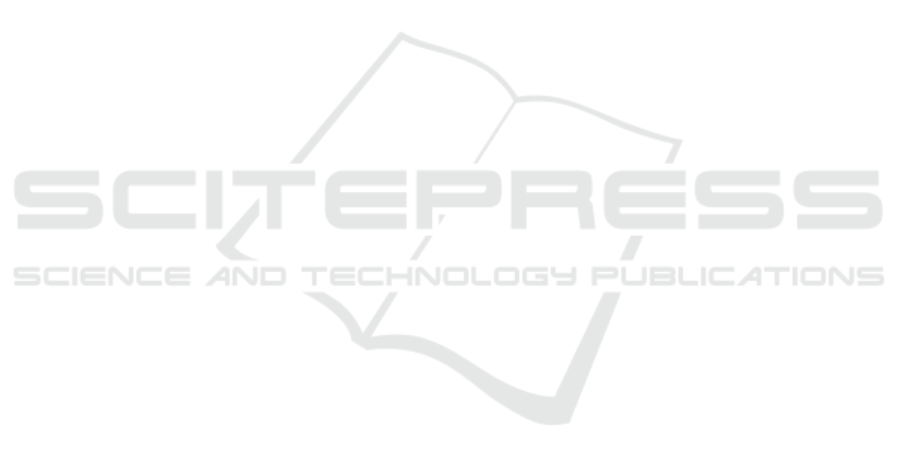
5 CONCLUSION
In conclusion, partial UDA methods have been
adapted and evaluated on AR and OE tasks aiming
to provide energy management, security, and HVAC
systems optimization for smart buildings. This work
has several contributions. Indeed, it is the first to
adapt ARPDA, ARSDA, and SAN approaches from
2-dimensional space to 1-dimensional and evaluate
them with partial and standard UDA. This research
has provided new architectures for the features extrac-
tor, classifier, and discriminator modules that fit IoT
data. The newly adapted approaches can be applied to
any 1-D data and are not restricted to smart building
data. Partial UDA methods that have been adapted
solve a real issue related to negative transfer which is
common in smart buildings data. Also, a comparison
analysis between the findings of the adapted meth-
ods with partial and standard UDA as well as previ-
ous UDA methods. The adapted UPDA methods have
outstanding performances with scores up to 98%. In
future work, we consider UPDA methods applied to
smart building tasks with more outlier labels in source
domains and compare the findings with the current re-
search.
REFERENCES
Ali, S. and Bouguila, N. (2020). Online learning for
beta-liouville hidden markov models: Incremental
variational learning for video surveillance and action
recognition. In 2020 IEEE International Conference
on Image Processing (ICIP), pages 3249–3253. IEEE.
Amayri, M. and Ploix, S. (2018). Decision tree and
parametrized classifier for estimating occupancy in
energy management. In 2018 5th International Con-
ference on Control, Decision and Information Tech-
nologies (CoDIT), pages 397–402. IEEE.
Amayri, M., Ploix, S., Najar, F., Bouguila, N., and Wurtz,
F. (2019). A statistical process control chart ap-
proach for occupancy estimation in smart buildings.
In 2019 IEEE Symposium Series on Computational
Intelligence (SSCI), pages 1729–1734. IEEE.
Cao, Z., Long, M., Wang, J., and Jordan, M. I. (2018).
Partial transfer learning with selective adversarial net-
works. In Proceedings of the IEEE conference on
computer vision and pattern recognition, pages 2724–
2732.
Chen, Z., Zhu, Q., Masood, M. K., and Soh, Y. C. (2017).
Environmental sensors-based occupancy estimation in
buildings via ihmm-mlr. IEEE Transactions on Indus-
trial Informatics, 13(5):2184–2193.
Cook, D. J. (2010). Learning setting-generalized activity
models for smart spaces. IEEE intelligent systems,
2010(99):1.
Dridi, J., Amayri, M., and Bouguila, N. (2022). Transfer
learning for estimating occupancy and recognizing ac-
tivities in smart buildings. Building and Environment,
217:109057.
Dridi, J., Amayri, M., and Bouguila, N. (2023a). Unsuper-
vised domain adaptation with and without access to
source data for estimating occupancy and recognizing
activities in smart buildings. Building and Environ-
ment, 243:110651.
Dridi, J., Amayri, M., and Bouguila, N. (2023b). Unsuper-
vised domain adaptation without source data for esti-
mating occupancy and recognizing activities in smart
buildings. Energy and Buildings, page 113808.
Gu, X., Yu, X., Sun, J., Xu, Z., et al. (2021). Adversarial
reweighting for partial domain adaptation. Advances
in Neural Information Processing Systems, 34:14860–
14872.
Kazmi, H., Mehmood, F., and Amayri, M. (2017). Smart
home futures: Algorithmic challenges and opportu-
nities. In 2017 14th International Symposium on
Pervasive Systems, Algorithms and Networks & 2017
11th International Conference on Frontier of Com-
puter Science and Technology & 2017 Third Inter-
national Symposium of Creative Computing (ISPAN-
FCST-ISCC), pages 441–448. IEEE.
Li, S., Liu, C. H., Lin, Q., Wen, Q., Su, L., Huang, G., and
Ding, Z. (2020). Deep residual correction network for
partial domain adaptation. IEEE transactions on pat-
tern analysis and machine intelligence, 43(7):2329–
2344.
Prabhakaran, K., Dridi, J., Amayri, M., and Bouguila, N.
(2022). Explainable k-means clustering for occupancy
estimation. Procedia Computer Science, 203:326–
333.
Wang, A., Chen, G., Yang, J., Zhao, S., and Chang, C.-Y.
(2016). A comparative study on human activity recog-
nition using inertial sensors in a smartphone. IEEE
Sensors Journal, 16(11):4566–4578.
Zamzami, N., Amayri, M., Bouguila, N., and Ploix, S.
(2019). Online clustering for estimating occupancy
in an office setting. In 2019 IEEE 28th International
Symposium on Industrial Electronics (ISIE), pages
2195–2200. IEEE.
SMARTGREENS 2025 - 14th International Conference on Smart Cities and Green ICT Systems
76