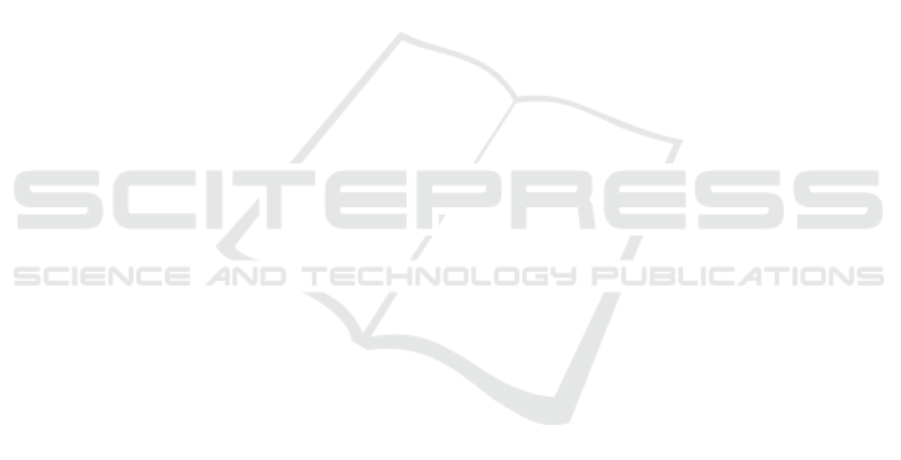
and artifact issues, as different models may offer vary-
ing performance trade-offs. Additionally, training a
GAN from scratch, rather than relying on pre-trained
models, could offer alternative approaches that may
better suit the specific dataset and improve perfor-
mance.
ACKNOWLEDGEMENTS
The authors thank the Fundac¸
˜
ao de Amparo
`
a
Pesquisa do Estado de Minas Gerais (FAPEMIG),
project APQ00234-18, for the financial support that
made this research possible.
ChatGPT was carefully used to refine and clarify
the English expressions in the text, ensuring the ideas
were communicated accurately. Additionally, Chat-
PDF was thoughtfully employed to identify key sec-
tions of the references, which helped the authors ad-
dress the findings effectively and make well-informed
comparisons of the results.
REFERENCES
Correa, V., Funk, P., Sundelius, N., Sohlberg, R., and
Ramos, A. (2024). Applications of gans to aid tar-
get detection in sar operations: A systematic literature
review. Drones, 8(9):448.
Correa, V. H. G. (2024). Application of real-esrgan in im-
proving ir sensor images for use in sar operations.
Master’s thesis, Federal University of Itajub
´
a, Brazil.
Dousai, N. M. K. and Lon
ˇ
cari
´
c, S. (2022). Detecting hu-
mans in search and rescue operations based on ensem-
ble learning. IEEE access, 10:26481–26492.
Fadnavis, S. (2014). Image interpolation techniques in dig-
ital image processing: an overview. International
Journal of Engineering Research and Applications,
4(10):70–73.
Kulkarni, S., Chaphekar, V., Chowdhury, M. M. U., Erden,
F., and Guvenc, I. (2020). Uav aided search and res-
cue operation using reinforcement learning. In 2020
SoutheastCon, volume 2, pages 1–8. IEEE.
Lucas, A., Lopez-Tapia, S., Molina, R., and Katsaggelos,
A. K. (2019). Generative adversarial networks and
perceptual losses for video super-resolution. IEEE
Transactions on Image Processing, 28(7):3312–3327.
Luo, Z. and Tian, Y. (2024a). Improved infrared road object
detection algorithm based on attention mechanism in
yolov8. IAENG International Journal of Computer
Science, 51(6).
Luo, Z. and Tian, Y. (2024b). Infrared road object detec-
tion based on improved yolov8. IAENG International
Journal of Computer Science, 51(3).
Lygouras, E., Santavas, N., Taitzoglou, A., Tarchanidis, K.,
Mitropoulos, A., and Gasteratos, A. (2019). Unsuper-
vised human detection with an embedded vision sys-
tem on a fully autonomous uav for search and rescue
operations. Sensors, 19(16):3542.
Pensieri, M. G., Garau, M., and Barone, P. M. (2020).
Drones as an integral part of remote sensing technolo-
gies to help missing people. Drones, 4(2):15.
Rahim, A. N. A., Yaakob, S. N., Ngadiran, R., and Nas-
ruddin, M. W. (2015). An analysis of interpolation
methods for super resolution images. In 2015 IEEE
Student Conference on Research and Development
(SCOReD), pages 72–77. IEEE.
Schoonmaker, J., Reed, S., Podobna, Y., Vazquez, J., and
Boucher, C. (2010). A multispectral automatic tar-
get recognition application for maritime surveillance,
search, and rescue. In Sensors, and Command, Con-
trol, Communications, and Intelligence (C3I) Tech-
nologies for Homeland Security and Homeland De-
fense IX, volume 7666, pages 374–384. SPIE.
Shen, L., Lang, B., and Song, Z. (2023). Infrared object
detection method based on dbd-yolov8. IEEE Access.
Svedin, J., Bernland, A., Gustafsson, A., Claar, E., and
Luong, J. (2021). Small uav-based sar system using
low-cost radar, position, and attitude sensors with on-
board imaging capability. International Journal of Mi-
crowave and Wireless Technologies, 13(6):602–613.
Wang, F., Wang, H., Qin, Z., and Tang, J. (2023). Uav target
detection algorithm based on improved yolov8. IEEE
Access.
Wang, J., Gao, K., Zhang, Z., Ni, C., Hu, Z., Chen, D., and
Wu, Q. (2021a). Multisensor remote sensing imagery
super-resolution with conditional gan. Journal of Re-
mote Sensing.
Wang, X., Xie, L., Dong, C., and Shan, Y. (2021b). Real-
esrgan: Training real-world blind super-resolution
with pure synthetic data. In Proceedings of the
IEEE/CVF international conference on computer vi-
sion, pages 1905–1914.
Wang, X., Yu, K., Wu, S., Gu, J., Liu, Y., Dong, C., Qiao,
Y., and Change Loy, C. (2018). Esrgan: Enhanced
super-resolution generative adversarial networks. In
Proceedings of the European conference on computer
vision (ECCV) workshops, pages 0–0.
Wang, Z., Jiang, K., Yi, P., Han, Z., and He, Z. (2020).
Ultra-dense gan for satellite imagery super-resolution.
Neurocomputing, 398:328–337.
Xue, X., Zhang, X., Li, H., and Wang, W. (2020). Research
on gan-based image super-resolution method. In 2020
IEEE International Conference on Artificial Intelli-
gence and Computer Applications (ICAICA), pages
602–605. IEEE.
Zhang, X., Feng, C., Wang, A., Yang, L., and Hao, Y.
(2021). Ct super-resolution using multiple dense
residual block based gan. Signal, Image and Video
Processing, 15:725–733.
Zhao, X., Zhang, W., Zhang, H., Zheng, C., Ma, J., and
Zhang, Z. (2024). Itd-yolov8: An infrared target de-
tection model based on yolov8 for unmanned aerial
vehicles. Drones, 8(4):161.
VISAPP 2025 - 20th International Conference on Computer Vision Theory and Applications
254