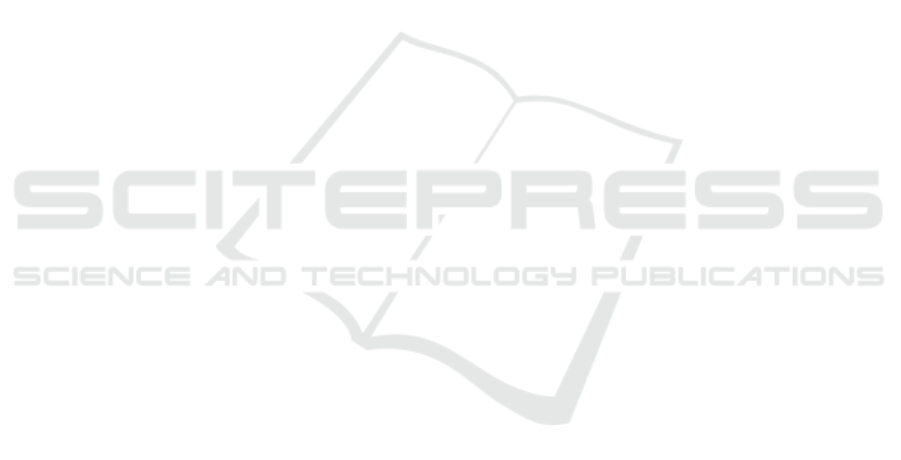
ACKNOWLEDGEMENTS
This research is part of the CADAIVision SBO
project funded and supported by Flanders Make vzw,
the strategic research center for the manufacturing in-
dustry.
REFERENCES
AlliedMarketResearch (2024). Construction vehicles mar-
ket size, share, competitive landscape and trend anal-
ysis report, by solution, by equipment, by type, by ap-
plication and, by industry : Global opportunity anal-
ysis and industry forecast, 2023-2032. Construction
Vehicles Market.
Baker, V. and Guzzomi, A. L. (2013). A model and com-
parison of 4-wheel-drive fixed-chassis tractor rollover
during phase i. Biosystems Engineering, 116(2):179–
189.
Bennett, N., Walawalkar, A., and Schindler, C. (2014). Pay-
load estimation in excavators: Model-based evalua-
tion of current payload estimation systems.
Cordos¸, N. and TodoruT¸ , A. (2019). Influences of the sus-
pensions characteristics on the vehicle stability. In
Burnete, N. and Varga, B. O., editors, Proceedings
of the 4th International Congress of Automotive and
Transport Engineering (AMMA 2018), pages 808–
813, Cham. Springer International Publishing.
Cuong, D., Zhu, S., Hung, D., and Ngoc, N. (2013). Study
on the vertical stiffness and damping coefficient of
tractor tire using semi-empirical model. Hue Univer-
sity Journal of Science, 83:5–15.
Edwards, D., Parn, E. A., Sing, M. C., and Thwala, W. D.
(2019). Risk of excavators overturning: Determining
horizontal centrifugal force when slewing freely sus-
pended loads. Engineering, construction, and archi-
tectural management, 26(3):479–498.
Ferlibas, M. and Ghabcheloo, R. (2021). The 17th scandi-
navian international conference on fluid power. Hue
University Journal of Science.
Gralak, M. and Walu
´
s, K. (2024). A model of an extending
front loader. Applied Sciences, 14:3948.
Huo, D., Chen, J., Zhang, H., Shi, Y., and Wang, T. (2023).
Intelligent prediction for digging load of hydraulic ex-
cavators based on rbf neural network. Measurement,
206:112210.
Ishihara, S., Kanazawa, A., and Narikawa, R. (2021). Re-
alization of excavator loading operation by nonlinear
model predictive control with bucket load estimation.
In IFAC-PapersOnLine, volume 54, pages 20–25. El-
sevier Ltd.
Lysych, M. N. (2020). Study driving dynamics of the
machine-tractor unit on a virtual stand with ob-
stacles. Journal of Physics: Conference Series,
1515(4):42079–.
MarkwideResearch (2024). Construction vehicles mar-
ket analysis- industry size, share, research report, in-
sights, covid-19 impact, statistics, trends, growth and
forecost 2024-2032. Automotive and Transportation,
pages 1–263.
Mitrev, R. and Marinkovi
´
c, D. (2019). Numerical study
of the hydraulic excavator overturning stability during
performing lifting operations. Advances in mechani-
cal engineering, 11(5):168781401984177–133.
Murphy, D. (2022). Tractor Stability and Instability. https:
//extension.psu.edu/tractor-stability-and-instability.
Updated : July 15, 2022.
New Holland Agriculture (2024). Front Axle and
Suspension. https://agriculture.newholland.com/
apac/th-th/equipment/products/agricultural-tractors/
t7-heavy-duty/detail/front-axle-and-suspension.
Accessed: 2024-08-06.
Pavlov, N. and Dacova, D. (2021). A multibody model of
a wheel loader with pneumatic boom suspension and
proving ground testing. IOP Conference Series: Ma-
terials Science and Engineering, 1031:012009.
Robyns, S., Heerwegh, W., and Weckx, S. (2024). A dig-
ital twin of an off highway vehicle based on a low
cost camera. Procedia Computer Science, 232:2366–
2375. 5th International Conference on Industry 4.0
and Smart Manufacturing (ISM 2023).
Tafazoli, S., Lawrence, P. D., and Salcudean, S. E.
(1999). Identification of inertial and friction parame-
ters for excavator arms. IEEE Trans. Robotics Autom.,
15:966–971.
Zhu, Q., Yang, C., Hu, H., and Wu, X. (2021). Building a
novel dynamics rollover model for critical instability
state analysis of articulated multibody vehicles. Inter-
national Journal of Heavy Vehicle Systems, 28:329–
352.
Real-Time Digital Twin for Construction Vehicle Stability Assessment and Visualization with Improved Front-Loader Payload Estimation
277