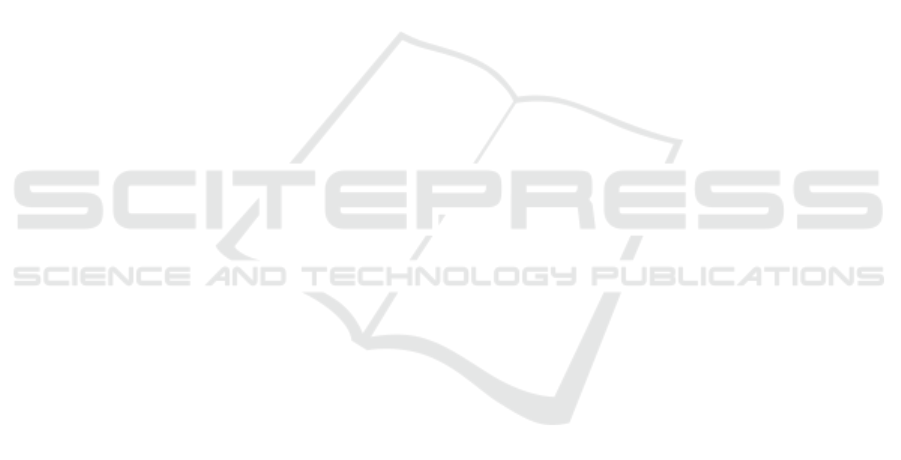
Chen, S., Han, Z., He, B., Buckley, M., Torr, P., Tresp, V.,
and Gu, J. (2024). Understanding and Improving In-
Context Learning on Vision-language Models. arXiv.
Chicco Davide and Jurman Giuseppe (2020). The advan-
tages of the Matthews correlation coefficient (MCC)
over F1 score and accuracy in binary classification
evaluation. BMC genomics, 21:1–13.
Defard, T., Setkov, A., Loesch, A., and Audigier, R. (2021).
Padim: A patch distribution modeling framework for
anomaly detection and localization. In International
Conference on Pattern Recognition, pages 475–489.
Springer.
Deng, J., Dong, W., Socher, R., Li, L.-J., Li, K., and Fei-
Fei, L. (2009). Imagenet: A large-scale hierarchical
image database. In IEEE/CVF Conference on Com-
puter Vision and Pattern Recognition, pages 248–255.
Dong, Q., Li, L., Dai, D., Zheng, C., Wu, Z., Chang, B.,
Sun, X., Xu, J., Li, L., and Sui, Z. (2023). A Survey
on In-context Learning.
G
¨
osgens, M., Zhiyanov, A., Tikhonov, A., and
Prokhorenkova, L. (2022). Good Classification
Measures and How to Find Them. neural information
processing systems, 34(17136-17147).
Grandini, M., Bagli, E., and Visani, G. (2020). Metrics for
Multi-Class Classification: An Overview.
Gu, Z., Zhu, B., Zhu, G., Chen, Y., Tang, M., and
Wang, J. (2024). AnomalyGPT: Detecting Indus-
trial Anomalies Using Large Vision-Language Mod-
els. In AAAI Conference on Artificial Intelligence, vol-
ume 38, pages 1932–1940. arXiv.
He, K., Zhang, X., Ren, S., and Sun, J. (2015). Deep Resid-
ual Learning for Image Recognition. In IEEE/CVF
Conference on Computer Vision and Pattern Recogni-
tion, pages 770–778.
Hu, E. J., Shen, Y., Wallis, P., Allen-Zhu, Z., Li, Y., Wang,
S., Wang, L., and Chen, W. (2021). LoRA: Low-Rank
Adaptation of Large Language Models.
Jeong, J., Zou, Y., Kim, T., Zhang, D., Ravichandran,
A., and Dabeer, O. (2023). WinCLIP: Zero-/Few-
Shot Anomaly Classification and Segmentation. In
IEEE/CVF Conference on Computer Vision and Pat-
tern Recognition, pages 19606–19616. arXiv.
Li, B., Zhang, Y., Chen, L., Wang, J., Pu, F., Yang, J., Li,
C., and Liu, Z. (2023a). MIMIC-IT: Multi-Modal In-
Context Instruction Tuning.
Li, B., Zhang, Y., Chen, L., Wang, J., Yang, J., and Liu,
Z. (2023b). Otter: A Multi-Modal Model with In-
Context Instruction Tuning.
Liu, H., Li, C., Li, Y., and Lee, Y. J. (2024a). Improved
Baselines with Visual Instruction Tuning.
Liu, H., Li, C., Wu, Q., and Lee, Y. J. (2023). Visual In-
struction Tuning. In Advances in Neural Information
Processing Systems, volume 36. arXiv.
Liu, Y., Duan, H., Zhang, Y., Li, B., Zhang, S., Zhao, W.,
Yuan, Y., Wang, J., He, C., Liu, Z., Chen, K., and Lin,
D. (2024b). MMBench: Is Your Multi-modal Model
an All-around Player?
Loshchilov, I. and Hutter, F. (2019). Decoupled Weight De-
cay Regularization.
Meta (2023). Llama 2: Open Foundation and Fine-Tuned
Chat Models.
OpenAI (2023). GPT-4 Technical Report.
Pengzhen Ren, Xiao, Y., Chang, X., Huang, P.-Y., Li, Z.,
Gupta, B. B., Chen, X., and Wang, X. (2021). A Sur-
vey of Deep Active Learning. ACM computing surveys
(CSUR), 54(9):1–40.
Radford, A., Kim, J. W., Hallacy, C., Ramesh, A., Goh, G.,
Agarwal, S., Sastry, G., Askell, A., Mishkin, P., Clark,
J., Krueger, G., and Sutskever, I. (2021). Learning
Transferable Visual Models From Natural Language
Supervision. In International Conference on Machine
Learning, pages 8748–8763.
Roth, K., Pemula, L., Zepeda, J., Sch
¨
olkopf, B., Brox,
T., and Gehler, P. (2022). Towards total recall in
industrial anomaly detection. In Proceedings of the
IEEE/CVF Conference on Computer Vision and Pat-
tern Recognition, pages 14318–14328.
Sokolova, M., Japkowicz, N., and Szpakowicz, S. (2006).
Beyond Accuracy, F-Score and ROC: A Family of
Discriminant Measures for Performance Evaluation.
In AI 2006: Advances in Artificial Intelligence, Lec-
ture Notes in Computer Science, volume 4304, pages
1015–1021.
Steck, H., Ekanadham, C., and Kallus, N. (2024). Is Cosine-
Similarity of Embeddings Really About Similarity?
In Companion Proceedings of the ACM on Web Con-
ference 2024, pages 887–890.
Tai, Y., Fan, W., Zhang, Z., Zhu, F., Zhao, R., and Liu,
Z. (2023). Link-Context Learning for Multimodal
LLMs.
Ueno, S., Yamada, Y., Nakatsuka, S., and Kato, K. (2023).
Benchmarking of Query Strategies: Towards Future
Deep Active Learning.
XTuner Contributors (2023). XTuner: A Toolkit for Effi-
ciently Fine-tuning LLM.
Yang, Z., Gan, Z., Wang, J., Hu, X., Lu, Y., Liu, Z., and
Wang, L. (2022). An Empirical Study of GPT-3 for
Few-Shot Knowledge-Based VQA. In AAAI Confer-
ence on Artificial Intelligence, volume 36 of 3, pages
3081–3089. arXiv.
Yi, J. and Yoon, S. (2020). Patch SVDD: Patch-level SVDD
for Anomaly Detection and Segmentation. In Asian
Conference on Computer Vision (ACCV). arXiv.
Yin, S., Fu, C., Zhao, S., Li, K., Sun, X., Xu, T., and Chen,
E. (2024). A Survey on Multimodal Large Language
Models.
Zong, Y., Bohdal, O., and Hospedales, T. (2024). VL-
ICL Bench: The Devil in the Details of Benchmarking
Multimodal In-Context Learning.
Zou, Y., Jeong, J., Pemula, L., Zhang, D., and Dabeer,
O. (2022). SPot-the-Difference Self-Supervised Pre-
training for Anomaly Detection and Segmentation.
In European Conference on Computer Vision, pages
392–408. arXiv.
VISAPP 2025 - 20th International Conference on Computer Vision Theory and Applications
260