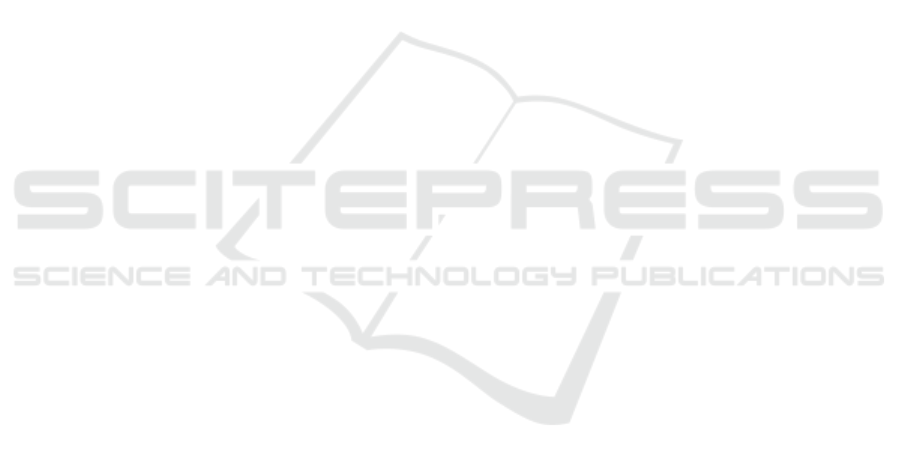
ACKNOWLEDGEMENTS
This work was supported in part by the Ministry
of Innovation and Technology National Research,
Development and Innovation Office (NRDI) within
the framework of the Autonomous Systems National
Laboratory Program RRF-2.3.1-21-2022-00002.
Supported by the
´
UNKP-23-4-I-BME-158 New
National Excellence Program of the Ministry for Cul-
ture and Innovation from the source of the National
Research, Development and Innovation Fund.
The study was prepared with the professional sup-
port of the Doctoral Student Scholarship Program of
the Co-operative Doctoral Program of the Ministry
for Innovation and Technology from the source of
the National Research, Development and Innovation
Fund.
This work was supported by Jaguar Land Rover
Hungary Ltd organization.
REFERENCES
5G Automotive Association (5GAA) (2023). TR C-V2X
Use Cases and Service Level Requirements Vol III
V1.0. Technical report, 5GAA.
Bae, J.-K., Park, M.-C., Yang, E.-J., and Seo, D.-W.
(2020). Implementation and performance evalua-
tion for DSRC-based vehicular communication sys-
tem. IEEE Access, 9:6878–6887.
Bazzi, A., Masini, B. M., and Zanella, A. (2017). Coop-
erative awareness in the internet of vehicles for safety
enhancement. EAI Endorsed Transactions on Internet
of Things, 3(9):e5–e5.
Cui, L., Hu, J., Park, B. B., and Bujanovic, P. (2018).
Development of a simulation platform for safety im-
pact analysis considering vehicle dynamics, sensor
errors, and communication latencies: Assessing co-
operative adaptive cruise control under cyber attack.
Transportation research part C: emerging technolo-
gies, 97:1–22.
de Almeida, T. T., de Carvalho Gomes, L., Ortiz, F. M.,
J
´
unior, J. G. R., and Costa, L. H. M. K. (2022). Com-
parative Analysis of a Vehicular Safety Application
in NS-3 and Veins. IEEE Transactions on Intelligent
Transportation Systems, 23:620–629.
ETSI, E. (2014). 302 637-2 v1. 3.1-intelligent transport
systems (ITS); vehicular communications; basic set
of applications; part 2: Specification of cooperative
awareness basic service. ETSI, Sept.
Fallah, Y. P. and Gani, S. M. O. (2018). Efficient and High
Fidelity DSRC Simulation. Wireless Networks.
Fitah, A., Badri, A., Moughit, M., and Sahel, A. (2018).
Performance of DSRC and WIFI for Intelligent Trans-
port Systems in VANET. Procedia Computer Science,
127:360–368.
Ghasemi, A., Abedi, A., and Ghasemi, F. (2016). Propa-
gation Engineering in Wireless Communications, Sec-
ond Edition. Springer.
IEEE (2012). IEEE Standard for Information technology–
Telecommunications and information exchange be-
tween systems Local and metropolitan area networks–
Specific requirements Part 11: Wireless LAN Medium
Access Control (MAC) and Physical Layer (PHY)
Specifications.
Knowles Flanagan, S., Tang, Z., He, J., and Yusoff, I.
(2021). Investigating and modeling of cooperative
vehicle-to-vehicle safety stopping distance. Future In-
ternet, 13(3):68.
Kryszkiewicz, P., Sroka, P., Sybis, M., and Kliks, A.
(2022). Path loss and shadowing modeling for
vehicle-to-vehicle communications in terrestrial TV
band. IEEE Transactions on Antennas and Propaga-
tion, 71(1):984–998.
Llatser, I., Michalke, T., Dolgov, M., Wildsch
¨
utte, F., and
Fuchs, H. (2019). Cooperative automated driving use
cases for 5G V2X communication. In 2019 IEEE 2nd
5G World Forum (5GWF), pages 120–125. IEEE.
Mannoni, V., Berg, V., Sesia, S., and Perraud, E. (2019). A
comparison of the V2X communication systems: ITS-
G5 and C-V2X. In 2019 IEEE 89th Vehicular Technol-
ogy Conference (VTC2019-Spring), pages 1–5. IEEE.
Rayamajhi, A., Biron, Z. A., Merco, R., Pisu, P., Westall,
J. M., and Martin, J. (2018). The impact of dedicated
short range communication on cooperative adaptive
cruise control. In 2018 IEEE International Confer-
ence on Communications (ICC), pages 1–7. IEEE.
Saponara, S. and Gagliardi, A. (2018). Modeling and analy-
sis of 802.11p physical layer for V2X connected trans-
port systems considering harsh operating conditions
and HW device performance. In 2018 International
Conference of Electrical and Electronic Technologies
for Automotive, pages 1–6. IEEE.
Shagdar, O., Nashashibi, F., and Tohme, S. (2017). Perfor-
mance study of CAM over IEEE 802.11p for cooper-
ative adaptive cruise control. In 2017 Wireless Days,
pages 70–76. IEEE.
Xing, H., Ploeg, J., and Nijmeijer, H. (2019). Compensa-
tion of communication delays in a cooperative ACC
system. IEEE Transactions on Vehicular Technology,
69(2):1177–1189.
Xue, S., Gong, S., and Li, X. (2024). A Comparative Study
of IEEE 802.11bd and IEEE 802.11p on the Data Dis-
semination Properties in Dynamic Traffic Scenarios.
Applied Sciences.
Investigating the Safety Effects of Degraded Wireless Performance on Connected Longitudinal Driver Assistance Functions
285