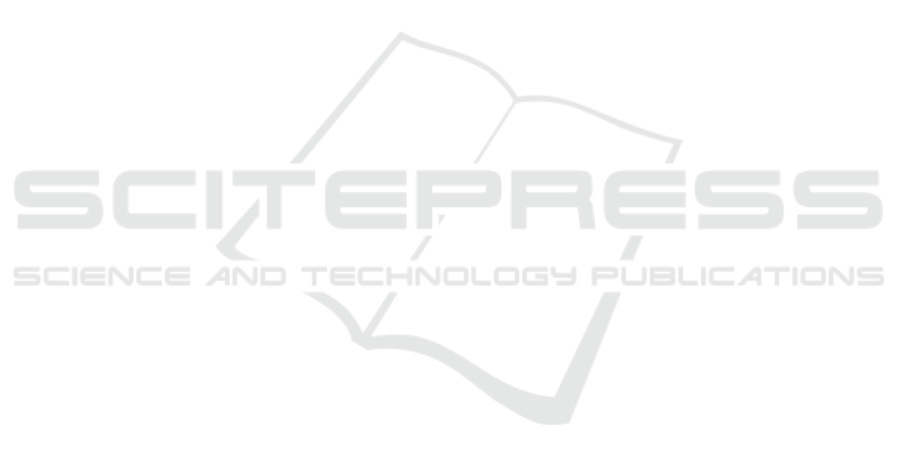
with uncertainty. As the method is not limited to data
from a certain domain, we were able to demonstrate
its expressiveness on data from diverse domains.
Animated 2DTFs open many avenues for fu-
ture work in uncertainty visualization. Instead of
the standard deviation the level-crossing probability
(LCP) (P
¨
othkow and Hege, 2011) could be used for
the y-axis of the 2DTF. The advantage of the LCP is
its capability to deal with non-parametric models of
uncertainty. The interpretation of the animation and
visualization would be a challenge for this research.
We present only a limited amount of widgets for an-
imation. Further widget designs and animation types
(scale, rotate, translate) could be used to explore the
complex data sets with uncertainty in other meaning-
ful ways. It is obvious that animation works well for
highlighting, nevertheless analyzing which animation
speed is most effective could be interesting.
REFERENCES
Akiba, H., Wang, C., and Ma, K.-L. (2010). Aniviz: A
template-based animation tool for volume visualiza-
tion. IEEE CG&A, 30(5):61–71.
Athawale, T. M., Ma, B., Sakhaee, E., Johnson, C. R., and
Entezari, A. (2021). Direct volume rendering with
nonparametric models of uncertainty. IEEE TVCG,
27(2):1797–1807.
Bonneau, G.-P., Hege, H.-C., Johnson, C. R., Oliveira,
M. M., Potter, K., Rheingans, P., and Schultz, T.
(2014). Overview and state-of-the-art of uncertainty
visualization. In Mathematics and Visualization,
pages 3–27. Springer London.
Brown, R. (2004). Animated visual vibrations as an uncer-
tainty visualisation technique. In Proc. of the 2nd Int.
Conf. on Compu. Graphics and Interactive Techniques
in Australasia and South East Asia, GRAPHITE ’04,
page 84–89, New York, NY, USA. ACM.
Correa, C. and Silver, D. (2005). Dataset traversal with
motion-controlled transfer functions. In VIS 05. IEEE
Visualization, 2005., pages 359–366.
Djurcilov, S., Kim, K., Lermusiaux, P., and Pang, A. (2002).
Visualizing scalar volumetric data with uncertainty.
Computers & Graphics, 26(2):239–248.
Ehlschlaeger, C. R., Shortridge, A. M., and Goodchild,
M. F. (1997). Visualizing spatial data uncertainty us-
ing animation. Comp. i. GeoSciences, 23(4):387–395.
Gershon, N. (1992). Visualization of fuzzy data using gen-
eralized animation. In Proc. Visu. ’92, pages 268–273.
H
¨
agele, D., Schulz, C., Beschle, C., Booth, H., Butt, M.,
Barth, A., Deussen, O., and Weiskopf, D. (2022). Un-
certainty visualization: Fundamentals and recent de-
velopments. it - Information Techn., 64(4-5):121–132.
Kamal, A., Dhakal, P., Javaid, A. Y., Devabhaktuni, V. K.,
Kaur, D., Zaientz, J., and Marinier, R. (2021). Recent
advances and challenges in uncertainty visualization:
a survey. Journal of Visualization, 24(5):861–890.
Kavur, A. E., Selver, M. A., Dicle, O., Barıs¸, M., and Gezer,
N. S. (2019). CHAOS - Combined (CT-MR) Healthy
Abdominal Organ Segmentation Challenge Data.
Kniss, J., Kindlmann, G., and Hansen, C. (2002). Multi-
dimensional transfer functions for interactive volume
rendering. IEEE TVCG, 8(3):270–285.
Knoll, A., Hijazi, Y., Kensler, A., Schott, M., Hansen, C.,
and Hagen, H. (2009). Fast ray tracing of arbitrary
implicit surfaces with interval and affine arithmetic.
Computer Graphics Forum, 28(1):26–40.
Liu, S., Levine, J. A., Bremer, P.-T., and Pascucci, V.
(2012). Gaussian mixture model based volume visu-
alization. In IEEE LDAV, pages 73–77.
Lundstr
¨
om, C., Ljung, P., Persson, A., and Ynnerman, A.
(2007). Uncertainty visualization in medical volume
rendering using probabilistic animation. IEEE TVCG,
13(6):1648–1655.
Meyer-Spradow, J., Ropinski, T., Mensmann, J., and Hin-
richs, K. (2009). Voreen: A rapid-prototyping envi-
ronment for ray-casting-based volume visualizations.
IEEE CG&A, 29(6):6–13.
Palmer, T. N., Alessandri, A., and et al. (2004).
DEVELOPMENT OF A EUROPEAN MULTI-
MODEL ENSEMBLE SYSTEM FOR SEASONAL-
TO-INTERANNUAL PREDICTION (DEMETER).
Bulletin of the Am. Meteorolog. Soc., 85(6):853–872.
Pang, A. T., Wittenbrink, C. M., and Lodha, S. K. (1997).
Approaches to uncertainty visualization. The Visual
Computer, 8(13):370–390.
Pfaffelmoser, T., Reitinger, M., and Westermann, R. (2011).
Visualizing the positional and geometrical variability
of isosurfaces in uncertain scalar fields. Computer
Graphics Forum, 30(3):951–960.
P
¨
othkow, K., Weber, B., and Hege, H.-C. (2011). Proba-
bilistic marching cubes. Computer Graphics Forum,
30(3):931–940.
P
¨
othkow, K. and Hege, H.-C. (2011). Positional uncertainty
of isocontours: Condition analysis and probabilistic
measures. IEEE TVCG, 17(10):1393–1406.
Ramamurthy, M. and Lakshminarayanan, V. (2015). Hu-
man Vision and Perception, page 1–23. Springer In-
ternational Publishing.
Sakhaee, E. and Entezari, A. (2017). A statistical direct
volume rendering framework for visualization of un-
certain data. IEEE TVCG, 23(12):2509–2520.
Sikachev, P., Rautek, P., Bruckner, S., and Gr
¨
oller, M. E.
(2010). Dynamic focus+context for volume rendering.
Vision, Modeling and Visualization, pages 331–338.
Woodring, J. and Shen, H.-W. (2007). Incorporating high-
lighting animations into static visualizations. In Visu-
alization and Data Analysis 2007, volume 6495, page
649503. Int. Soc. for Optics and Photonics, SPIE.
IVAPP 2025 - 16th International Conference on Information Visualization Theory and Applications
806