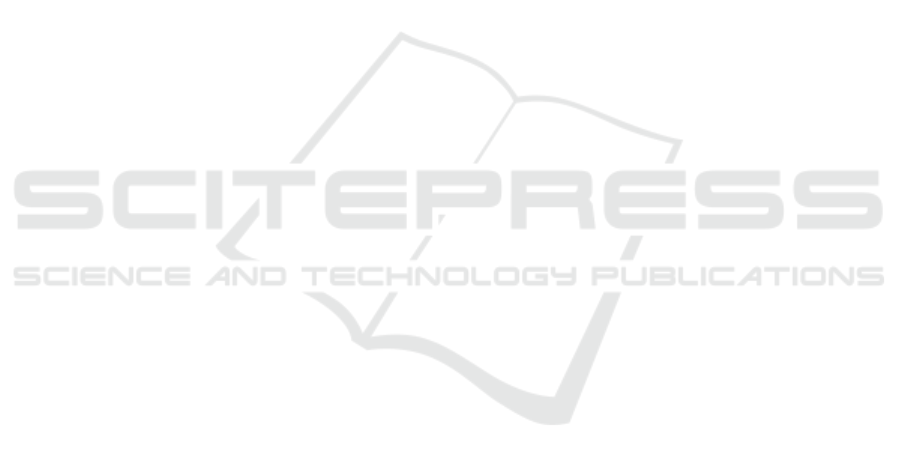
Sukthankar, R., Schmid, C., and Malik, J. (2018).
Ava: A video dataset of spatio-temporally localized
atomic visual actions. In 2018 IEEE/CVF Conference
on Computer Vision and Pattern Recognition, pages
6047–6056.
Jhuang, H., Gall, J., Zuffi, S., Schmid, C., and Black, M. J.
(2013). Towards understanding action recognition. In
2013 IEEE International Conference on Computer Vi-
sion, pages 3192–3199.
Kalogeiton, V., Weinzaepfel, P., Ferrari, V., and Schmid,
C. (2017). Action tubelet detector for spatio-temporal
action localization. In Proceedings of the IEEE Inter-
national Conference on Computer Vision (ICCV).
Kay, W., Carreira, J., Simonyan, K., Zhang, B., Hillier, C.,
Vijayanarasimhan, S., Viola, F., Green, T., Back, T.,
Natsev, P., Suleyman, M., and Zisserman, A. (2017).
The kinetics human action video dataset. CoRR,
abs/1705.06950.
K
¨
op
¨
ukl
¨
u, O., Wei, X., and Rigoll, G. (2021). You only
watch once: A unified cnn architecture for real-time
spatiotemporal action localization.
Li, Y., Wang, Z., Wang, L., and Wu, G. (2020). Ac-
tions as moving points. In Vedaldi, A., Bischof, H.,
Brox, T., and Frahm, J.-M., editors, Computer Vision
– ECCV 2020, pages 68–84, Cham. Springer Interna-
tional Publishing.
Lin, T., Maire, M., Belongie, S. J., Hays, J., Perona, P.,
Ramanan, D., Doll
´
ar, P., and Zitnick, C. L. (2014).
Microsoft COCO: common objects in context. In
Fleet, D. J., Pajdla, T., Schiele, B., and Tuytelaars,
T., editors, Computer Vision - ECCV 2014 - 13th
European Conference, Zurich, Switzerland, Septem-
ber 6-12, 2014, Proceedings, Part V, volume 8693 of
Lecture Notes in Computer Science, pages 740–755.
Springer.
Singh, G., Choutas, V., Saha, S., Yu, F., and Van Gool, L.
(2023). Spatio-temporal action detection under large
motion. In Proceedings of the IEEE/CVF Winter Con-
ference on Applications of Computer Vision (WACV),
pages 6009–6018.
Singh, G., Saha, S., Sapienza, M., Torr, P. H. S., and Cuz-
zolin, F. (2017). Online real-time multiple spatiotem-
poral action localisation and prediction. In Proceed-
ings of the IEEE International Conference on Com-
puter Vision (ICCV).
Sohn, K. (2016). Improved deep metric learning with multi-
class n-pair loss objective. In Lee, D., Sugiyama,
M., Luxburg, U., Guyon, I., and Garnett, R., editors,
Advances in Neural Information Processing Systems,
volume 29. Curran Associates, Inc.
Sun, C., Shrivastava, A., Vondrick, C., Murphy, K., Suk-
thankar, R., and Schmid, C. (2018). Actor-centric re-
lation network. In Ferrari, V., Hebert, M., Sminchis-
escu, C., and Weiss, Y., editors, Computer Vision –
ECCV 2018, pages 335–351, Cham. Springer Interna-
tional Publishing.
Wu, C.-Y., Feichtenhofer, C., Fan, H., He, K., Krahenbuhl,
P., and Girshick, R. (2019). Long-term feature banks
for detailed video understanding. In Proceedings of
the IEEE/CVF Conference on Computer Vision and
Pattern Recognition (CVPR).
Yang, A., Miech, A., Sivic, J., Laptev, I., and Schmid, C.
(2022). TubeDETR: Spatio-Temporal Video Ground-
ing with Transformers. In 2022 IEEE/CVF Con-
ference on Computer Vision and Pattern Recogni-
tion (CVPR), pages 16421–16432, New Orleans, LA,
USA. IEEE.
Zhao, J., Zhang, Y., Li, X., Chen, H., Shuai, B., Xu, M.,
Liu, C., Kundu, K., Xiong, Y., Modolo, D., Mar-
sic, I., Snoek, C. G., and Tighe, J. (2022). Tuber:
Tubelet transformer for video action detection. In
2022 IEEE/CVF Conference on Computer Vision and
Pattern Recognition (CVPR), pages 13588–13597.
VISAPP 2025 - 20th International Conference on Computer Vision Theory and Applications
268