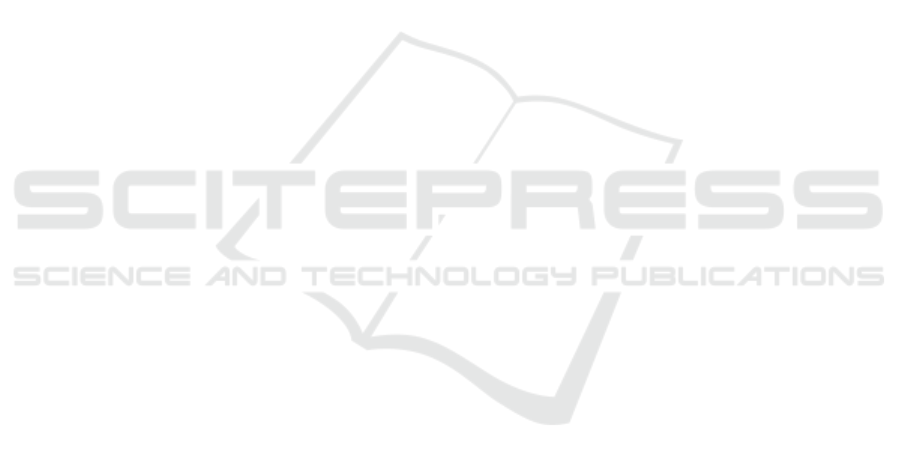
tional conference on machine learning, pages 3040–
3049. PMLR.
Kim, Y. G., Lee, S., Son, J., Bae, H., and Do Chung, B.
(2020). Multi-agent system and reinforcement learn-
ing approach for distributed intelligence in a flexible
smart manufacturing system. Journal of Manufactur-
ing Systems, 57:440–450.
K
¨
olle, M., Matheis, T., Altmann, P., and Schmid, K. (2023).
Learning to participate through trading of reward
shares. In Proceedings of the 15th International Con-
ference on Agents and Artificial Intelligence, ICAART
’23, pages 355–362. SciTePress.
Kuhnle, A., Richley, J., and Perez-Lavin, D. (2023).
Learning strategic value and cooperation in multi-
player stochastic games through side payments. arXiv
preprint arXiv:2303.05307.
Lashkari, B. and Musilek, P. (2021). A comprehensive re-
view of blockchain consensus mechanisms. IEEE Ac-
cess, 9:43620–43652.
Laurent, G. J., Matignon, L., Fort-Piat, L., et al. (2011). The
world of independent learners is not markovian. Inter-
national Journal of Knowledge-based and Intelligent
Engineering Systems, 15(1):55–64.
Leibo, J. Z., Zambaldi, V., Lanctot, M., Marecki, J., and
Graepel, T. (2017). Multi-agent reinforcement learn-
ing in sequential social dilemmas. In Proceedings of
the 16th Conference on Autonomous Agents and Mul-
tiAgent Systems, pages 464–473.
Lerer, A. and Peysakhovich, A. (2017). Maintaining coop-
eration in complex social dilemmas using deep rein-
forcement learning. arXiv preprint arXiv:1707.01068.
Letcher, A., Foerster, J., Balduzzi, D., Rockt
¨
aschel, T., and
Whiteson, S. (2019). Stable opponent shaping in dif-
ferentiable games. In International Conference on
Learning Representations.
Li, Q., Cascudo, I., and Christensen, M. G. (2019). Privacy-
preserving distributed average consensus based on ad-
ditive secret sharing. In 2019 27th European Signal
Processing Conference (EUSIPCO), pages 1–5. IEEE.
Li, Y. and Tan, C. (2019). A survey of the consensus for
multi-agent systems. Systems Science & Control En-
gineering, 7:468 – 482.
Littman, M. L. (2001). Value-function reinforcement learn-
ing in markov games. Cognitive systems research,
2(1):55–66.
Lupu, A. and Precup, D. (2020). Gifting in multi-agent
reinforcement learning. In Proceedings of the 19th
International Conference on autonomous agents and
multiagent systems, pages 789–797.
Merhej, R. and Chetouani, M. (2021). Lief: Learning to in-
fluence through evaluative feedback. In Adaptive and
Learning Agents Workshop (AAMAS 2021).
Monrat, A. A., Schel
´
en, O., and Andersson, K. (2019). A
survey of blockchain from the perspectives of appli-
cations, challenges, and opportunities. IEEE Access,
7:117134–117151.
No
¨
e, R. (2006). Cooperation experiments: coordination
through communication versus acting apart together.
Animal behaviour, 71(1):1–18.
Olfati-Saber, R. and Shamma, J. S. (2005). Consensus fil-
ters for sensor networks and distributed sensor fusion.
In Proceedings of the 44th IEEE Conference on Deci-
sion and Control, pages 6698–6703. IEEE.
Omitaomu, O. A. and Niu, H. (2021). Artificial intelligence
techniques in smart grid: A survey. Smart Cities,
4(2):548–568.
Perolat, J., Leibo, J. Z., Zambaldi, V., Beattie, C., Tuyls, K.,
and Graepel, T. (2017). A multi-agent reinforcement
learning model of common-pool resource appropria-
tion.
Phan, T., Ritz, F., Belzner, L., Altmann, P., Gabor, T., and
Linnhoff-Popien, C. (2021). Vast: Value function fac-
torization with variable agent sub-teams. In Advances
in Neural Information Processing Systems, NeurIPS
’21, pages 24018–24032. Curran Associates, Inc.
Phan, T., Sommer, F., Altmann, P., Ritz, F., Belzner, L.,
and Linnhoff-Popien, C. (2022). Emergent coop-
eration from mutual acknowledgment exchange. In
Proceedings of the 21st International Conference on
Autonomous Agents and Multiagent Systems, pages
1047–1055.
Phan, T., Sommer, F., Ritz, F., Altmann, P., N
¨
ußlein,
J., K
¨
olle, M., Belzner, L., and Linnhoff-Popien, C.
(2024). Emergent cooperation from mutual acknowl-
edgment exchange in multi-agent reinforcement learn-
ing. Autonomous Agents and Multi-Agent Systems,
38(34).
Qureshi, K. N. and Abdullah, A. H. (2013). A survey on in-
telligent transportation systems. Middle-East Journal
of Scientific Research, 15(5):629–642.
Rashid, T., Samvelyan, M., De Witt, C. S., Farquhar, G., Fo-
erster, J., and Whiteson, S. (2020). Monotonic value
function factorisation for deep multi-agent reinforce-
ment learning. The Journal of Machine Learning Re-
search, 21(1):7234–7284.
Russell, S. J. (2010). Artificial intelligence a modern ap-
proach. Pearson Education, Inc.
Salimitari, M. and Chatterjee, M. (2018). A survey on con-
sensus protocols in blockchain for iot networks. arXiv
preprint arXiv:1809.05613.
Sandholm, T. W. and Crites, R. H. (1996). Multiagent rein-
forcement learning in the iterated prisoner’s dilemma.
Biosystems, 37(1-2):147–166.
Schenato, L. and Gamba, G. (2007). A distributed consen-
sus protocol for clock synchronization in wireless sen-
sor network. In 2007 46th ieee conference on decision
and control, pages 2289–2294. IEEE.
Sunehag, P., Lever, G., Gruslys, A., Czarnecki, W. M.,
Zambaldi, V., Jaderberg, M., Lanctot, M., Sonnerat,
N., Leibo, J. Z., Tuyls, K., and Graepel, T. (2018).
Value-decomposition networks for cooperative multi-
agent learning based on team reward. In Proceedings
of the 17th International Conference on Autonomous
Agents and MultiAgent Systems, page 2085–2087.
Tawalbeh, L., Muheidat, F., Tawalbeh, M., and Quwaider,
M. (2020). Iot privacy and security: Challenges and
solutions. Applied Sciences, 10(12):4102.
Wang, J. X., Hughes, E., Fernando, C., Czarnecki, W. M.,
Du
´
e
˜
nez Guzm
´
an, E. A., and Leibo, J. Z. (2019).
MEDIATE: Mutually Endorsed Distributed Incentive Acknowledgment Token Exchange
43