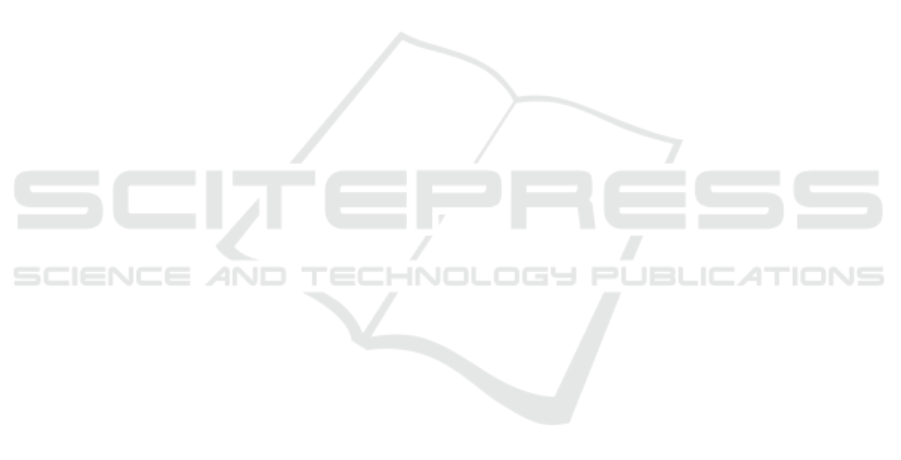
tional Conference on INnovations in Intelligent Sys-
Tems and Applications (INISTA), pages 1–6. IEEE.
Imtiaz, S., Taj, I. A., and Nawaz, R. (2021). Visible to
thermal image synthesis using light weight pyramid
network. In 2021 16th International Conference on
Emerging Technologies (ICET), pages 1–5. IEEE.
Jolicoeur-Martineau, A. (2018). The relativistic discrimina-
tor: a key element missing from standard gan. arXiv
preprint arXiv:1807.00734.
Kri
ˇ
sto, M., Ivasic-Kos, M., and Pobar, M. (2020). Thermal
object detection in difficult weather conditions using
yolo. IEEE access, 8:125459–125476.
Li, X., Li, J., Li, Y., Ozcan, A., and Jarrahi, M. (2023).
High-throughput terahertz imaging: progress and
challenges. Light: Science & Applications, 12(1):233.
Liu, J., Fan, X., Huang, Z., Wu, G., Liu, R., Zhong, W., and
Luo, Z. (2022). Target-aware dual adversarial learn-
ing and a multi-scenario multi-modality benchmark to
fuse infrared and visible for object detection. In Pro-
ceedings of the IEEE/CVF Conference on Computer
Vision and Pattern Recognition, pages 5802–5811.
Liu, R., Ge, Y., Choi, C. L., Wang, X., and Li, H. (2021).
Divco: Diverse conditional image synthesis via con-
trastive generative adversarial network. In Proceed-
ings of the IEEE/CVF Conference on Computer Vi-
sion and Pattern Recognition (CVPR), pages 16377–
16386.
Low, S., Nina, O., Bowald, D., Sappa, A. D., Inkawhich, N.,
and Bruns, P. (2024). Multi-modal aerial view image
challenge: Sensor domain translation. In Proceedings
of the IEEE/CVF Conference on Computer Vision and
Pattern Recognition, pages 3096–3104.
Low, S., Nina, O., Sappa, A. D., Blasch, E., and Inkawhich,
N. (2023). Multi-modal aerial view image chal-
lenge: Translation from synthetic aperture radar to
electro-optical domain results - PBVS 2023. In 2023
IEEE/CVF Conference on Computer Vision and Pat-
tern Recognition Workshops (CVPRW), pages 515–
523.
Mizginov, V. and Danilov, S. Y. (2019). Synthetic ther-
mal background and object texture generation using
geometric information and GAN. The International
Archives of the Photogrammetry, Remote Sensing and
Spatial Information Sciences, 42:149–154.
Pavez, V., Hermosilla, G., Silva, M., and Farias, G. (2023).
Advanced deep learning techniques for high-quality
synthetic thermal image generation. Mathematics,
11(21):4446.
Pons, G., El Ali, A., and Cesar, P. (2020). ET-CycleGAN:
Generating thermal images from images in the visi-
ble spectrum for facial emotion recognition. In Com-
panion publication of the international conference on
multimodal interaction, pages 87–91.
Pradawet, C., Khongdee, N., Pansak, W., Spreer, W., Hilger,
T., and Cadisch, G. (2023). Thermal imaging for as-
sessment of maize water stress and yield prediction
under drought conditions. Journal of Agronomy and
Crop Science, 209(1):56–70.
Qu, Y., Meng, Y., Fan, H., and Xu, R. X. (2022).
Low-cost thermal imaging with machine learning
for non-invasive diagnosis and therapeutic monitor-
ing of pneumonia. Infrared Physics & Technology,
123:104201.
Rippa, M., Pagliarulo, V., Lanzillo, A., Grilli, M., Fati-
gati, G., Rossi, P., Cennamo, P., Trojsi, G., Ferraro,
P., and Mormile, P. (2021). Active thermography for
non-invasive inspection of an artwork on poplar panel:
novel approach using principal component thermogra-
phy and absolute thermal contrast. Journal of Nonde-
structive Evaluation, 40(1):21.
Su
´
arez, P. L., Carpio, D., and Sappa, A. (2023a). A
deep learning based approach for synthesizing realis-
tic depth maps. In International Conference on Image
Analysis and Processing, pages 369–380. Springer.
Su
´
arez, P. L., Carpio, D., and Sappa, A. (2023b). Depth
map estimation from a single 2d image. In 2023
17th International Conference on Signal-Image Tech-
nology & Internet-Based Systems (SITIS), pages 347–
353. IEEE.
Su
´
arez, P. L. and Sappa, A. D. (2023). Toward a thermal
image-like representation. In VISIGRAPP (4: VIS-
APP), pages 133–140.
Teutsch, M., Sappa, A. D., and Hammoud, R. I. (2022).
Computer vision in the infrared spectrum: challenges
and approaches. Springer.
Tuzcuo
˘
glu,
¨
O., K
¨
oksal, A., Sofu, B., Kalkan, S., and Alatan,
A. A. (2024). Xoftr: Cross-modal feature matching
transformer. In Proceedings of the IEEE/CVF Con-
ference on Computer Vision and Pattern Recognition,
pages 4275–4286.
Wang, Z., Bovik, A. C., Sheikh, H. R., and Simoncelli, E. P.
(2004). Image quality assessment: from error visi-
bility to structural similarity. IEEE Transactions on
Image Processing, 13(4):600–612.
Yang, L., Kang, B., Huang, Z., Xu, X., Feng, J., and Zhao,
H. (2024). Depth anything: Unleashing the power
of large-scale unlabeled data. In Proceedings of the
IEEE/CVF Conference on Computer Vision and Pat-
tern Recognition, pages 10371–10381.
Yin, W., Zhang, J., Wang, O., Niklaus, S., Mai, L., Chen,
S., and Shen, C. (2021). Learning to recover 3d scene
shape from a single image. In Proceedings of the
IEEE/CVF Conference on Computer Vision and Pat-
tern Recognition, pages 204–213.
Zhang, L., Gonzalez-Garcia, A., Van De Weijer, J., Danell-
jan, M., and Khan, F. S. (2018). Synthetic data gener-
ation for end-to-end thermal infrared tracking. Trans-
actions on Image Processing, 28(4):1837–1850.
Zhu, J.-Y., Park, T., Isola, P., and Efros, A. A. (2017).
Unpaired image-to-image translation using cycle-
consistent adversarial networks. In Proceedings of
the IEEE international conference on computer vi-
sion, pages 2223–2232.
VISAPP 2025 - 20th International Conference on Computer Vision Theory and Applications
282