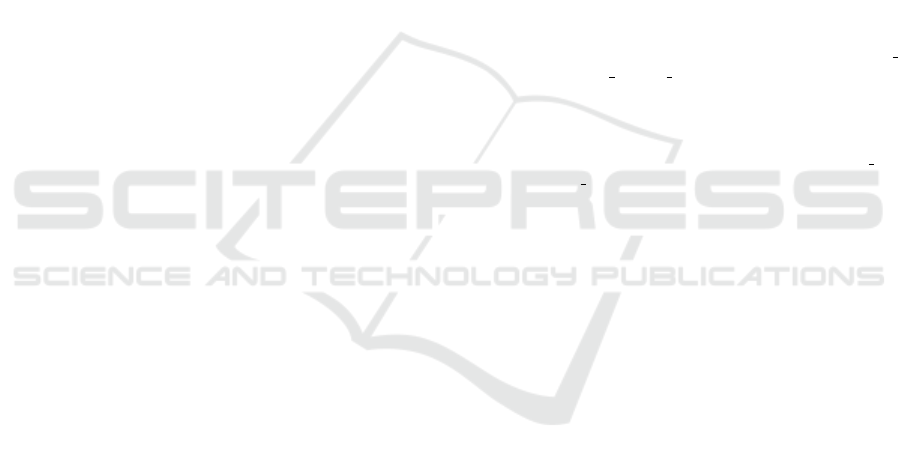
object-level access control vulnerabilities in web ap-
plications. A2CT can be applied to a wide range of
web applications, requires only a small configuration
effort, and can detect vulnerabilities in the context of
all relevant HTTP request types (GET, POST, PUT,
PATCH, DELETE). During our evaluation in the con-
text of 30 publicly available web applications, we
managed to detect 14 previously unknown vulnerabil-
ities in two of these applications, which resulted in six
newly published CVE records. This demonstrates the
soundness of the solution approach and the practical
applicability of A2CT.
To our knowledge, A2CT is the first approach that
combines a high degree of automation, broad applica-
bility, and demonstrated capabilities to detect access
control vulnerabilities in practice. To encourage fur-
ther research in this direction, A2CT is made avail-
able to the community under an open-source license.
ACKNOWLEDGEMENTS
This work was partly funded by the Swiss Confed-
eration’s innovation promotion agency Innosuissse
(project 48528.1 IP-ICT).
REFERENCES
Alalfi, M. H., Cordy, J. R., and Dean, T. R. (2012). Auto-
mated Verification of Role-Based Access Control Se-
curity Models Recovered from Dynamic Web Appli-
cations. In 2012 14th IEEE International Symposium
on Web Systems Evolution (WSE), pages 1–10, Trento,
Italy.
Atlidakis, V., Godefroid, P., and Polishchuk, M. (2019).
RESTler: Stateful REST API Fuzzing. In 2019
IEEE/ACM 41st International Conference on Soft-
ware Engineering (ICSE), pages 748–758.
Bennets, S. (2023). Open Source Web Scanners. https:
//github.com/psiinon/open-source-web-scanners.
Deng, G., Zhang, Z., Li, Y., Liu, Y., Zhang, T., Liu, Y.,
Yu, G., and Wang, D. (2023). NAUTILUS: Auto-
mated RESTful API Vulnerability Detection. In 32nd
USENIX Security Symposium (USENIX Security 23),
pages 5593–5609, Anaheim, CA. USENIX Associa-
tion.
Docker (2024). Docker. https://www.docker.com.
Kushnir, M., Favre, O., Rennhard, M., Esposito, D., and
Zahnd, V. (2021). Automated Black Box Detection
of HTTP GET Request-based Access Control Vulner-
abilities in Web Applications. In Proceedings of the
7th International Conference on Information Systems
Security and Privacy - ICISSP, pages 204–216.
Le, H. T., Nguyen, C. D., Briand, L., and Hourte, B. (2015).
Automated Inference of Access Control Policies for
Web Applications. In Proceedings of the 20th ACM
Symposium on Access Control Models and Technolo-
gies, SACMAT ’15, pages 27–37, Vienna, Austria.
Li, X., Si, X., and Xue, Y. (2014). Automated Black-Box
Detection of Access Control Vulnerabilities in Web
Applications. In Proceedings of the 4th ACM Confer-
ence on Data and Application Security and Privacy,
CODASPY ’14, pages 49–60, San Antonio, USA.
Liu, Y., Li, Y., Deng, G., Liu, Y., Wan, R., Wu, R., Ji, D.,
Xu, S., and Bao, M. (2022). Morest: Model-based
RESTful API Testing with Execution Feedback. ICSE
’22, page 1406–1417, New York, NY, USA. Associa-
tion for Computing Machinery.
Mautic (2024). Mautic. https://www.mautic.org.
mitmproxy (2024). mitmproxy. https://mitmproxy.org.
MITRE (2024). CVE – Common Vulnerabilities and Expo-
sures. https://www.cve.org.
Noseevich, G. and Petukhov, A. (2011). Detecting In-
sufficient Access Control in Web Applications. In
2011 First SysSec Workshop, pages 11–18, Amster-
dam, Netherlands.
OWASP (2021a). OWASP Top 10 – A01:2021 – Bro-
ken Access Control. https://owasp.org/Top10/A01
2021-Broken Access Control.
OWASP (2021b). OWASP Top 10:2021. https://owasp.org/
Top10.
OWASP (2024). Vulnerability Scanning Tools.
https://owasp.org/www-community/Vulnerability
Scanning Tools.
Playwright (2024a). Playwright. https://playwright.dev.
Playwright (2024b). Playwright Codgen. https://
playwright.dev/docs/codegen##running-codegen.
Portswigger (2024). Access Control Vulnerabilities
and Privilege Escalation. https://portswigger.net/
web-security/access-control.
Puppeteer (2024). Puppeteer. https://pptr.dev.
Rennhard, M., Kushnir, M., Favre, O., Esposito, D., and
Zahnd, V. (2022). Automating the Detection of Ac-
cess Control Vulnerabilities in Web Applications. SN
Computer Science, 3(5):376.
Scrapy (2024). Scrapy. https://scrapy.org.
SQLite (2024). SQLite. https://www.sqlite.org.
Unifiedtransform (2024). Unifiedtransform. https://
changeweb.github.io/Unifiedtransform.
Verizon (2023). 2023 Data Breach Investigation
Report. https://www.verizon.com/business/en-gb/
resources/reports/dbir.
Viglianisi, E., Dallago, M., and Ceccato, M. (2020).
RESTTESTGEN: Automated Black-Box Testing of
RESTful APIs. In 2020 IEEE 13th International Con-
ference on Software Testing, Validation and Verifica-
tion (ICST), pages 142–152.
Xu, D., Kent, M., Thomas, L., Mouelhi, T., and Traon,
Y. L. (2015). Automated Model-Based Testing of
Role-Based Access Control Using Predicate/Tran-
sition Nets. IEEE Transactions on Computers,
64(9):2490–2505.
ZHAW Infosec Research Group (2024). A2CT. https://
github.com/ZHAW-Infosec-Research-Group/A2CT.
ICISSP 2025 - 11th International Conference on Information Systems Security and Privacy
436