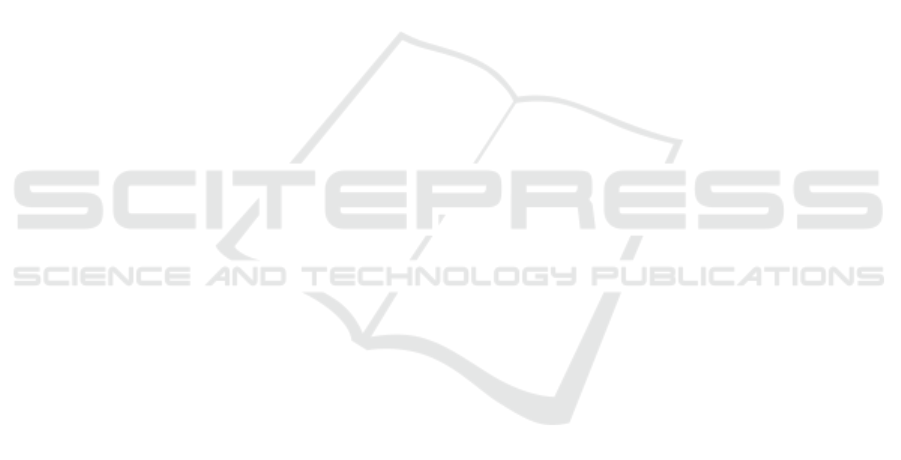
ACKNOWLEDGEMENTS
This work was supported by the Office of Naval Re-
search Command Decision Making Program under
Contract N00014-24-1-2135. The results do not re-
flect the official position of this agency.
REFERENCES
Andrews, R. W., Lilly, J. M., Srivastava, D., and Feigh,
K. M. (2023). The role of shared mental models in
human-ai teams: a theoretical review. Theoretical Is-
sues in Ergonomics Science, 24(2):129–175.
Awad, E., Dsouza, S., Kim, R., Schulz, J., Henrich, J., Shar-
iff, A., Bonnefon, J.-F., and Rahwan, I. (2018). The
moral machine experiment. Nature, 563:59–64.
Bansal, G., Nushi, B., Kamar, E., Lasecki, W. S., Weld,
D. S., and Horvitz, E. (2019). Beyond accuracy:
The role of mental models in human-ai team perfor-
mance. In AAAI Conference on Human Computation
& Crowdsourcing.
Bartneck, C., L
¨
utge, C., Wagner, A., and Welsh, S. (2021).
An Introduction to Ethics in Robotics and AI.
Cannon-Bowers, J. and Salas, E. (1990). Cognitive psychol-
ogy and team training: Training shared mental models
and complex systems. Human Factors Society Bul-
letin, pages 1–4.
Converse, S., Cannon-Bowers, J. A., and Salas, E. (1993).
Shared mental models in expert team decision mak-
ing. Individual and group decision making: Current
issues, 221:221–46.
Cummings, M. (2004). Automation bias in intelligent time
critical decision support systems. Collection of Tech-
nical Papers - AIAA 1st Intelligent Systems Technical
Conference, 2.
Das, D., Banerjee, S., and Chernova, S. (2021). Ex-
plainable ai for robot failures. In Proceedings of
the 2021 ACM/IEEE International Conference on
Human-Robot Interaction. ACM.
Dietvorst, B., Simmons, J., and Massey, C. (2014). Algo-
rithm aversion: People erroneously avoid algorithms
after seeing them err. Journal of experimental psy-
chology. General, 144.
Doshi-Velez, F. and Kim, B. (2017). Towards a rigorous
science of interpretable machine learning.
Groom, V. and Nass, C. (2007). Can robots be teammates?
benchmarks in human-robot teams. Interaction Stud-
ies, 8:483–500.
Handmer, J. and Proudley, B. (2007). Communicating un-
certainty via probabilities: The case of weather fore-
casts. Environmental Hazards, 7:79–87.
Hart, S. G. and Staveland, L. E. (1988). Development
of nasa-tlx (task load index): Results of empirical
and theoretical research. Human mental workload,
1(3):139–183.
Illingworth, D. A. and Feigh, K. M. (2021). Impact map-
ping for geospatial reasoning and decision making.
Human Factors, page 0018720821999021.
Johnson-Laird, P. N. (1983). Mental models: Towards
a cognitive science of language, inference, and con-
sciousness. Number 6. Harvard University Press.
Kamar, E. (2016). Directions in hybrid intelligence: Com-
plementing ai systems with human intelligence. In
Proceedings of the Twenty-Fifth International Joint
Conference on Artificial Intelligence, IJCAI’16, page
4070–4073. AAAI Press.
Kamar, E., Hacker, S., and Horvitz, E. (2012). Com-
bining human and machine intelligence in large-
scale crowdsourcing. In Proceedings of the 11th In-
ternational Conference on Autonomous Agents and
Multiagent Systems - Volume 1, AAMAS ’12, page
467–474, Richland, SC. International Foundation for
Autonomous Agents and Multiagent Systems.
Kingston, J. (2018). Artificial intelligence and legal liabil-
ity.
Kleinman, D. L. and Serfaty, D. (1989). Team performance
assessment in distributed decision making. In Pro-
ceedings of the symposium on interactive networked
simulation for training, pages 22–27. University of
Central Florida Orlando, FL.
Kulesza, T., Stumpf, S., Burnett, M., and Kwan, I. (2012).
Tell me more? the effects of mental model soundness
on personalizing an intelligent agent. In Proceedings
of the SIGCHI Conference on Human Factors in Com-
puting Systems, CHI ’12, page 1–10, New York, NY,
USA. Association for Computing Machinery.
Kulesza, T., Stumpf, S., Burnett, M., Yang, S., Kwan, I.,
and Wong, W.-K. (2013). Too much, too little, or
just right? ways explanations impact end users’ men-
tal models. In 2013 IEEE Symposium on Visual Lan-
guages and Human Centric Computing, pages 3–10.
Mathieu, J. E., Heffner, T. S., Goodwin, G. F., Salas, E.,
and Cannon-Bowers, J. A. (2000). The influence of
shared mental models on team process and perfor-
mance. Journal of applied psychology, 85(2):273.
Mosier, K. L. and Skitka, L. J. (1999). Automation use and
automation bias. Proceedings of the Human Factors
and Ergonomics Society Annual Meeting, 43(3):344–
348.
Mueller, S. T., Hoffman, R. R., Clancey, W. J., Emrey, A.,
and Klein, G. (2019). Explanation in human-ai sys-
tems: A literature meta-review, synopsis of key ideas
and publications, and bibliography for explainable ai.
ArXiv, abs/1902.01876.
Norman, D. A. (2013). The Design of Everyday Things.
MIT Press.
Orasanu, J. M. and Salas, E. (1993). Team decision mak-
ing in complex environments. page 327–345. Ablex
Publishing.
O’Neill, T., McNeese, N., Barron, A., and Schelble, B.
(2022). Human–autonomy teaming: A review and
analysis of the empirical literature. Human Factors,
64(5):904–938. PMID: 33092417.
Poursabzi-Sangdeh, Forough, Goldstein, G, D., Hofman,
J. M., Wortman Vaughan, J. W., and Wallach, H.
(2021). Manipulating and measuring model inter-
pretability. In Proceedings of the 2021 CHI Confer-
ence on Human Factors in Computing Systems, CHI
HUCAPP 2025 - 9th International Conference on Human Computer Interaction Theory and Applications
462