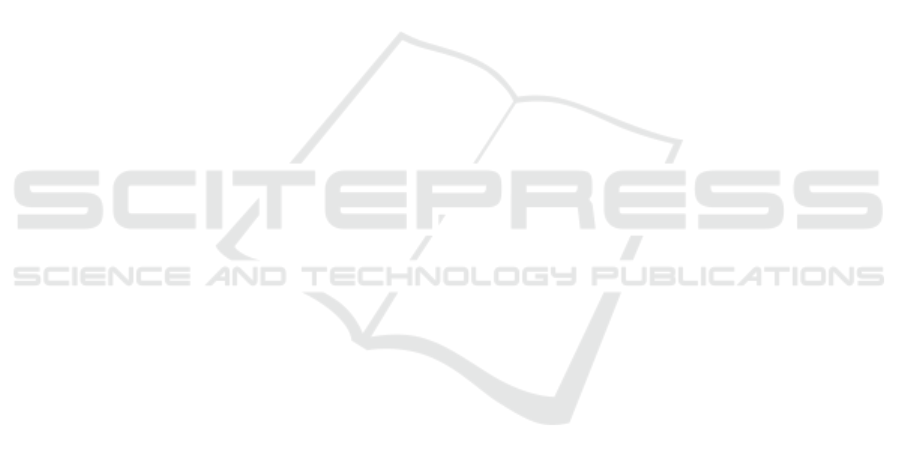
6 SUMMARY
A procedure for estimating driver’s attention levels
over a driven course was developed using a state-
space modelling technique with saccade rates and
pupillary changes. In order to consider the interac-
tion of the model’s parameters, the attention estima-
tion model was revised. The estimated attention levels
are assessed along the routes driven. As a result, the
estimated attention level decreased during routes with
turns, such as Left-turn and Right-turn, in comparison
with the straight route.
Also, driver’s ratings for cognitive workload, such
as the Frustration factor, correlate with mean atten-
tion levels over all 5 route groups when surveyed after
driving as been completed. Some statistical informa-
tion regarding changes in levels of attention correlate
with some of the ratings for cognitive workload fac-
tors.
Examination of the contribution of route factors to
attention levels will be a subject of our further study.
REFERENCES
Adler, G., Rottunda, S., and Dysken, M. (2005). The older
driver with dementia: an updated literature review.
Journal of safety research, 36(4):399–407.
Arrington Research (2016). ViewPoint EyeTracker User-
Guide.
Ball, K. and Owsley, C. (1993). The useful field of view
test: A new technique for evaluating age-related de-
clines in visual function. Journal of the American Op-
tometric Association, 64(1):71–79.
Deng, T., Yan, H., Qin, L., Ngo, T., and Manjunath, B. S.
(2020). How do drivers allocate their potential at-
tention? driving fixation prediction via convolutional
neural networks. IEEE Trans. ITS, 21(5):2146–2154.
Dubiel, M., Nakayama, M., and Wang, X. (2023). Mod-
elling attention levels with ocular responses in a
speech-in-noise recall task. In Proc. ETRA 2023,
pages 89:1–89:7. ACM.
Hart, S. G. (2006). NASA-task load index (NASA-TLX);
20 years later. In Proceedings of the human factors
and Ergonomics Society 50th Annual meeting, pages
904–908.
Hu, Z., Lv, C., Hang, P., Huang, C., and Xing, Y.
(2022). Data-driven estimation of driver attention us-
ing calibration-free eye gaze and scene features. IEEE
Trans. Industorial Electronics, 69(2):1800–1808.
Kapitaniak, B., Walczak, M., Kosobudzki, M., J
´
o
´
zwiak,
Z., and Bortkiewicz, A. (2015). Application of eye-
tracking in drivers testing: a review of research. Inter-
national Journals of Occupational Medicine and En-
vironmental Health, 28(6):941–954.
K
¨
ubler, T. C., Fuhl, W., Wagner, E., and Kasneci, E. (2021).
55 rides: Attention annotated head and gaze data dur-
ing naturalistic driving. In Spencer, S. N., editor,
Proceedings of ACM Symposium on Eye-Tracking Re-
search & Applications (ETRA 2021), pages 17(1–8),
New York, USA. ACM.
Mombaugh, T. N. and McIntyre, N. J. (1992). The
mini-mental state examination: A comprehensive re-
view. Journal of the American Geriatrics Society,
40(9):922–935.
Nakayama, M., Sun, Q. C., and Xia, J. C. (2022). Es-
timation of older driver’s cognitive performance and
workload using features of eye movement and pupil
response on test routes. In Proc. IV 2022, pages 155–
160. IEEE.
Nakayama, M., Sun, Q. C., and Xia, J. C. (2024a). Car driv-
ing temporal cognitive workload estimation using fea-
tures of eye tracking. In Proc. Eye Tracking Research
& Applications (ETRA) 2024, pages 35:1–3. ACM.
Nakayama, M., Sun, Q. C., and Xia, J. C. (2024b). Using
oculo-motor features to estimate cognitive workload
while driving a test route. The Transactions of Human
Interface Society, 26(3):273–276.
Paeglis, R., Bluss, K., and Atvars, A. (2011). Driving expe-
rience and special skills reflected in eye movements.
In Proceedings of SPIE, pages 1–7.
Palazzi, A., Abati, D., Calderara, S., Solera, F., and Cuc-
chiara, R. (2019). Predicting the driver’s focus of at-
tention: The DR(eye)VE project. IEEE Trans. PAMI,
41(7):1720–1733.
Schmitt, K.-U., Seeger, R., Fischer, H., Lanz, C., Muser,
M., Walz, F., and Schwarz, U. (2015). Saccadic eye
movement performance as an indicator of driving abil-
ity in elderly drivers. Swiss Medical Weekly, 145:1–
10.
Sun, Q. C., Xia, J. C., Falkmer, T., and Lee, H. (2016a). In-
vestigating the spatial pattern of older drivers’ eye fix-
ation behaviour and associations with their visual ca-
pacity. Journal of Eye Movement Research, 9(6):2:1–
16.
Sun, Q. C., Xia, J. C., Foster, J., Falkmer, T., and Lee, H.
(2018a). Driving manoeuvre during lane maintenance
in older adults: Associations with neuropsychological
scores. Transportation Research Part F, 53:117–129.
Sun, Q. C., Xia, J. C., Foster, J., Falkmer, T., and Lee, H.
(2018b). A psycho-Geoinformatics approach for in-
vestigating older adults’ driving behaviours and un-
derlying cognitive mechanisms. European Transport
Research Review, 10(2)(36):1–11.
Sun, Q. C., Xia, J. C., He, J., Foster, J., Falkmer, T., and Lee,
H. (2018c). Towards unpacking older drivers’ visual-
motor coodination: A gaze-based integrated driving
assessment. Accident Analysis & Prevention, 113:85–
96.
Sun, Q. C., Xia, J. C., Nadarajah, N., Falkmer, T., and Lee,
H. (2016b). Assessing drivers’ visual-motor coordi-
nation using eye tracking, GNSS and GIS: a spatial
turn in driving psychology. Journal of Spatial Science,
61(2):299–316.
Sun, Q. C., Xia, J. C., Nadarajah, N., Foster, J., and
Lee, H. (2015). Positioning eye fixation and vehi-
cle movement: Visual-motor coordination assessment
Changes in Attention Levels While Driving a Car Estimated Using Modelling Techniques with Features of Oculo-Motors
851