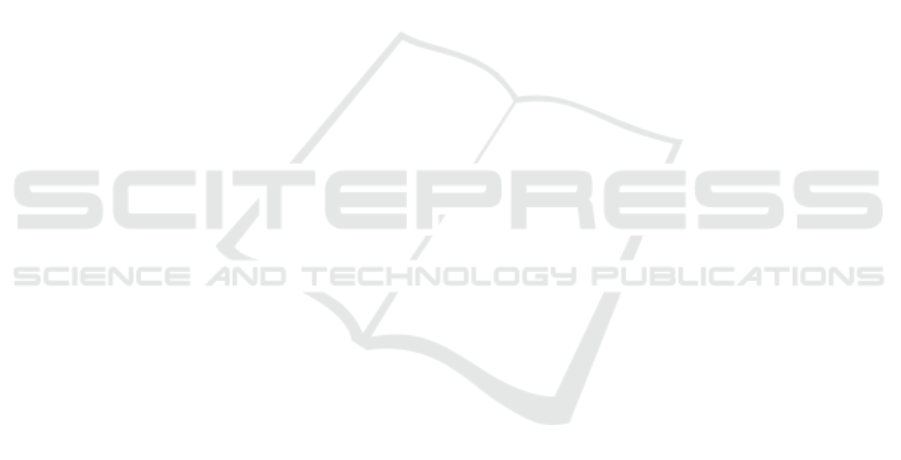
REFERENCES
Angel, E. and Morrison, D. (1991). Speeding up bresen-
ham’s algorithm. IEEE Computer Graphics and Ap-
plications, 11(6):16–17.
Bader, M., Mehl, M., R
¨
ude, U., and Wellein, G. (2011).
Simulation software for supercomputers. Journal of
Computational Science, 2(2):93–94.
Bryson, S. and Levit, C. (1992). The virtual wind tun-
nel. IEEE Computer Graphics and Applications,
12(4):25–34.
Burch, M. (2022). Eye Tracking and Visual Analytics. River
Publishers.
Burch, M., Wallner, G., Arends, S. T. T., and Beri, P.
(2020). Procedural city modeling for AR applications.
In et al., E. B., editor, Proceedings of the 24th Inter-
national Conference on Information Visualisation, IV,
pages 581–586. IEEE.
Chorin, A. J. (1967). Numerical solution of the navier-
stokes equations. Mathematics of Computation,
22:745–762.
Deininger, M. E., von der Gr
¨
un, M., Piepereit, R., Schnei-
der, S., Santhanavanich, T., Coors, V., and Voß, U.
(2020). A continuous, semi-automated workflow:
From 3d city models with geometric optimization and
CFD simulations to visualization of wind in an urban
environment. ISPRS International Journal of Geo-
Information, 9(11):657.
Eidgenossenschaft, S. (2024). Bundesamt f
¨
ur Landesto-
pografie swisstopo.
Forney, G. P., Madrzykowski, D., McGrattan, K. B., and
Sheppard, L. (2003). Understanding fire and smoke
flow through modeling and visualization. IEEE Com-
puter Graphics and Applications, 23(4):6–13.
Kirkil, G. and Lin, C. (2020). Large eddy simulation of
wind flow over A realistic urban area. Computation,
8(2):47.
Li, Y. and Zhao, H. (2023). Spatial wind flow load and
shape optimization for folding grid shell buildings.
IEEE Access, 11:135304–135322.
Mayo, M., Wakes, S., and Anderson, C. (2018). Neural net-
works for predicting the output of wind flow simula-
tions over complex topographies. In Wu, X., Ong, Y.,
Aggarwal, C. C., and Chen, H., editors, Proceedings
of the IEEE International Conference on Big Knowl-
edge, ICBK, pages 184–191. IEEE Computer Society.
Meinel, G. (2008). High resolution analysis of settlement
structure on base of topographic raster maps - method
and implementation. In Gervasi, O., Murgante, B., La-
gan
`
a, A., Taniar, D., Mun, Y., and Gavrilova, M. L.,
editors, Computational Science and Its Applications
- ICCSA 2008, International Conference, Perugia,
Italy, June 30 - July 3, 2008, Proceedings, Part I,
volume 5072 of Lecture Notes in Computer Science,
pages 16–25. Springer.
Papalexiou, S. and Moussiopoulos, N. (2006). Wind
flow and photochemical air pollution in thessaloniki,
greece. part II: statistical evaluation of european
zooming model’s simulation results. Environmental
Modelling and Software, 21(12):1752–1758.
Paterson, D. and Apelt, C. (1989). Simulation of wind flow
around three-dimensional buildings. Building and En-
vironment, 24(1):39–50.
Ridzuan, N., Ujang, U., and Azri, S. (2023). 3d vector-
ization and rasterization of citygml standard in wind
simulation. Earth Science Informatics, 16(3):2635–
2647.
Schlemmer, M., Hotz, I., Hamann, B., Morr, F., and Hagen,
H. (2007). Priority streamlines: A context-based visu-
alization of flow fields. In Museth, K., M
¨
oller, T., and
Ynnerman, A., editors, Proceedings of the 9th Joint
Eurographics - IEEE VGTC Symposium on Visualiza-
tion, EuroVis, pages 227–234. Eurographics Associa-
tion.
Seebacher, D., Miller, M., Polk, T., Fuchs, J., and Keim,
D. A. (2019). Visual analytics of volunteered geo-
graphic information: Detection and investigation of
urban heat islands. IEEE Computer Graphics and Ap-
plications, 39(5):83–95.
van Wijk, J. J. (1993). Flow visualization with surface par-
ticles. IEEE Computer Graphics and Applications,
13(4):18–24.
Wang, T., Wang, B., Matekole, E. S., and Atif, M. (2022).
Finding hidden patterns in high resolution wind flow
model simulations. In Kothe, D. B., Geist, A.,
Pophale, S., Liu, H., and Parete-Koon, S., editors,
Proceedings of the Accelerating Science and Engi-
neering Discoveries Through Integrated Research In-
frastructure for Experiment, Big Data, Modeling and
Simulation - 22nd Smoky Mountains Computational
Sciences and Engineering Conference, SMC, Revised
Selected Papers, volume 1690 of Communications in
Computer and Information Science, pages 345–365.
Springer.
Wu, A., Deng, D., Chen, M., Liu, S., Keim, D. A., Ma-
ciejewski, R., Miksch, S., Strobelt, H., Vi
´
egas, F. B.,
and Wattenberg, M. (2023). Grand challenges in vi-
sual analytics applications. IEEE Computer Graphics
and Applications, 43(5):83–90.
Yan, Z., Chen, R., and Cai, X. (2022). Large eddy simu-
lation of the wind flow in a realistic full-scale urban
community with a scalable parallel algorithm. Com-
puter Physics Communications, 270:108170.
Yang, Z., Sarkar, P., and Hu, H. (2010). Visualiza-
tion of flow structures around a gable-roofed building
model in tornado-like winds. Journal of Visualization,
13(4):285–288.
Yi, J. S., ah Kang, Y., Stasko, J. T., and Jacko, J. A.
(2007). Toward a deeper understanding of the role
of interaction in information visualization. IEEE
Transactions on Visualization and Computer Graph-
ics, 13(6):1224–1231.
Yin, J., Zhan, Q., Xiao, Y., Wang, T., Che, E., Meng, F.,
and Qian, Y. (2014). Correlation between urban mor-
phology and wind environment in digital city using
GIS and CFD simulations. International Journal of
Online Engineering, 10(3):42–48.
Zu, G. and Lam, K. M. (2018). LES and wind tunnel test
of flow around two tall buildings in staggered arrange-
ment. Computation, 6(2):28.
IVAPP 2025 - 16th International Conference on Information Visualization Theory and Applications
830