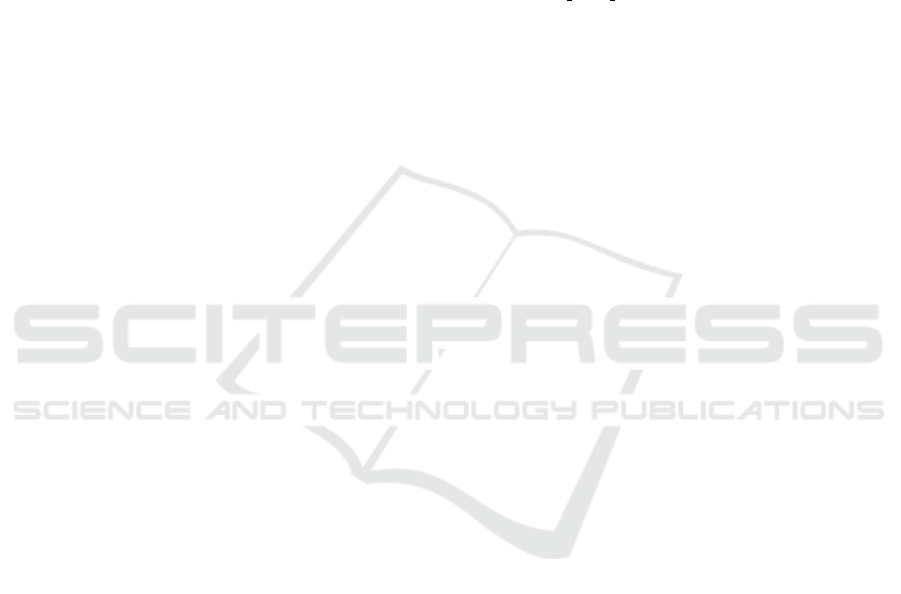
Dhakar, L. (n.d.). Color-thief. Available at
https://lokeshdhakar.com/projects/color-thief/,
accessed April 24.
Dougherty, D. (2002). Vischeck. Available at https://www.
vischeck.com/, accessed April 24.
Eddins, S. (2006). A lab-based uniform color scale.
https://blogs.mathworks.com/steve/2006/05/09/
a-lab-based-uniform-color-scale/.
Fang, H., Walton, S., Delahaye, E., Harris, J., Storchak,
D. A., and Chen, M. (2017). Categorical colormap
optimization with visualization case studies. IEEE
Transactions on Visualization and Computer Graph-
ics, 23(1):871–880.
Geissbuehler, M. and Lasser, T. (2013). How to display data
by color schemes compatible with red-green color per-
ception deficiencies. Opt. Express, 21(8):9862–9874.
Gramazio, C. C., Laidlaw, D. H., and Schloss, K. B. (2017).
Colorgorical: Creating discriminable and preferable
color palettes for information visualization. IEEE
Transactions on Visualization and Computer Graph-
ics, 23(1):521–530.
Green, D. A. (2011). A colour scheme for the display of as-
tronomical intensity images. https://doi.org/10.48550/
arXiv.1108.5083 [Preprint].
Green-Armytage, P. (2006). The value of knowledge
for colour design. Color Research & Application,
31(4):253–269.
Guidelines, A. S. (n.d.). https://spectrum.adobe.com/page/
color-for-data-visualization/.
Harrower, M. and Brewer, C. A. (2003). Colorbrewer.org:
An online tool for selecting colour schemes for maps.
The Cartographic Journal, 40(1):27–37.
Heer, J. and Stone, M. (2012). Color naming models
for color selection, image editing and palette design.
In Proceedings of the SIGCHI Conference on Hu-
man Factors in Computing Systems, CHI ’12, page
1007–1016, New York, NY, USA. Association for
Computing Machinery.
Kim, Y., Thayer, K., Gorsky, G. S., and Heer, J. (2019).
Color names across languages: Salient colors and
term translation in multilingual color naming models.
In EuroVis (Short Papers), pages 31–35.
Kita, N. and Miyata, K. (2016). Aesthetic rating and color
suggestion for color palettes. Computer Graphics Fo-
rum, 35:127–136.
Lee, W.-Y., Gong, S.-M., and Leung, C.-Y. (2009). Is color
preference affected by age difference. International
Association of Societies of Design Research, pages
1837–1846.
Li, C. J., Luo, M. R., and Hunt, R. W. G. (2000). A revision
of the ciecam97s model. Color Research & Applica-
tion, 25(4):260–266.
Lim, W. C., Wong, C. O., and Wong, L. K. (2021). Color
aesthetic enhancement for categorical data visualiza-
tion. In Advances in Visual Informatics: 7th Interna-
tional Visual Informatics Conference, IVIC 2021, Ka-
jang, Malaysia, November 23–25, 2021, Proceedings,
page 15–26, Berlin, Heidelberg. Springer-Verlag.
Lu, S. and Meeks, E. (2022). Viz palette. Available at https:
//projects.susielu.com/viz-palette, accessed April 24.
Mittelst
¨
adt, S., J
¨
ackle, D., Stoffel, F., and Keim, D. A.
(2015). ColorCAT: Guided Design of Colormaps for
Combined Analysis Tasks. In Bertini, E., Kennedy,
J., and Puppo, E., editors, Eurographics Conference
on Visualization (EuroVis) - Short Papers. The Euro-
graphics Association.
Mittelst
¨
adt, S., Stoffel, A., and Keim, D. A. (2014). Meth-
ods for Compensating Contrast Effects in Information
Visualization. Computer Graphics Forum.
Niccoli, M. (2013). Perceptual rainbow palette –
the method. https://mycartablog.com/2013/02/21/
perceptual-rainbow-palette-the-method/.
pair color code, . (n.d.). https://en.wikipedia.org/wiki/
25-pair color code.
Pettiross, J. (2014). Crafting data colors and stay-
ing on brand. https://observablehq.com/blog/
crafting-data-colors.
Shimizu, C. and Meyer, G. W. (2010). Color styling tools.
In International Conference on Communications in
Computing.
Stone, M. (2012). In color perception, size matters. IEEE
Computer Graphics and Applications, 32(2):8–13.
Stone, M. (2016). How we designed the new color palettes
in tableau 10. Available at https://www.tableau.com/
blog/colors-upgrade-tableau-10-56782, accessed
April 24.
Szafir, D. A. (2018). Modeling color difference for visu-
alization design. IEEE Transactions on Visualization
and Computer Graphics, 24(1):392–401.
Waskom, M. L. (2021). seaborn: statistical data visualiza-
tion. Journal of Open Source Software, 6(60):3021.
Zeileis, A., Hornik, K., and Murrell, P. (2009). Escaping rg-
bland: Selecting colors for statistical graphics. Com-
putational Statistics & Data Analysis, 53(9):3259–
3270.
IVAPP 2025 - 16th International Conference on Information Visualization Theory and Applications
850