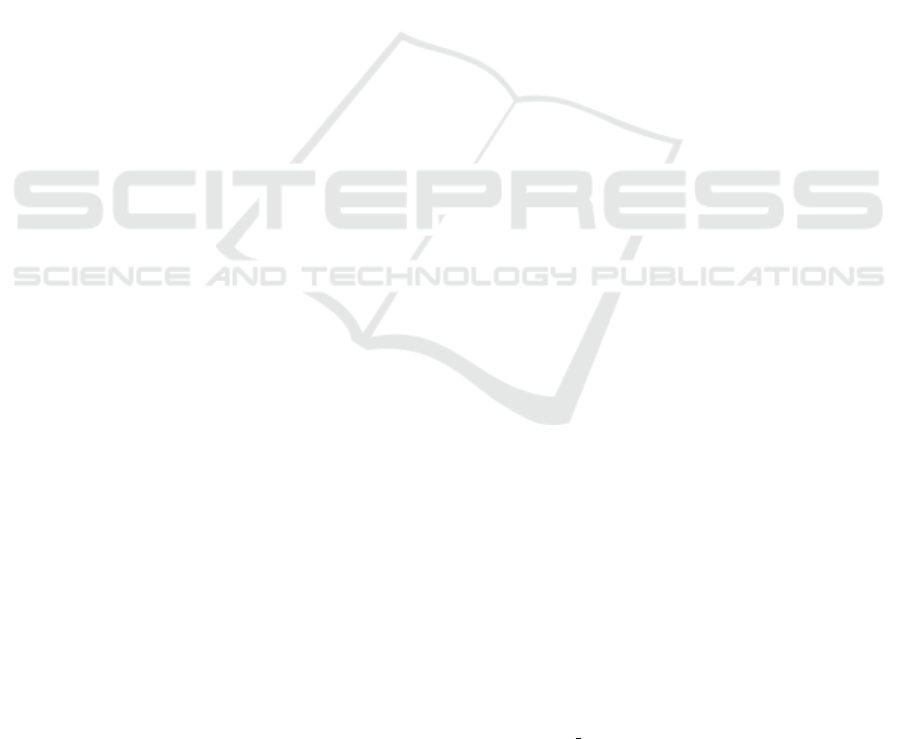
captured simultaneously, and takes these data in a
temporal sequence as input.
The study showcases the adaptability of NNs
for predicting punctual objects in images, leverag-
ing NMS techniques, residual blocks using morpho-
logical features of images, and their integration with
dropout layers. StrikeNet yielded compelling results,
achieving an IoU of 48 %, a F
1
of 53 % and a F
2
of
55 %, outperforming other deep neural networks. The
StrikeNet encoder also showed some improvements
on other datasets such as AED compared to other
state-of-the-art networks. Compared to traditional se-
mantic segmentation models, these findings represent
a significant advancement for this type of task.
Future objectives include extending the forecast
range to an hour, with predictions every 5 minutes.
Additionally, we plan to use different data sources
such as GLM groups data or NWP outputs, and see
if it is improving the predictions.
ACKNOWLEDGEMENTS
This research is co-funded by the ALBATROS
project, from the European Union Horizon Europe
under Grant Agreement N°101077071. We thank the
NOAA National Geophysical Data Center for provid-
ing the GOES-R data.
REFERENCES
Bodnar, C., Bruinsma, W. P., Lucic, A., Stanley, M., Brand-
stetter, J., Garvan, P., Riechert, M., Weyn, J., Dong,
H., Vaughan, A., Gupta, J. K., Tambiratnam, K.,
Archibald, A., Heider, E., Welling, M., Turner, R. E.,
and Perdikaris, P. (2024). Aurora: A foundation
model of the atmosphere. arXiv 2405.1306.
Bouchard, A., Buguet, M., Chan-Hon-Tong, A., Dezert, J.,
and Lalande, P. (2022). Comparison of different fore-
casting tools for short-range lightning strike risk as-
sessment. Natural Hazards.
Bouget, V., B
´
er
´
eziat, D., Brajard, J., Charantonis, A., and
Filoche, A. (2021). Fusion of rain radar images and
wind forecasts in a deep learning model applied to rain
nowcasting. Remote Sensing.
Che, H., Niu, D., Zang, Z., Cao, Y., and Chen, X. (2022).
ED-DRAP: Encoder–Decoder deep residual attention
prediction network for radar echoes. IEEE Geoscience
and Remote Sensing Letters.
Chen, L.-C., Papandreou, G., Kokkinos, I., Murphy, K., and
Yuille, A. L. (2018). Deeplab: Semantic image seg-
mentation with deep convolutional nets, atrous convo-
lution, and fully connected crfs. IEEE Transactions
on Pattern Analysis and Machine Intelligence.
Defazio, A., Yang, X., Mehta, H., Mishchenko, K., Khaled,
A., and Cutkosky, A. (2024). The road less scheduled.
Esmaeilzehi, A., Ahmad, M. O., and Swamy, M. N. S.
(2022). SRNMSM: A deep light-weight image super
resolution network using multi-scale spatial and mor-
phological feature generating residual blocks. IEEE
Transactions on Broadcasting.
Ferreira, G. A. V. S., Leal, A. F. R., Lopes, M. N. G., and
da Rocha, L. C. (2024). Lightning nowcast on airports
in the amazon region using machine learning.
Goodman, S. J., Blakeslee, R. J., Koshak, W. J., Mach,
D., Bailey, J., Buechler, D., Carey, L., Schultz, C.,
Bateman, M., McCaul, E., and Stano, G. (2013). The
goes-r geostationary lightning mapper (GLM). Atmo-
spheric Research.
Lam, R., Sanchez-Gonzalez, A., Willson, M., Wirnsberger,
P., Fortunato, M., Alet, F., Ravuri, S., Ewalds, T.,
Eaton-Rosen, Z., Hu, W., Merose, A., Hoyer, S., Hol-
land, G., Vinyals, O., Stott, J., Pritzel, A., Mohamed,
S., and Battaglia, P. (2023). Learning skillful medium-
range global weather forecasting. Science.
Leinonen, J., Hamann, U., and Germann, U. (2022).
Seamless lightning nowcasting with recurrent-
convolutional deep learning. Artificial Intelligence
for the Earth Systems.
Leinonen, J., Hamann, U., Sideris, I. V., and Germann, U.
(2023). Thunderstorm nowcasting with deep learn-
ing: A multi-hazard data fusion model. Geophysical
Research Letters.
Naude, J. and Joubert, D. (2019). The aerial elephant
dataset: A new public benchmark for aerial object de-
tection. In Conference on Computer Vision and Pat-
tern Recognition.
Neubeck, A. and Van Gool, L. (2006). Efficient non-
maximum suppression. In 18th International Confer-
ence on Pattern Recognition (ICPR’06).
P
´
edeboy, S., Barneoud, P., and Berthet, C. (2016). First
results on severe storms prediction based on the french
national lightning locating system. In International
Lightning Detection Conference.
Ronneberger, O., Fischer, P., and Brox, T. (2015). U-
Net: Convolutional networks for biomedical image
segmentation. In Medical Image Computing and
Computer-Assisted Intervention.
Schmit, T. J., Griffith, P., Gunshor, M. M., Daniels, J. M.,
Goodman, S. J., and Lebair, W. J. (2017). A closer
look at the ABI on the GOES-R Series. Bulletin of the
American Meteorological Society.
Tan, M. and Le, Q. (2021). EfficientNetV2: Smaller mod-
els and faster training. In International conference on
machine learning. PMLR.
Ukkonen, P., Manzato, A., and M
¨
akel
¨
a, A. (2017). Evalu-
ation of thunderstorm predictors for finland using re-
analyses and neural networks. Journal of Applied Me-
teorology and Climatology.
U.S. Department of Transportation, F. A. A. F.
(2/19/13). Advisory circular (ac) no 00-24c.
https://www.faa.gov/documentlibrary/media/
advisory circular/ac%2000-24c.pdf.
Zhou, K., Zheng, Y., Dong, W., and Wang, T. (2020). A
deep learning network for cloud-to-ground lightning
nowcasting with multisource data. Journal of Atmo-
spheric and Oceanic Technology.
VISAPP 2025 - 20th International Conference on Computer Vision Theory and Applications
306