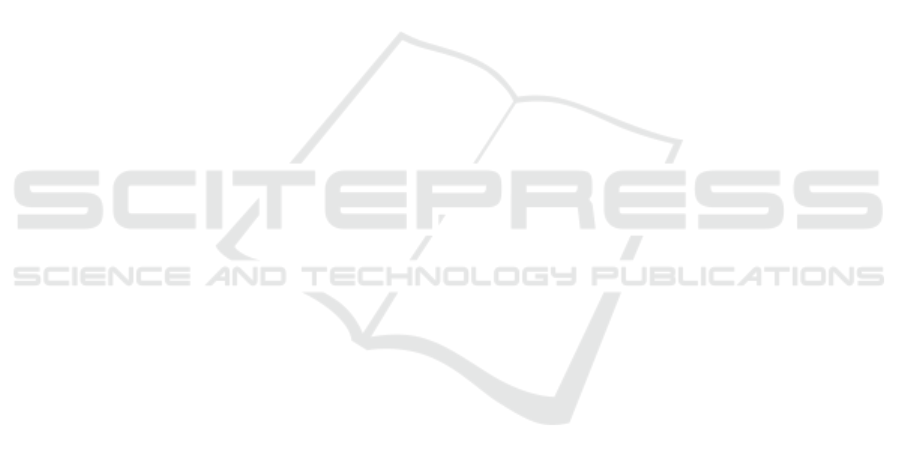
works. Also, we could develop concrete instances of
systems that use AI for graph drawing and provide
explanations. Evaluation of these can teach us what
the contribution of XGD could be in practice. Fur-
thermore, expanding the valuation on different tech-
niques could lead to the creation of design guidelines
for XAI method selection, ultimately contributing to
the broader goal of enhancing AI interpretability and
trustworthiness of AI for GD.
REFERENCES
Ahmed, R., De Luca, F., Devkota, S., Kobourov, S., and
Li, M. (2022). Multicriteria scalable graph drawing
via stochastic gradient descent, (sgd)
2
(sgd)2. IEEE
Transactions on Visualization and Computer Graph-
ics, 28(6):2388–2399.
Alicioglu, G. and Sun, B. (2022). A survey of visual an-
alytics for explainable artificial intelligence methods.
Computers & Graphics, 102:502–520.
Barredo Arrieta, A., D
´
ıaz-Rodr
´
ıguez, N., Del Ser, J., Ben-
netot, A., Tabik, S., Barbado, A., Garcia, S., Gil-
Lopez, S., Molina, D., Benjamins, R., Chatila, R.,
and Herrera, F. (2020). Explainable artificial intelli-
gence (xai): Concepts, taxonomies, opportunities and
challenges toward responsible ai. Information Fusion,
58:82–115.
Cao, J., Li, M., Chen, X., Wen, M., Tian, Y., Wu, B., and
Cheung, S.-C. (2022). Deepfd: automated fault diag-
nosis and localization for deep learning programs. In
Proceedings of the 44th International Conference on
Software Engineering, page 573–585. Association for
Computing Machinery.
Eades, P., Hong, S.-H., Nguyen, A., and Klein, K. (2017).
Shape-based quality metrics for large graph visualiza-
tion. Journal of Graph Algorithms and Applications,
21(1):29–53.
El-Assady, M., Jentner, W., Kehlbeck, R., Schlegel, U.,
Sevastjanova, R., Sperrle, F., Spinner, T., and Keim,
D. (2019). Towards explainable artificial intelligence:
Structuring the processes of explanations. In HCML
Workshop at CHI’19, Glasgow, UK.
Elzen, S. v. d., Andrienko, G., Andrienko, N., Fisher, B. D.,
Martins, R. M., Peltonen, J., Telea, A. C., and Ver-
leysen, M. (2023). The flow of trust: A visualization
framework to externalize, explore, and explain trust in
ml applications. IEEE Computer Graphics and Appli-
cations, 43(2):78–88.
Giovannangeli, L., Lalanne, F., Auber, D., Giot, R., and
Bourqui, R. (2021). Deep neural network for drawing
networks, (dnn)
2
. In Purchase, H. C. and Rutter, I.,
editors, Graph Drawing and Network Visualization,
pages 375–390, Cham.
Giovannangeli, L., Lalanne, F., Auber, D., Giot, R., and
Bourqui, R. (2024). Toward efficient deep learning for
graph drawing (dl4gd). IEEE Transactions on Visual-
ization and Computer Graphics, 30(2):1516–1532.
Gobbo, B., Elli, T., Hinrichs, U., and El-Assady, M. (2022).
xai-primer.com — a visual ideation space of interac-
tive explainers. In Extended Abstracts of the 2022 CHI
Conference on Human Factors in Computing Systems,
CHI EA ’22, New York, NY, USA. Association for
Computing Machinery.
Holter, S. and El-Assady, M. (2024). Deconstruct-
ing human-ai collaboration: Agency, interaction,
and adaptation. Computer Graphics Forum, 43(3).
Manuscript received; accepted. The final version
available at Eurographics.
Kwon, O.-H., Crnovrsanin, T., and Ma, K.-L. (2019). A
deep generative model for graph layout. In IEEE Pa-
cific Visualization Symposium (PacificVis), pages 96–
100, Bangkok, Thailand. IEEE.
Lundberg, S. M. and Lee, S. (2017). A unified approach
to interpreting model predictions. In Proceedings of
the 31st International Conference on Neural Informa-
tion Processing Systems (NIPS’17), pages 4768–4777,
Red Hook, NY, USA. Curran Associates Inc.
Miksch, S. and Aigner, W. (2014). A matter of time: Ap-
plying a data–users–tasks design triangle to visual an-
alytics of time-oriented data. Computers & Graphics,
38:286–290.
Ribeiro, M. T., Singh, S., and Guestrin, C. (2016). “why
should i trust you?” explaining the predictions of any
classifier. In Proceedings of the 22nd ACM SIGKDD
International Conference on Knowledge Discovery
and Data Mining, pages 1135–1144, San Francisco,
CA, USA. ACM.
Ribeiro, M. T., Singh, S., and Guestrin, C. (2018). Anchors:
High-precision model-agnostic explanations. In Pro-
ceedings of the Thirty-Second AAAI Conference on
Artificial Intelligence (AAAI-18). AAAI.
Simonyan, K., Vedaldi, A., and Zisserman, A. (2014).
Deep inside convolutional networks: Visualising im-
age classification models and saliency maps. arXiv
preprint arXiv:1312.6034.
Spinner, T., Schlegel, U., Sch
¨
afer, H., and El-Assady, M.
(2020). explainer: A visual analytics framework for
interactive and explainable machine learning. IEEE
Transactions on Visualization and Computer Graph-
ics, 26(1):1064–1074.
Tiezzi, M., Ciravegna, G., and Gori, M. (2024). Graph neu-
ral networks for graph drawing. IEEE Transactions on
Neural Networks and Learning Systems, 35(4):4668–
4681.
Wang, X., Yen, K., Hu, Y., and Shen, H.-W. (2023).
Smartgd: A gan-based graph drawing framework for
diverse aesthetic goals. IEEE Transactions on Visual-
ization and Computer Graphics, pages 1–12.
Wang, Y., Jin, Z., Wang, Q., Cui, W., Ma, T., and Qu, H.
(2020). Deepdrawing: A deep learning approach to
graph drawing. IEEE Transactions on Visualization
and Computer Graphics, 26(1):676–686.
Yan, K., Zhao, T., and Yang, M. (2022). Graphuly: Graph u-
nets-based multi-level graph layout. IEICE Transac-
tions on Information and Systems, E105.D(12):2135–
2138.
IVAPP 2025 - 16th International Conference on Information Visualization Theory and Applications
858