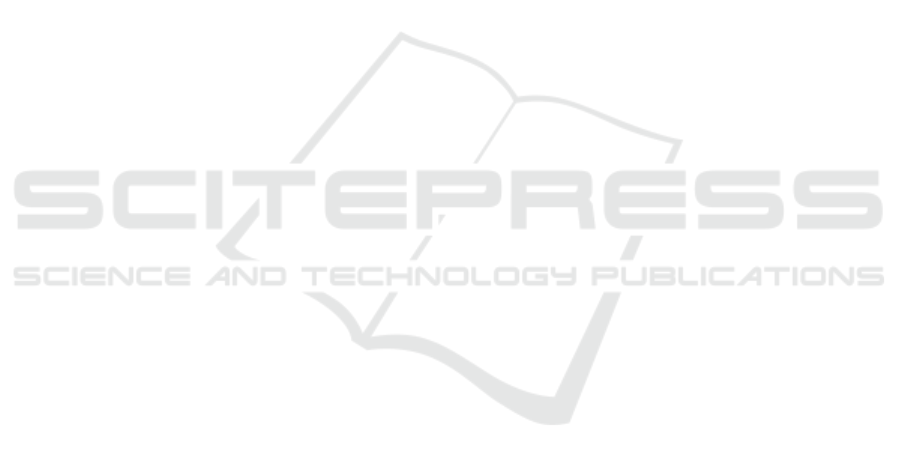
6 CONCLUSIONS
The effectiveness of visual embedding vectors for
predicting both aggregate particle size distribution
and concrete properties was investigated. Our find-
ings demonstrate that embedding vectors, when used
to train simple MLP (Multi-Layer Perceptron) mod-
els, can accurately classify aggregates. The achieved
accuracy is comparable to that obtained with more
specialized or complex models. Therefore, when
dealing with new aggregate sources, only their im-
ages need to be taken, the corresponding embedding
vectors generated, and the model retrained with these
new vectors.
In addition to aggregate classification, the poten-
tial of embedding vectors to help predict one of the
key properties of concrete was explored. To evalu-
ate this, we contructed a custom dataset and a novel
estimation approach was developed. Due to the high
cost and time investment associated with each con-
crete formulation, a limited dataset was built, focus-
ing solely on the variability of aggregate proportions
while keeping water and cement quantities constant.
To ensure the validity of the findings, the limited
dataset was intentionally split in half, training the
models on one portion and reserving the other for
evaluation on unseen data. This choice led to a very
small training set size, so data augmentation tech-
niques were proposed and implemented to expand the
training dataset with realistic synthetic new samples.
Through extensive experimentation, we validated
the effectiveness of the proposed approach. The re-
sults showed that embedding vectors are useful in
predicting concrete compressive strength. The pro-
posed augmentation techniques were also validated.
The best results were obtained when embedding vec-
tors were added to the input raw data and the train-
ing dataset was augmented using the weight and com-
pressive strength augmentation techniques. The best
model can predict concrete compressive strength with
an MAE error of 1.94 MPa and an RMSE of 2.39
MPa. Considering that this is less than half of the
5 MPa standard deviation assigned to compressive
strength when statistical data is missing (Matthews
et al., 2023), it can be stated that the approach shows
promising results despite the limited dataset. Further
exploration of hyperparameter tuning for both particle
size and compressive strength prediction processes is
now feasible to obtain the best parameters for each
model.
A limitation of our study is the size and scope of
the dataset, which included a limited number of col-
lected data points and variations in mix recipes. Ex-
panding the dataset to include other key ingredients
such as water content and cement content and its type,
would be an interesting future direction.
As future work, and to further confirm our find-
ings, we propose to expand our study to the other
properties of concrete e.g. workability, early strength,
carbonation resistance, etc. It would also be benefi-
cial to evaluate the proposed data augmentation tech-
niques on conventional datasets from the literature to
validate their contribution. Finally, we also intend to
explore the use of the visual embedding vectors to
classify mixed aggregates and again use them in the
process of estimating the properties of concrete, that
has been manufactured using these mixed aggregates.
ACKNOWLEDGEMENTS
This research was supported by a grant of the HES-
SO / Appel
`
a projets
`
a th
`
eme (AGP 123165, fils de
AGP 123164).
REFERENCES
Abdelgader, S., Kurpinska, M., Khatib, J., and Abdelgader,
H. (2022). Concrete mix design using abrams and
bolomey methods. BAU Journal - Science and Tech-
nology, 4(1).
Coenen, M. (2022). Dataset: Visual granulometry: Image-
based granulometry of concrete aggregate.
Coenen, M. (2023). Dataset: Deep granulometry.
Coenen, M., Beyer, D., Heipke, C., and Haist, M. (2022).
Learning to sieve: Prediction of grading curves from
images of concrete aggregate. ISPRS Annals of the
Photogrammetry, Remote Sensing and Spatial Infor-
mation Sciences, V-2-2022:227–235.
Hosseinzadeh, M., Dehestani, M., and Hosseinzadeh, A.
(2023). Prediction of mechanical properties of re-
cycled aggregate fly ash concrete employing machine
learning algorithms. Journal of Building Engineering,
76:107006.
Matthews, S., Bigaj-van Vliet, A., and Dieteren, G. (2023).
Outlook upon fib model code for concrete structures
(2020). Structural Concrete, 24(4):4334–4335.
Nithurshan, M. and Elakneswaran, Y. (2023). A system-
atic review and assessment of concrete strength pre-
diction models. Case Studies in Construction Materi-
als, 18:e01830.
Oquab, M., Darcet, T., Moutakanni, T., Vo, H., Szafraniec,
M., Khalidov, V., Fernandez, P., Haziza, D., Massa,
F., El-Nouby, A., Assran, M., Ballas, N., Galuba, W.,
R.Howes, Huang, P.-Y., Li, S.-W., Misra, I., Rabbat,
M., Sharma, V., Synnaeve, G., Xu, H., Jegou, H.,
Mairal, J., Labatut, P., Joulin, A., and Bojanowski, P.
(2024). Dinov2: Learning robust visual features with-
out supervision.
ICAART 2025 - 17th International Conference on Agents and Artificial Intelligence
122