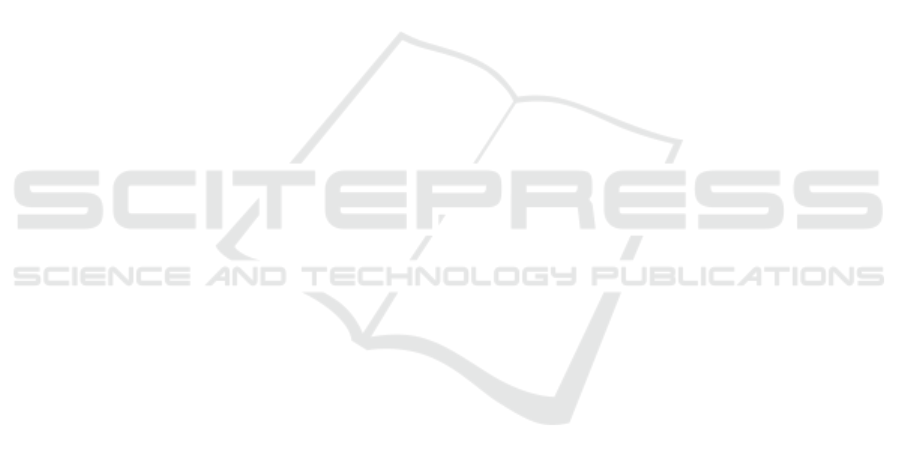
6 CONCLUSION
This paper introduces a novel two-stage approach
to 4D Panoptic Lidar Segmentation by decoupling
single-scan semantic and multi-scan instance segmen-
tation tasks. Our D-PLS framework leverages se-
mantic predictions as a coarse form of clustering and
prior information to aid in instance segmentation, sig-
nificantly outperforming the baseline, while offering
a modular and flexible solution. D-PLS capitalizes
on pretrained networks from the well-explored do-
main of single-scan semantic segmentation to sup-
ply these prior predictions, making it adaptable to a
wide range of architectures. Future work will ex-
plore extending our approach to diverse semantic and
panoptic networks. We validated our results using the
SemanticKITTI dataset, and aim to further advance
this research by incorporating label-efficient LiDAR
segmentation techniques (Reichardt et al., 2023), in-
stance specific augmentation (Reichardt et al., 2024)
and motion information (Rishav et al., 2020).
ACKNOWLEDGEMENTS
This work was funded by the German Federal
Ministry for Economic Affairs and Climate Action
(BMWK) under the grant SERiS (KK5335502LB3).
REFERENCES
Ayg
¨
un, M., Osep, A., Weber, M., Maximov, M., Stachniss,
C., Behley, J., and Leal-Taixe, L. (2021). 4D Panoptic
Segmentation. In Conference on Computer Vision and
Pattern Recognition (CVPR).
Behley, J., Garbade, M., Milioto, A., Quenzel, J., Behnke,
S., Stachniss, C., and Gall, J. (2019). SemanticKITTI:
A Dataset for Semantic Scene Understanding of Li-
DAR Sequences. In International Conference on
Computer Vision (ICCV).
Ester, M., Kriegel, H.-P., Sander, J., Xu, X., et al. (1996).
A density-based algorithm for discovering clusters in
large spatial databases with noise. In KDD.
Hong, F., Zhou, H., Zhu, X., Li, H., and Liu, Z. (2021).
Lidar-based panoptic segmentation via dynamic shift-
ing network. In Conference on Computer Vision and
Pattern Recognition (CVPR).
Hong, F., Zhou, H., Zhu, X., Li, H., and Liu, Z. (2022).
Lidas-based 4d panoptic segmentation via dynamic
shifting network. arXiv preprint arXiv:2203.07186.
Kreuzberg, L., Zulfikar, I. E., Mahadevan, S., Engelmann,
F., and Leibe, B. (2022). 4d-stop: Panoptic segmenta-
tion of 4d lidar using spatio-temporal object proposal
generation and aggregation. In European Conference
on Computer Vision (ECCV).
Marcuzzi, R., Nunes, L., Wiesmann, L., Marks, E., Behley,
J., and Stachniss, C. (2023). Mask4d: End-to-end
mask-based 4d panoptic segmentation for lidar se-
quences. Robotics and Automation Letters (RA-L).
Marcuzzi, R., Nunes, L., Wiesmann, L., Vizzo, I., Behley,
J., and Stachniss, C. (2022). Contrastive instance
association for 4d panoptic segmentation using se-
quences of 3d lidar scans. Robotics and Automation
Letters (RA-L).
Qi, C. R., Su, H., Mo, K., and Guibas, L. J. (2017). Pointnet:
Deep learning on point sets for 3d classification and
segmentation. In Conference on Computer Vision and
Pattern Recognition.
Reichardt, L., Ebert, N., and Wasenm
¨
uller, O. (2023). 360
◦
from a single camera: A few-shot approach for lidar
segmentation. In International Conference on Com-
puter Vision Workshop (ICCVW).
Reichardt, L., Uhr, L., and Wasenm
¨
uller, O. (2024).
Text3daug – prompted instance augmentation for lidar
perception. In International Conference on Intelligent
Robots and Systems (IROS).
Rishav, R., Battrawy, R., Schuster, R., Wasenm
¨
uller, O., and
Stricker, D. (2020). Deeplidarflow: A deep learning
architecture for scene flow estimation using monocu-
lar camera and sparse lidar. In IEEE/RSJ International
Conference on Intelligent Robots and Systems (IROS).
Tang, H., Liu, Z., Zhao, S., Lin, Y., Lin, J., Wang, H.,
and Han, S. (2020). Searching efficient 3d architec-
tures with sparse point-voxel convolution. In Euro-
pean Conference on Computer Vision (ECCV).
Thomas, H., Qi, C. R., Deschaud, J.-E., Marcotegui, B.,
Goulette, F., and Guibas, L. J. (2019). Kpconv: Flex-
ible and deformable convolution for point clouds. In
International Conference on Computer Vision (ICCV).
Wu, X., Jiang, L., Wang, P.-S., Liu, Z., Liu, X., Qiao, Y.,
Ouyang, W., He, T., and Zhao, H. (2024). Point trans-
former v3: Simpler faster stronger. In Conference on
Computer Vision and Pattern Recognition (CVPR).
Yan, X., Gao, J., Zheng, C., Zheng, C., Zhang, R., Cui, S.,
and Li, Z. (2022). 2dpass: 2d priors assisted seman-
tic segmentation on lidar point clouds. In European
Conference on Computer Vision (ECCV).
Yilmaz, K., Schult, J., Nekrasov, A., and Leibe, B. (2024).
Mask4former: Mask transformer for 4d panoptic seg-
mentation. In International Conference on Robotics
and Automation (ICRA).
Zhu, M., Han, S., Cai, H., Borse, S., Ghaffari, M., and
Porikli, F. (2023). 4d panoptic segmentation as invari-
ant and equivariant field prediction. In International
Conference on Computer Vision (ICCV).
VISAPP 2025 - 20th International Conference on Computer Vision Theory and Applications
650