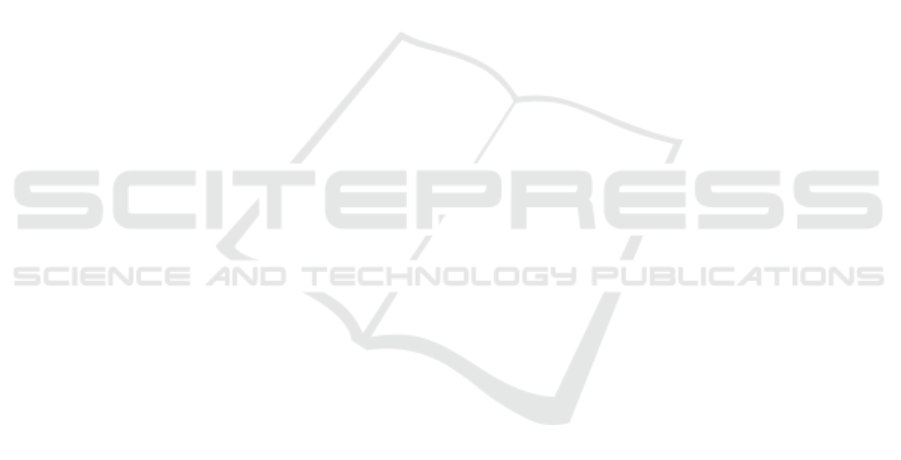
ACKNOWLEDGEMENTS
This work was supported in part by the National Sci-
ence Foundation grants 2417510 and 2309564.
REFERENCES
Amazon (2016). Amazon AWS Polly.
Andujar, C. and Argelaguet, F. (2007). Anisomorphic
ray-casting manipulation for interacting with 2d guis.
Computers & Graphics, 31(1):15–25.
Argelaguet, F. and Andujar, C. (2013). A survey of 3d
object selection techniques for virtual environments.
Computers & Graphics, 37(3):121–136.
Argelaguet, F., Andujar, C., and Trueba, R. (2008). Over-
coming eye-hand visibility mismatch in 3d pointing
selection. In Proceedings of the 2008 ACM sympo-
sium on Virtual reality software and technology, pages
43–46.
Brown, T., Mann, B., Ryder, N., Subbiah, M., Kaplan, J. D.,
Dhariwal, P., Neelakantan, A., Shyam, P., Sastry, G.,
Askell, A., et al. (2020). Language models are few-
shot learners. Advances in neural information pro-
cessing systems, 33:1877–1901.
Buchta, K., W
´
ojcik, P., Nakonieczny, K., Janicka, J.,
Gałuszka, D., Sterna, R., and Igras-Cybulska, M.
(2022a). Microtransactions in vr. a qualitative com-
parison between voice user interface and graphical
user interface. In 2022 15th International Conference
on Human System Interaction (HSI), pages 1–5.
Buchta, K., W
´
ojcik, P., Nakonieczny, K., Janicka, J.,
Gałuszka, D., Sterna, R., and Igras-Cybulska, M.
(2022b). Modeling and optimizing the voice assistant
behavior in virtual reality. In 2022 IEEE International
Symposium on Mixed and Augmented Reality Adjunct
(ISMAR-Adjunct), pages 397–402.
Buchta, K., W
´
ojcik, P., Pelc, M., G
´
orowska, A., Mota, D.,
Boichenko, K., Nakonieczny, K., Wrona, K., Szym-
czyk, M., Czuchnowski, T., Janicka, J., Gałuszka, D.,
Sterna, R., and Igras-Cybulska, M. (2022c). Nux ive -
a research tool for comparing voice user interface and
graphical user interface in vr. In 2022 IEEE Confer-
ence on Virtual Reality and 3D User Interfaces Ab-
stracts and Workshops (VRW), pages 982–983.
Calandra, D., Prattic
`
o, F. G., and Lamberti, F. (2022). Com-
parison of hands-free speech-based navigation tech-
niques for virtual reality training. In 2022 IEEE 21st
Mediterranean Electrotechnical Conference (MELE-
CON), pages 85–90.
Chowdhery, A., Narang, S., Devlin, J., Bosma, M., Mishra,
G., Roberts, A., Barham, P., Chung, H. W., Sut-
ton, C., Gehrmann, S., et al. (2022). Palm: Scal-
ing language modeling with pathways. arXiv preprint
arXiv:2204.02311.
chromadb (2022). Trychroma. version 0.4.12.
Chu, C.-C., Dani, T., and Gadh, R. (1997). Multimodal in-
terface for a virtual reality based computer aided de-
sign system. In Proceedings of International Confer-
ence on Robotics and Automation, volume 2, pages
1329–1334 vol.2.
Chung, H. W., Hou, L., Longpre, S., Zoph, B., Tay, Y., Fe-
dus, W., Li, Y., Wang, X., Dehghani, M., Brahma, S.,
Webson, A., Gu, S. S., Dai, Z., Suzgun, M., Chen, X.,
Chowdhery, A., Castro-Ros, A., Pellat, M., Robinson,
K., Valter, D., Narang, S., Mishra, G., Yu, A., Zhao,
V., Huang, Y., Dai, A., Yu, H., Petrov, S., Chi, E. H.,
Dean, J., Devlin, J., Roberts, A., Zhou, D., Le, Q. V.,
and Wei, J. (2022). Scaling instruction-finetuned lan-
guage models.
Cohen, J. (2013). Statistical power analysis for the behav-
ioral sciences. Academic press.
Fashimpaur, J., Kin, K., and Longest, M. (2020). Pinchtype:
Text entry for virtual and augmented reality using
comfortable thumb to fingertip pinches. In Extended
abstracts of the 2020 CHI conference on human fac-
tors in computing systems, pages 1–7.
Forsberg, A., Herndon, K., and Zeleznik, R. (1996). Aper-
ture based selection for immersive virtual environ-
ments. In Proceedings of the 9th annual ACM sym-
posium on User interface software and technology,
pages 95–96.
G
¨
obl., B., Kriglstein., S., and Hlavacs., H. (2021). Conver-
sational interfaces in serious games: Identifying po-
tentials and future research directions based on a sys-
tematic literature review. In Proceedings of the 13th
International Conference on Computer Supported Ed-
ucation - Volume 1: CSEDU, pages 108–115. IN-
STICC, SciTePress.
Han, X. and Wan, H. (2010). A framework for virtual hand
haptic interaction. Transactions on edutainment IV,
pages 229–240.
Hombeck, J., Voigt, H., Heggemann, T., Datta, R. R., and
Lawonn, K. (2023). Tell me where to go voice-
controlled hands-free locomotion for virtual reality
systems. In 2023 IEEE Conference Virtual Reality and
3D User Interfaces (VR), pages 123–134.
Hutama, W., Harashima, H., Ishikawa, H., and Manabe, H.
(2021). Hmk: Head-mounted-keyboard for text input
in virtual or augmented reality. In Adjunct Proceed-
ings of the 34th Annual ACM Symposium on User In-
terface Software and Technology, pages 115–117.
IBM Corp. (2022). IBM SPSS Statistics [Computer soft-
ware]. Version 27.0.
LangChain (2022). Langchain. version 0.0.299.
Lewis, P., Perez, E., Piktus, A., Petroni, F., Karpukhin,
V., Goyal, N., K
¨
uttler, H., Lewis, M., tau
Yih, W., Rockt
¨
aschel, T., Riedel, S., and Kiela,
D. (2021). Retrieval-augmented generation for
knowledge-intensive nlp tasks.
Li, Z., Zhang, S., Anwar, M. S., and Wang, J. (2018). Appli-
cability analysis on three interaction paradigms in im-
mersive vr environment. In 2018 International Con-
ference on Virtual Reality and Visualization (ICVRV),
pages 82–85.
Loshchilov, I. and Hutter, F. (2017). Fixing weight decay
regularization in adam. CoRR, abs/1711.05101.
Meta (2013). Quest 3: New Mixed Reality Headset. https:
Hands-Free VR
541