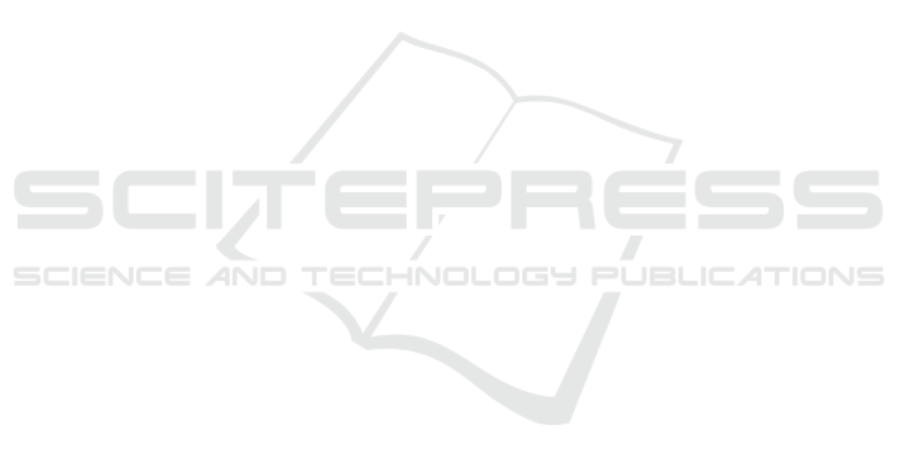
running gait analysis outside of the lab: A systematic
review. Gait and Posture, 63:124–138.
Borz
`
ı, L., Sigcha, L., Rodr
´
ıguez-Mart
´
ın, D., and Olmo,
G. (2023). Real-time detection of freezing of gait
in parkinson’s disease using multi-head convolutional
neural networks and a single inertial sensor. Artificial
Intelligence in Medicine, 135:102459.
BTS Bioengineering (2024a). BTS G-WALK - Wire-
less Sensor for Gait Analysis. https://www.
btsbioengineering.com/products/g-walk/. Accessed:
2024-08-31.
BTS Bioengineering (2024b). BTS GAITLAB - 3D Mo-
tion Analysis System. https://www.btsbioengineering.
com/products/bts-gaitlab/. Accessed: 2024-08-31.
Burdack, J., Horst, F., Giesselbach, S., Hassan, I., Daffner,
S., and Sch
¨
ollhorn, W. I. (2020). Systematic compar-
ison of the influence of different data preprocessing
methods on the performance of gait classifications us-
ing machine learning. Frontiers in Bioengineering and
Biotechnology, 8.
Caldas, R., Sarai, R., Buarque de Lima Neto, F., and Mark-
ert, B. (2020). Validation of two hybrid approaches for
clustering age-related groups based on gait kinematics
data. Medical Engineering & Physics, 78:90–97.
da Rosa Tavares, J., Ullrich, M., Roth, N., Kluge, F.,
Eskofier, B., Gaßner, H., Klucken, J., Gladow, T.,
Marxreiter, F., da Costa, C., da Rosa Righi, R., and
Vict
´
oria Barbosa, J. (2023). utug: An unsupervised
timed up and go test for parkinson’s disease. Biomed-
ical Signal Processing and Control, 81:104394.
Delval, A., Betrouni, N., Tard, C., Devos, D., Dujardin,
K., Defebvre, L., Labidi, J., and Moreau, C. (2021).
Do kinematic gait parameters help to discriminate
between fallers and non-fallers with parkinson’s dis-
ease? Clinical Neurophysiology, 132(2):536–541.
Desai, R., Martelli, D., Alomar, J., Agrawal, S., Quinn, L.,
and Bishop, L. (2024). Validity and reliability of in-
ertial measurement units for gait assessment within a
post stroke population. Topics in Stroke Rehabilita-
tion, 31(3):235–243. PMID: 37545107.
He, Y., Chen, Y., Tang, L., Chen, J., Tang, J., Yang, X., Su,
S., Zhao, C., and Xiao, N. (2024). Accuracy valida-
tion of a wearable imu-based gait analysis in healthy
female. BMC Sports Science, Medicine and Rehabili-
tation, 16(1):2.
Jakob, V., K
¨
uderle, A., Kluge, F., Klucken, J., Eskofier,
B., Winkler, J., Winterholler, M., and Gassner, H.
(2021). Validation of a sensor-based gait analysis sys-
tem with a gold-standard motion capture system in pa-
tients with parkinson’s disease. Sensors, 21(22).
Kim, H., Kim, Y.-H., Kim, S.-J., and Choi, M.-T. (2022).
Pathological gait clustering in post-stroke patients us-
ing motion capture data. Gait & Posture, 94:210–216.
Kotiadis, D., Hermens, H., and Veltink, P. (2010). Inertial
gait phase detection for control of a drop foot stimula-
tor: Inertial sensing for gait phase detection. Medical
Engineering and Physics, 32(4):287–297.
Kvist, A., Tinmark, F., Bezuidenhout, L., Reimeringer, M.,
Conradsson, D., and Franz
´
en, E. (2024). Validation
of algorithms for calculating spatiotemporal gait pa-
rameters during continuous turning using lumbar and
foot mounted inertial measurement units. Journal of
Biomechanics, 162:111907.
Millecamps, A., Lowry, K., Brach, J., Perera, S., Redfern,
M., and Sejdi
´
c, E. (2015). Understanding the effects
of pre-processing on extracted signal features from
gait accelerometry signals. Computers in Biology and
Medicine, 62:164–174.
Nguyen, A., Roth, N., Ghassemi, N., Hannink, J., Seel, T.,
Klucken, J., Gassner, H., and Eskofier, B. (2019). De-
velopment and clinical validation of inertial sensor-
based gait-clustering methods in parkinson’s dis-
ease. Journal of NeuroEngineering and Rehabilita-
tion, 16(1):77.
Parashar, A., Parashar, A., Ding, W., Shabaz, M., and Rida,
I. (2023). Data preprocessing and feature selection
techniques in gait recognition: A comparative study of
machine learning and deep learning approaches. Pat-
tern Recognition Letters, 172:65–73.
Ripic, Z., Nienhuis, M., Signorile, J., Best, T., Ja-
cobs, K., and Eltoukhy, M. (2023). A comparison
of three-dimensional kinematics between markerless
and marker-based motion capture in overground gait.
Journal of Biomechanics, 159:111793.
Rousanoglou, E., Foskolou, A., Emmanouil, A., and
Boudolos, K. (2024). Inertial sensing of the abdomi-
nal wall kinematics during diaphragmatic breathing in
head standing. Biomechanics, 4(1):63–83.
Silva, L. and Stergiou, N. (2020). Chapter 7 - the basics
of gait analysis. In Stergiou, N., editor, Biomechanics
and Gait Analysis, pages 225–250. Academic Press.
Tsakanikas, V., Ntanis, A., Rigas, G., Androutsos,
C., Boucharas, D., Tachos, N., Skaramagkas, V.,
Chatzaki, C., Kefalopoulou, Z., Tsiknakis, M., and
Fotiadis, D. (2023). Evaluating gait impairment in
parkinson’s disease from instrumented insole and imu
sensor data. Sensors, 23(8).
Zhang, Y., Wang, M., Awrejcewicz, J., Fekete, G., Ren, F.,
and Gu, Y. (2017). Using gold-standard gait analy-
sis methods to assess experience effects on lower-limb
mechanics during moderate high-heeled jogging and
running. Journal of visualized experiments : JoVE,
127:55714.
Zhou, L., Tunca, C., Fischer, E., Brahms, C., Ersoy, C.,
Granacher, U., and Arnrich, B. (2020). Validation of
an imu gait analysis algorithm for gait monitoring in
daily life situations. In Annual International Confer-
ence of the IEEE Engineering in Medicine and Biol-
ogy Society (EMBC), pages 4229–4232.
HEALTHINF 2025 - 18th International Conference on Health Informatics
86