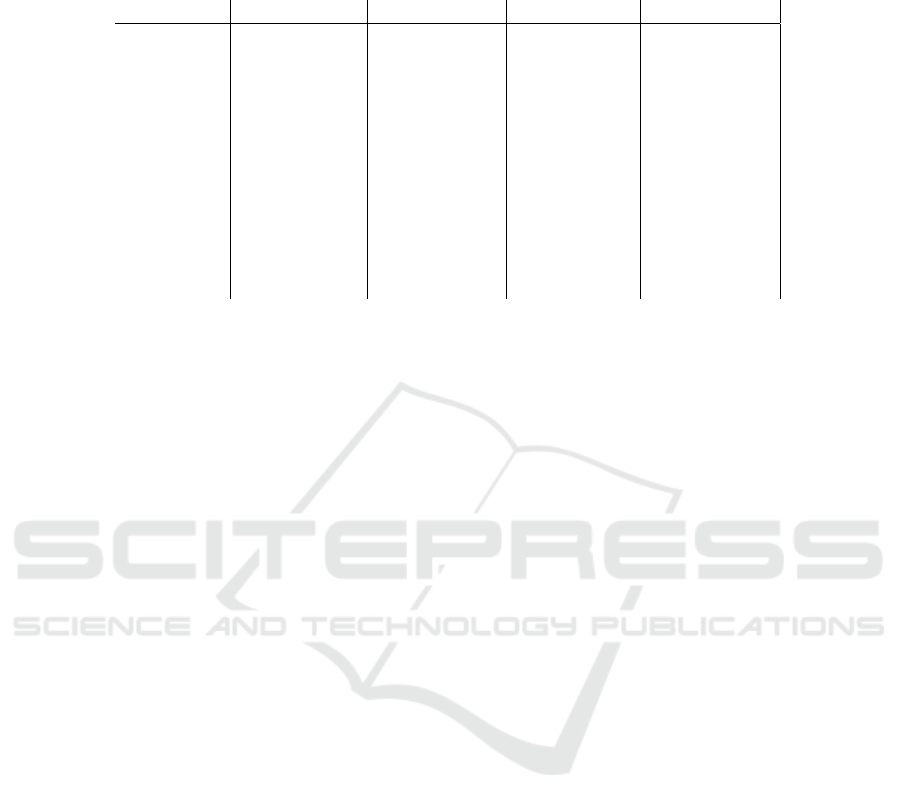
Table 4: Summary statistic error rate over all instances of the used models over the interval (-60, 60). The acquisition error
rate is based on the experiment in Table 3, i.e. the difference between the best obtained angle and the found angle over the
shear data interval.
Object Min. Diff. [°] Max. Diff. [°] Avg. Diff. [°] Med. Diff. [°]
Ant 0.00 1.00 0.25 0.00
Armadillo 0.00 3.40 0.54 0.40
Beetle 0.00 1.40 0.45 0.30
Buddha 0.00 6.10 0.99 0.50
Bunny 0.00 4.60 0.79 0.50
Cow 0.00 3.50 0.43 0.40
Elephant 0.00 1.00 0.25 0.10
Formula 0.00 2.40 0.53 0.40
Homer 0.00 2.00 0.32 0.20
Lion 0.00 1.80 0.50 0.40
Lucy 0.00 3.50 0.60 0.50
Mannequin 0.00 1.50 0.40 0.40
REFERENCES
Bruckstein, A. M. and Shaked, D. (1998). Skew-symmetry
detection via invariant signatures. Pattern Recogni-
tion, 31(2):181–192.
Cham, T.-J. and Cipolla, R. (1995). Symmetry detection
through local skewed symmetries. Image and Vision
Computing, 13(5):439–450.
Chaouch, M. and Verroust-Blondet, A. (2009). Alignment
of 3d models. Graphical Models, 71(2):63–76.
Fang, R., Godil, A., Li, X., and Wagan, A. (2008). A new
shape benchmark for 3d object retrieval. In Interna-
tional Symposium on Visual Computing, pages 381–
392. Springer.
Friedberg, S. A. (1986). Finding axes of skewed symme-
try. Comput. Vision Graphics Image Process., 34:138–
155.
Gross, A. D. and Boult, T. E. (1994). Analyzing skewed
symmetries. Int. J. Comput. Vision, 13:91–111.
Hruda, L., Kolingerov
´
a, I., and V
´
a
ˇ
sa, L. (2020). Robust, fast
and flexible symmetry plane detection based on differ-
entiable symmetry measure: Supplementary material.
Available at http://meshcompression.org/tvcj-2020.
Hruda, L., Kolingerov
´
a, I., and V
´
a
ˇ
sa, L. (2022). Robust, fast
and flexible symmetry plane detection based on differ-
entiable symmetry measure. Visual Comput., 38:555–
571.
Ji, P. and Liu, X. (2019). A fast and efficient 3d reflection
symmetry detector based on neural networks. Multi-
media Tools and Applications, 78(24):35471–35492.
Ji, S., Ren, Y., Ji, Z., Liu, X., and Hong, X. (2017). An im-
proved method for registration of point cloud. Optik,
140:451–458.
Levoy, M., Gerth, J., Curless, B., and Pull, K. (2005).
The stanford 3d scanning repository. Available at
http://www.graphics.stanford.edu/data/3Dscanrep/.
Li, B., Johan, H., Ye, Y., and Lu, Y. (2016). Efficient 3d re-
flection symmetry detection: A view-based approach.
Graphical Models, 83:2–14.
Martinet, A., Soler, C., Holzschuch, N., and Sillion,
F. X. (2006). Accurate detection of symmetries in
3d shapes. ACM Transactions on Graphics (TOG),
25(2):439–464.
Mitra, N. J., Pauly, M., Wand, M., and Ceylan, D. (2013).
Symmetry in 3d geometry: Extraction and applica-
tions. Computer Graphics Forum, 32(6):1–23.
Mitra, S. and Liu, Y. (2004). Local facial asymmetry for
expression classification. In CVPR.
Podolak, J., Golovinskiy, A., and Rusinkiewicz, S. (2007).
Symmetry-enhanced remeshing of surfaces. In Pro-
ceedings of the Fifth Eurographics Symposium on Ge-
ometry Processing (SGP ’07), pages 235–242. Euro-
graphics Association.
Schiebener, D., Schmidt, A., Vahrenkamp, N., and As-
four, T. (2016). Heuristic 3d object shape completion
based on symmetry and scene context. In Intelligent
Robots and Systems (IROS), 2016 IEEE/RSJ Interna-
tional Conference on, pages 74–81. IEEE.
Shilane, P., Min, P., Kazhdan, M., and Funkhouser, T.
(2004). The princeton shape benchmark. In Shape
Modeling Applications, 2004. Proceedings, pages
167–178. IEEE.
Shu, Q., He, X., Wang, C., Yang, Y., and Cui, Z. (2021).
Fast point cloud registration in multidirectional affine
transformation. Optik, 229:165884.
Simari, P., Kalogerakis, E., and Singh, K. (2006). Fold-
ing meshes: Hierarchical mesh segmentation based on
planar symmetry. In Symposium on Geometry Pro-
cessing, volume 256, pages 111–119.
Sipiran, I. (2017). Analysis of partial axial symmetry on 3d
surfaces and its application in the restoration of cul-
tural heritage objects. In Proceedings of the IEEE
International Conference on Computer Vision Work-
shops, pages 2925–2933.
Sun, C. and Sherrah, J. (1997). 3d symmetry detection
using the extended gaussian image. IEEE Transac-
tions on Pattern Analysis and Machine Intelligence,
19(2):164–168.
GRAPP 2025 - 20th International Conference on Computer Graphics Theory and Applications
210