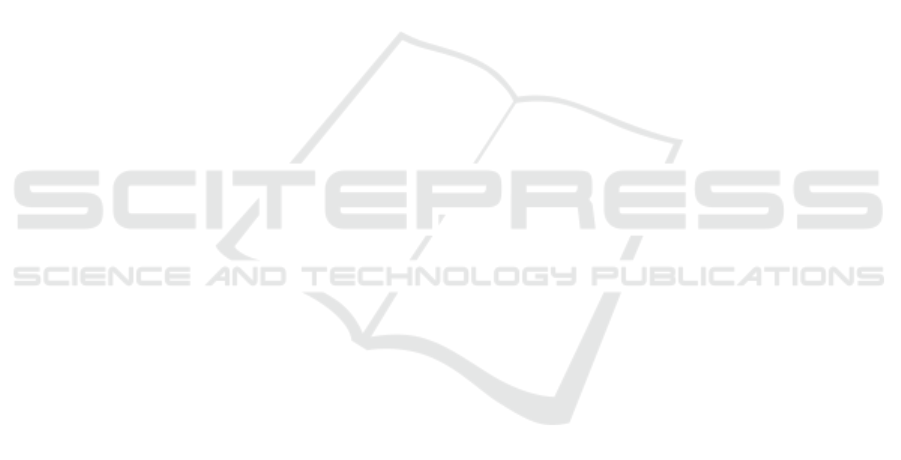
space dimensionality and reconstruction performance
across models. These results are corroborated by the
t-SNE plots where significant divergence is observed
between clean and noisy points in the CAE and DAE,
while such points for the VAE are tightly clustered
near the origin.
Much work remains to be done. We plan to extend
this analysis to other autoencoder variants and vali-
date the results with more datasets. Further, we also
plan to characterize the latent spaces of other genera-
tive models. As a constructive next step, we intend to
devise denoising and deblurring algorithms by lever-
aging the understanding of the manifold structure of
autoencoders, particularly VAEs.
REFERENCES
Abdelkader, M. F., Abd-Almageed, W., Srivastava, A., and
Chellappa, R. (2011). Silhouette-based gesture and
action recognition via modeling trajectories on Rie-
mannian shape manifolds. Computer Vision and Im-
age Understanding, 115(3):439–455.
Bengio, Y., Courville, A., and Vincent, P. (2013). Represen-
tation learning: A review and new perspectives. IEEE
Trans. on Pattern Analysis and Machine Intelligence,
35(8):1798–1828.
Berthelot, D., Raffel, C., Roy, A., and Goodfellow, I.
(2018). Understanding and improving interpola-
tion in autoencoders via an adversarial regularizer.
arXiv:1807.07543.
Bonnabel, S. and Sepulchre, R. (2010). Riemannian metric
and geometric mean for positive semidefinite matrices
of fixed rank. SIAM Journal on Matrix Analysis and
Applications, 31(3):1055–1070.
Chadebec, C. and Allassonnière, S. (2022). A geomet-
ric perspective on variational autoencoders. Advances
in Neural Information Processing Systems, 35:19618–
19630.
Chen, N., Klushyn, A., Ferroni, F., Bayer, J., and Van
Der Smagt, P. (2020). Learning flat latent manifolds
with VAEs. arXiv:2002.04881.
Connor, M., Canal, G., and Rozell, C. (Apr. 2021). Vari-
ational autoencoder with learned latent structure. In
Proc. AISTATS, Online.
Cristovao, P., Nakada, H., Tanimura, Y., and Asoh,
H. (2020). Generating in-between images through
learned latent space representation using variational
autoencoders. IEEE Access, 8:149456–149467.
Doersch, C. (2016). Tutorial on variational autoencoders.
arXiv:1606.05908.
Fefferman, C., Mitter, S., and Narayanan, H. (2016). Test-
ing the manifold hypothesis. Journal of the American
Mathematical Society, 29(4):983–1049.
Kingma, D. P. and Welling, M. (2013). Auto-encoding vari-
ational Bayes. arXiv:1312.6114.
Knyazev, A. V. and Zhu, P. (2012). Principal angles between
subspaces and their tangents. arXiv:1209.0523.
Lee, J. M. and Lee, J. M. (2012). Smooth manifolds.
Springer.
Leeb, F., Bauer, S., Besserve, M., and Schölkopf, B. (2022).
Exploring the latent space of autoencoders with in-
terventional assays. Advances in Neural Information
Processing Systems, 35:21562–21574.
Lui, Y. M. (2012). Human gesture recognition on prod-
uct manifolds. The Journal of Machine Learning Re-
search, 13(1):3297–3321.
Lui, Y. M., Beveridge, J. R., and Kirby, M. (Jun. 2010).
Action classification on product manifolds. In Proc.
IEEE/CVF CVPR, San Francisco, CA.
Massart, E., Hendrickx, J. M., and Absil, P.-A. (Aug. 2019).
Curvature of the manifold of fixed-rank positive-
semidefinite matrices endowed with the Bures–
Wasserstein metric. In Proc. Geometric Science of
Information Science (GSI), Toulouse, France.
Ng, A. et al. (2011). Sparse autoencoder. CS294A Lecture
notes, 72(2011):1–19.
Oring, A. (2021). Autoencoder image interpolation by
shaping the latent space. Master’s thesis, Reichman
University (Israel).
Rifai, S., Vincent, P., Muller, X., Glorot, X., and Bengio,
Y. (Jun. 2011). Contractive autoencoders: Explicit
invariance during feature extraction. In Proc. ICML,
Bellevue, WA.
Rodolà, E., Lähner, Z., Bronstein, A. M., Bronstein, M. M.,
and Solomon, J. (2019). Functional maps represen-
tation on product manifolds. In Computer Graphics
Forum. Wiley Online Library, pages 678–689.
Rumelhart, D. E., Hinton, G. E., and Williams, R. J. (1986).
Learning representations by back-propagating errors.
Nature, 323(6088):533–536.
Sharma, K. and Rameshan, R. (2021). Distance based ker-
nels for video tensors on product of Riemannian ma-
trix manifolds. Journal of Visual Communication and
Image Representation, 75:103045.
Sharma, K. and Rameshan, R. (May 2019). Linearized ker-
nel representation learning from video tensors by ex-
ploiting manifold geometry for gesture recognition. In
Proc. ICASSP, Brighton, UK.
Takatsu, A. (2011). Wasserstein geometry of Gaussian mea-
sures. Osaka J. Math, 48(4):1055–1026.
Van der Maaten, L. and Hinton, G. (2008). Visualizing data
using t-SNE. Journal of Machine Learning Research,
9(11).
Vincent, P., Larochelle, H., Bengio, Y., and Manzagol, P.-
A. (Jun. 2008). Extracting and composing robust fea-
tures with denoising autoencoders. In Proc. ICML,
Helsinki, Finland.
Zhai, J., Zhang, S., Chen, J., and He, Q. (Oct. 2018). Au-
toencoder and its various variants. In Proc. IEEE
SMC, Miyazaki, Japan.
Zhang, K., Lan, L., Wang, Z., and Moerchen, F. (Apr.
2012). Scaling up kernel SVM on limited resources:
A low-rank linearization approach. In Proc. AISTATS,
La Palma, Canary Islands.
Latent Space Characterization of Autoencoder Variants
67