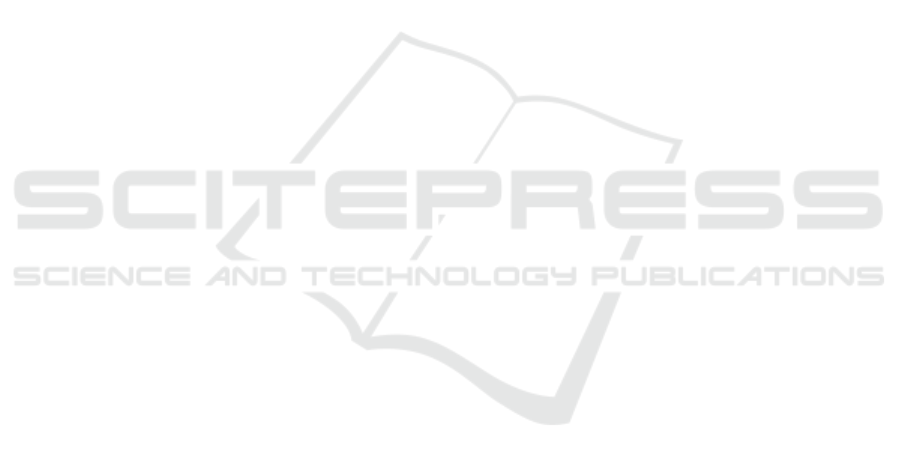
heiro, J., and Casanova, M. (2024). Improving the
accuracy of text-to-sql tools based on large language
models for real-world relational databases. In Strauss,
C., Amagasa, T., Manco, G., Kotsis, G., Tjoa, A.,
and Khalil, I., editors, Database and Expert Systems
Applications, pages 93–107, Cham. Springer Nature
Switzerland.
Dong, X., Zhang, C., Ge, Y., Mao, Y., Gao, Y.,
Chen, L., Lin, J., and Lou, D. (2023). C3:
Zero-shot text-to-sql with chatgpt. arXiv preprint.
https://doi.org/10.48550/arXiv.2307.07306.
Floratou, A. et al. (2024). Nl2sql is a solved problem... not!
In Conference on Innovative Data Systems Research.
Garc
´
ıa, G., Izquierdo, Y., Menendez, E., Dartayre, F.,
and Casanova, M. (2017). Rdf keyword-based query
technology meets a real-world dataset. In Proceed-
ings of the 20th International Conference on Extend-
ing Database Technology (EDBT), pages 656–667,
Venice, Italy. OpenProceedings.org.
Izquierdo, Y., Garc
´
ıa, G., Lemos, M., Novello, A., Nov-
elli, B., Damasceno, C., Leme, L., and Casanova, M.
(2021). A platform for keyword search and its appli-
cation for covid-19 pandemic data. Journal of Infor-
mation and Data Management, 12(5):521–535.
Izquierdo, Y., Lemos, M., Oliveira, C., Novelli, B., Garc
´
ıa,
G., Coelho, G., Feij
´
o, L., Coutinho, B., Santana, T.,
Garcia, R., and Casanova, M. (2024). Busca360: A
search application in the context of top-side asset in-
tegrity management in the oil & gas industry. In Anais
do XXXIX Simp
´
osio Brasileiro de Bancos de Dados,
pages 104–116, Porto Alegre, RS, Brasil. SBC.
Katsogiannis-Meimarakis, G. and Koutrika, G. (2023). A
survey on deep learning approaches for text-to-sql.
The VLDB Journal, 32(4):905–936.
Kim, H., So, B.-H., Han, W.-S., and Lee, H. (2020). Natural
language to sql: Where are we today? Proc. VLDB
Endow., 13(10):1737–1750.
Lei, F. et al. (2024). Spider 2.0: Evaluat-
ing language models on real-world enter-
prise text-to-sql workflows. arXiv preprint.
https://doi.org/10.48550/arXiv.2411.07763.
Li, J., Hui, B., Qu, G., Yang, J., Li, B., Li, B., Wang, B.,
Qin, B., Geng, R., Huo, N., Zhou, X., Ma, C., Li, G.,
Chang, K., Huang, F., Cheng, R., and Li, Y. (2024).
Can llm already serve as a database interface? a big
bench for large-scale database grounded text-to-sqls.
In Proceedings of the 37th International Conference
on Neural Information Processing Systems, NIPS ’23,
Red Hook, NY, USA. Curran Associates Inc.
Nascimento, E., Garc
´
ıa, G., Feij
´
o, L., Victorio, W.,
Izquierdo, Y., Oliveira, A., Coelho, G., M., L., Garcia,
R., Leme, L., and Casanova, M. (2024a). Text-to-sql
meets the real-world. In Proceedings of the 26th Inter-
national Conference on Enterprise Information Sys-
tems - Volume 1: ICEIS, pages 61–72, Set
´
ubal, Portu-
gal. INSTICC, SciTePress.
Nascimento, E., Izquierdo, Y., Garc
´
ıa, G., Coelho, G.,
Feij
´
o, L., Lemos, M., Leme, L., and M.A., C.
(2024b). My database user is a large language model.
In Proceedings of the 26th International Confer-
ence on Enterprise Information Systems - Volume 1:
ICEIS, pages 800–806, Set
´
ubal, Portugal. INSTICC,
SciTePress.
Oliveira, A., Nascimento, E., Pinheiro, J., Avila, C., Coelho,
G., Feij
´
o, L., Izquierdo, Y., Garc
´
ıa, G., Leme, L.,
Lemos, M., and Casanova, M. (2025). Small, medium,
and large language models for text-to-sql. In Maass,
W., Han, H., Yasar, H., and Multari, N., editors, Con-
ceptual Modeling, pages 276–294, Cham. Springer
Nature Switzerland.
Pourreza, M. and Rafiei, D. (2024). Din-sql: decomposed
in-context learning of text-to-sql with self-correction.
In Proceedings of the 37th International Conference
on Neural Information Processing Systems, NIPS ’23,
Red Hook, NY, USA. Curran Associates Inc.
Shi, L., Tang, Z., Zhang, N., Zhang, X., and Yang,
Z. (2024). A survey on employing large lan-
guage models for text-to-sql tasks. arXiv preprint.
https://doi.org/10.48550/arXiv.2407.15186.
Yu, T., Zhang, R., Yang, K., Yasunaga, M., Wang, D., Li,
Z., Ma, J., Li, I., Yao, Q., Roman, S., Zhang, Z.,
and Radev, D. (2018). Spider: A large-scale human-
labeled dataset for complex and cross-domain seman-
tic parsing and text-to-sql task. In Riloff, E., Chiang,
D., Hockenmaier, J., and Tsujii, J., editors, Proc. 2018
Conference on Empirical Methods in Natural Lan-
guage Processing, pages 3911–3921, Brussels, Bel-
gium. Association for Computational Linguistics.
ICEIS 2025 - 27th International Conference on Enterprise Information Systems
180