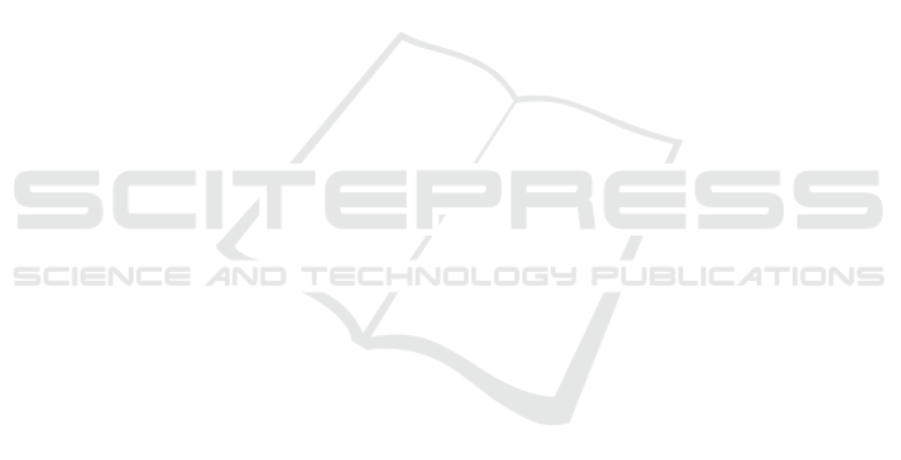
IEEE/RSJ International Conference on Intelligent
Robots and Systems (IROS), pages 14056–14062.
IEEE.
Kianfar, R., Ali, M., Falcone, P., and Fredriksson, J. (2014).
Combined longitudinal and lateral control design for
string stable vehicle platooning within a designated
lane. In 17th International IEEE Conference on In-
telligent Transportation Systems (ITSC), pages 1003–
1008.
Kwon, J.-W. and Chwa, D. (2014). Adaptive bidirectional
platoon control using a coupled sliding mode control
method. IEEE Transactions on Intelligent Transporta-
tion Systems, 15(5):2040–2048.
Li, S. E., Zheng, Y., Li, K., Wu, Y., Hedrick, J. K., Gao,
F., and Zhang, H. (2017). Dynamical modeling and
distributed control of connected and automated vehi-
cles: Challenges and opportunities. IEEE Intelligent
Transportation Systems Magazine, 9(3):46–58.
Ma, K., Wang, H., and Ruan, T. (2021). Analysis of road
capacity and pollutant emissions: Impacts of con-
nected and automated vehicle platoons on traffic flow.
Physica A: Statistical Mechanics and its Applications,
583:126301.
Negenborn, R. and Maestre, J. (2014). Distributed model
predictive control: An overview and roadmap of fu-
ture research opportunities. IEEE Control Systems
Magazine, 34(4):87–97.
Rajamani, R., Tan, H.-S., Law, B. K., and Zhang, W.-B.
(2000). Demonstration of integrated longitudinal and
lateral control for the operation of automated vehicles
in platoons. IEEE Transactions on Control Systems
Technology, 8(4):695–708.
Wei, S., Zou, Y., Zhang, X., Zhang, T., and Li, X. (2019).
An integrated longitudinal and lateral vehicle follow-
ing control system with radar and vehicle-to-vehicle
communication. IEEE Transactions on Vehicular
Technology, 68(2):1116–1127.
Wu, J., Wang, Y., and Yin, C. (2022). Curvilinear multi-
lane merging and platooning with bounded control in
curved road coordinates. IEEE Transactions on Vehic-
ular Technology, 71(2):1237–1252.
Zeng, J., Qian, Y., Li, J., Zhang, Y., and Xu, D. (2023).
Congestion and energy consumption of heterogeneous
traffic flow mixed with intelligent connected vehicles
and platoons. Physica A: Statistical Mechanics and its
Applications, 609:128331.
Zhao, H., Sun, D., Zhao, M., Pu, Q., and Tang, C. (2022).
Combined longitudinal and lateral control for hetero-
geneous nodes in mixed vehicle platoon under v2i
communication. IEEE Transactions on Intelligent
Transportation Systems, 23(7):6751–6765.
Zheng, Y., Li, S. E., Li, K., Borrelli, F., and Hedrick,
J. K. (2017). Distributed model predictive control for
heterogeneous vehicle platoons under unidirectional
topologies. IEEE Transactions on Control Systems
Technology, 25(3):899–910.
Zuo, Z., Yang, K., Wang, H., Wang, Y., and Wu, Y. (2024).
Distributed mpc for automated vehicle platoon: A
path-coupled extended look-ahead approach. IEEE
Transactions on Intelligent Vehicles, pages 1–13.
Coupled Lateral and Longitudinal Control for Vehicle Platoons on Curved Roads
293