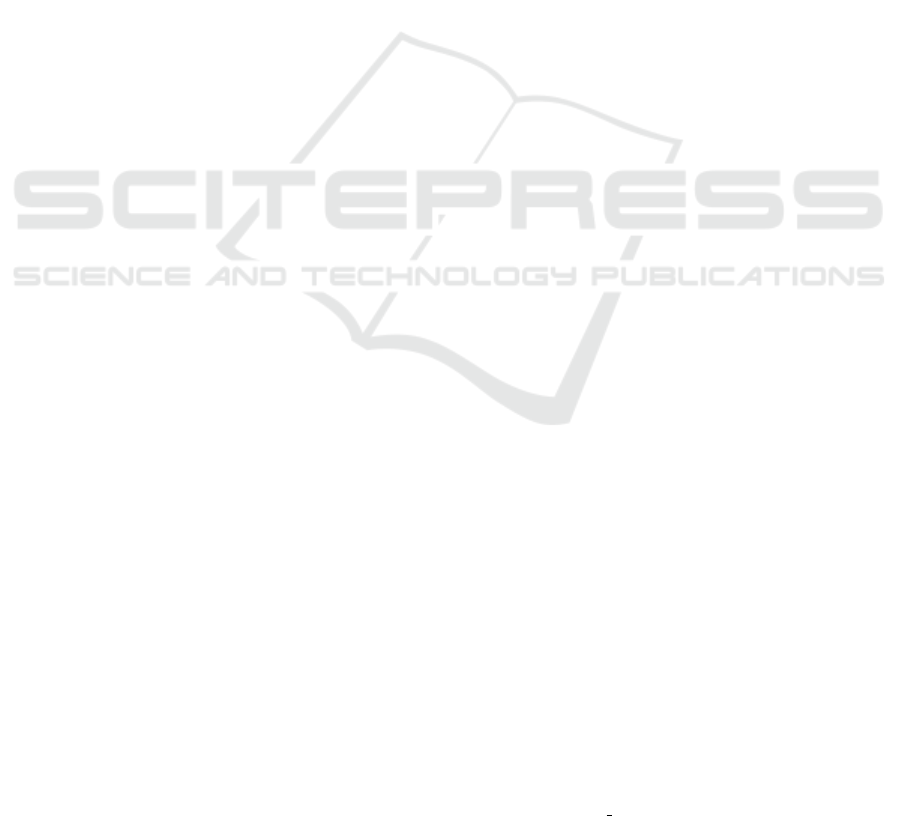
Cabral, B. and Leedom, L. C. (1993). Imaging vector fields
using line integral convolution. In ACM SIGGRAPH,
pages 263–270. DOI: 10.1145/166117.166151.
Calvetti, D., Morigi, S., Reichel, L., and Sgallari, F. (2000).
Tikhonov regularization and the l-curve for large dis-
crete ill-posed problems. Journal of Computational
and Applied Mathematics, 123(1):423–446. Numeri-
cal Analysis 2000. Vol. III: Linear Algebra.
Catmull, E. and Rom, R. (1974). A class of local interpo-
lating splines. In Computer Aided Geometric Design,
pages 317–326.
Chow, E., Anzt, H., Scott, J., and Dongarra, J. (2018). Us-
ing jacobi iterations and blocking for solving sparse
triangular systems in incomplete factorization precon-
ditioning. Journal of Parallel and Distributed Com-
puting, 119:219–230.
Dai, B., Ding, S., and Wahba, G. (2013). Multivariate
Bernoulli distribution. Bernoulli, 19(4):1465–1482.
Daßler, N. and G
¨
unther, T. (2024). Variational feature ex-
traction in scientific visualization. ACM Transactions
on Graphics (TOG), 43(4):1–16.
Delmarcelle, T. and Hesselink, L. (2023). A unified frame-
work for flow visualization. In Computer Visualiza-
tion, pages 129–170. CRC Press.
Farin, G. (2001). Curves and Surfaces for CAGD: A Prac-
tical Guide. Morgan Kaufmann, 5
th
edition. (Chapter
11).
Forsell, L. and Cohen, S. (1995). Using line integral
convolution for flow visualization: curvilinear grids,
variable-speed animation, and unsteady flows. IEEE
Transactions on Visualization and Computer Graph-
ics, 1(2):133–141. DOI: 10.1109/2945.468406.
Garth, C., Gerhardt, F., Tricoche, X., and Hans, H. (2007).
Efficient computation and visualization of coherent
structures in fluid flow applications. IEEE Trans-
actions on Visualization and Computer Graphics,
13(6):1464–1471. DOI: 10.1109/TVCG.2007.70551.
Goualard, F. (2022). Drawing random floating-point num-
bers from an interval. ACM Transactions on Modeling
and Computer Simulation (TOMACS), 32(3):1–24.
G
¨
unther, T. (2020). Visibility, topology, and inertia:
New methods in flow visualization. IEEE Computer
Graphics and Applications, 40(2):103–111. DOI:
10.1109/10.1109/MCG.2019.2959568.
G
¨
unther, T., Gross, M., and Theisel, H. (2017). Generic
objective vortices for flow visualization. ACM
Transactions on Graphics, 36(4):Art. #141. DOI:
10.1145/3072959.3073684.
G
¨
unther, T. and Theisel, H. (2018). The state of the
art in vortex extraction. Computer Graphics Forum,
37(6):149–173. DOI: 10.1111/cgf.13319.
G
¨
unther, T. and Theisel, H. (2024). Objective lagrangian
vortex cores and their visual representations. IEEE
Transactions on Visualization and Computer Graph-
ics.
Hadwiger, M., Mlejnek, M., Theußl, T., and Rautek, P.
(2019). Time-dependent flow seen through approx-
imate observer killing fields. IEEE Transactions on
Visualization and Computer Graphics, 25(1):1257–
1266. DOI: 10.1109/TVCG.2018.2864839.
Hanser, K., Meggendorfer, S., H
¨
ugel, P., Fallenb
¨
uchel,
F., Fahad, H. M., and Sadlo, F. (2019). Energy-
based visualization of 2d flow fields. In VISI-
GRAPP (3: IVAPP), pages 250–258. DOI:
10.5220/0007359602500258.
Hofmann, L. and Sadlo, F. (2021). Local extraction of 3d
time-dependent vector field topology. In Computer
Graphics Forum, volume 40, pages 111–122. Wiley
Online Library.
Inouye, D. I., Yang, E., Allen, G. I., and Ravikumar, P.
(2017). A review of multivariate distributions for
count data derived from the poisson distribution. Wi-
ley Interdisciplinary Reviews: Computational Statis-
tics, 9(3):e1398.
Interrante, V. (1997). Illustrating surface shape in vol-
ume data via principal direction-driven 3D line inte-
gral convolution. In ACM SIGGRAPH, pages 109—-
116. DOI: 10.1145/258734.258796.
Jakob, J., Gross, M., and G
¨
unther, T. (2021). A fluid flow
data set for machine learning and its application to
neural flow map interpolation. IEEE Transactions on
Visualization and Computer Graphics, 27(2):1279–
1289. DOI: 10.1109/TVCG.2020.3028947.
Jenny, B. (2021). Terrain generalization with line
integral convolution. Cartography and Geo-
graphic Information Science”, 48(1):78–92. DOI:
10.1080/15230406.2020.1833762.
Kamgar-Parsi, B., Kamgar-Parsi, B., and Rosenfeld, A.
(1999). Optimally isotropic laplacian operator. IEEE
Transactions on Image Processing, 8(10):1467–1472.
DOI: 10.1109/83.791975.
Kim, B. and G
¨
unther, T. (2019). Robust reference frame
extraction from unsteady 2D vector fields with convo-
lutional neural networks. Computer Graphics Forum,
38(3):285–295. DOI: 10.1111/cgf.13689.
Laramee, R. S., Erlebacher, G., Garth, C., Schafhitzel,
T., Theisel, H., Tricoche, X., Weinkauf, T., and
Weiskopf, D. (2008). Applications of texture-based
flow visualization. Engineering Applications of Com-
putational Fluid Mechanics, 2(3):264–274. DOI:
10.1080/19942060.2008.11015227.
Lawonn, K., Krone, M., Ertl, T., and Preim, B. (2014).
Line integral convolution for real-time illustration
of molecular surface shape and salient regions.
Computer Graphics Forum, 33(3):181–190. DOI:
10.1111/cgf.12374.
MacDonald, C. B. and Ruuth, S. J. (2008). Level
set equations on surfaces via the closest point
method. Scientific Computing, 35(2–3):219–240.
DOI: 10.1007/s10915-008-9196-6.
Matsumoto, M. and Nishimura, T. (1998). Mersenne
twister: a 623-dimensionally equidistributed uniform
pseudo-random number generator. ACM Transactions
on Modeling and Computer Simulation (TOMACS),
8(1):3–30.
McGraw, T., Vemuri, B. C., Wang, Z., Chen, Y., Rao, M.,
and Mareci, T. (2002). Line integral convolution for
visualization of fiber tract maps from DTI. In Med-
ical Image Computing and Computer-Assisted Inter-
vention (MICCAI), pages 615–622. DOI: 10.1007/3-
540-45787-9
77.
LIC-R: Line Integral Convolution Revisited
885