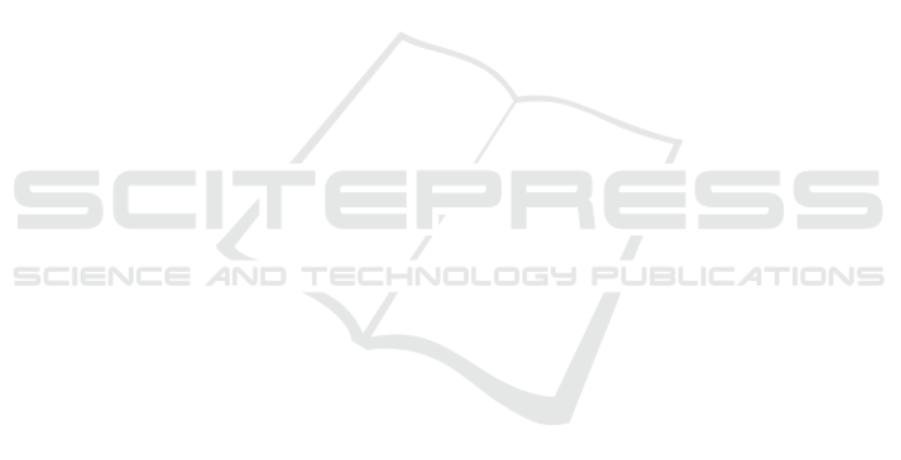
this study, can be effective strategies.
REFERENCES
Belkin, M., Hsu, D., Ma, S., and Mandal, S. (2019). Recon-
ciling modern machine-learning practice and the clas-
sical bias–variance trade-off. Proc. Natl. Acad. Sci.
U.S.A., 116(32):15849–15854.
Cherkassky, V. and Lee, E. H. (2024). To understand dou-
ble descent, we need to understand vc theory. Neural
Networks, 169:242–256.
Defferrard, M., Bresson, X., and Vandergheynst, P. (2016).
Convolutional neural networks on graphs with fast lo-
calized spectral filtering. In Advances in Neural Infor-
mation Processing Systems (NeurIPS), volume 29.
Derezinski, M., Liang, F. T., and Mahoney, M. W.
(2020). Exact expressions for double descent and
implicit regularization via surrogate random design.
In Larochelle, H., Ranzato, M., Hadsell, R., Balcan,
M., and Lin, H., editors, Advances in Neural Infor-
mation Processing Systems, volume 33, pages 5152–
5164. Curran Associates, Inc.
Geiger, M., Jacot, A., Spigler, S., Gabriel, F., Sagun, L.,
d’Ascoli, S., Biroli, G., Hongler, C., and Wyart, M.
(2020). Scaling description of generalization with
number of parameters in deep learning. J. Stat. Mech.
Theory Exp., 2020(2):023401.
Geman, S., Bienenstock, E., and Doursat, R. (1992). Neu-
ral networks and the bias/variance dilemma. Neural
Comput., 4(1):1–58.
Guo, Y. (2018). A survey on methods and theo-
ries of quantized neural networks. arXiv preprint
arXiv:1808.04752.
Hamilton, W., Ying, Z., and Leskovec, J. (2017). Inductive
representation learning on large graphs. In Advances
in Neural Information Processing Systems (NeurIPS),
volume 30.
Hasani, R., Lechner, M., Amini, A., Rus, D., and Grosu, R.
(2021). Liquid time-constant networks. In Proc. AAAI
Conf. Artif. Intell., volume 35, pages 7657–7666.
Hastie, T., Montanari, A., Rosset, S., and Tibshirani, R. J.
(2022). Surprises in high-dimensional ridgeless least
squares interpolation. Ann. Stat., 50(2):949–986.
Hastie, T., Tibshirani, R., and Friedman, J. (2001). The Ele-
ments of Statistical. Learning, Data Mining, Inference
and Prediction, volume 1.
Hubara, I., Courbariaux, M., Soudry, D., El-Yaniv, R., and
Bengio, Y. (2018). Quantized neural networks: Train-
ing neural networks with low precision weights and
activations. J. Mach. Learn. Res., 18(187):1–30.
Kipf, T. N. and Welling, M. (2017). Semi-supervised clas-
sification with graph convolutional networks. In Proc.
Int. Conf. Learn. Represent. (ICLR).
Lafon, M. and Thomas, A. (2024). Understanding the
double descent phenomenon in deep learning. arXiv
preprint arXiv:2403.10459.
Nakkiran, P., Kaplun, G., Bansal, Y., Yang, T., Barak, B.,
and Sutskever, I. (2021). Deep double descent: Where
bigger models and more data hurt. J. Stat. Mech. The-
ory Exp., 2021(12):124003.
Neal, B., Mittal, S., Baratin, A., Tantia, V., Scicluna, M.,
Lacoste-Julien, S., and Mitliagkas, I. (2018). A mod-
ern take on the bias-variance tradeoff in neural net-
works. arXiv preprint arXiv:1810.08591.
Pagliardini, M., Gupta, P., and Jaggi, M. (2018). Un-
supervised learning of sentence embeddings us-
ing compositional n-gram features. arXiv preprint
arXiv:1703.02507.
Pezeshki, M., Mitra, A., Bengio, Y., and Lajoie, G. (2022).
Multi-scale feature learning dynamics: Insights for
double descent. In International Conference on Ma-
chine Learning, pages 17669–17690. PMLR.
Salakhutdinov, R. (2017). Deep learning tu-
torial at the simons institute, berkeley.
Available: https://simons.berkeley.edu/talks/
ruslan-salakhutdinov-01-26-2017-1.
Shi, C., Pan, L., Hu, H., and Dokmani
´
c, I. (2024). Ho-
mophily modulates double descent generalization in
graph convolution networks. Proc. Natl. Acad. Sci.
U.S.A., 121(8):e2309504121.
Tavanaei, A., Ghodrati, M., Kheradpisheh, S. R., Masque-
lier, T., and Maida, A. (2019). Deep learning in spik-
ing neural networks. Neural Netw., 111:47–63.
Veli
ˇ
ckovi
´
c, P., Cucurull, G., Casanova, A., Romero, A., Li
`
o,
P., and Bengio, Y. (2017). Graph attention networks.
arXiv preprint arXiv:1710.10903.
Double Descent Phenomenon in Liquid Time-Constant Networks, Quantized Neural Networks and Spiking Neural Networks
359