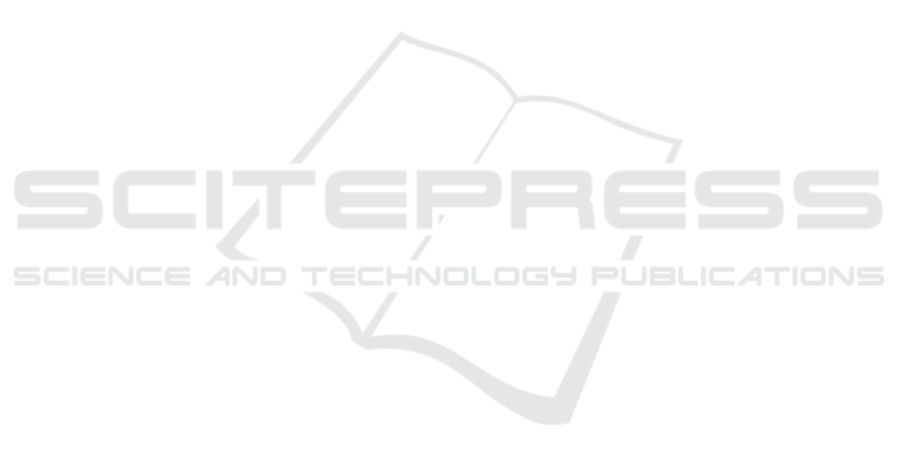
Baur, D. and Stephaner, M. (2018). Everything except the
chart. http://webvis-do.minik.us/. Accessed: 2023-
01-11.
Bloom, B., Engelhart, M., Furst, E., W.Hill, and Krath-
wohl, D. (1956). Taxonomy of Educational Objec-
tives, Handbook 1: Cognitive Domain. Longman,
New York; New York; London, 2nd edition edition
edition.
B
¨
orner, K., Maltese, A., Balliet, R. N., and Heimlich, J.
(2016). Investigating aspects of data visualization
literacy using 20 information visualizations and 273
science museum visitors. Information Visualization,
15(3):198–213.
Ceneda, D., Arleo, A., Gschwandtner, T., and Miksch, S.
(2021). Show me your face: towards an automated
method to provide timely guidance in visual analyt-
ics. IEEE Transactions on Visualization and Com-
puter Graphics, 28(12):4570–4581.
Ceneda, D., Gschwandtner, T., May, T., Miksch, S., Schulz,
H.-J., Streit, M., and Tominski, C. (2017). Character-
izing guidance in visual analytics. IEEE Transactions
on Visualization and Computer Graphics, 23:111–
120.
Ceneda, D., Gschwandtner, T., and Miksch, S. (2019a). A
Review of Guidance Approaches in Visual Data Anal-
ysis: A Multifocal Perspective. Computer Graphics
Forum, 38(3):861–879.
Ceneda, D., Gschwandtner, T., and Miksch, S. (2019b). You
get by with a little help: The effects of variable guid-
ance degrees on performance and mental state. Visual
Informatics, 3(4):177–191.
Chundury, P., Yalc¸in, M. A., Crabtree, J., Mahurkar, A.,
Shulman, L. M., and Elmqvist, N. (2023). Contex-
tual in situ help for visual data interfaces. Information
Visualization, 22(1):69–84.
Dhanoa, V., Walchshofer, C., Hinterreiter, A., Stitz, H.,
Groeller, E., and Streit, M. (2022). A process model
for dashboard onboarding. Computer Graphics Forum
(EuroVis ’22), 41(3):501–513.
Firat, E. E., Denisova, A., and Laramee, R. S. (2020).
Treemap Literacy: A Classroom-Based Investigation.
In Romero, M. and Sousa Santos, B., editors, Euro-
graphics 2020 - Education Papers, pages 29–38, n.p.
The Eurographics Association.
Galesic, M. and Garcia-Retamero, R. (2010). Graph Liter-
acy: A Cross-Cultural Comparison. Medical Decision
Making, 31(3):444–457. Publisher: SAGE Publica-
tionsSage CA: Los Angeles, CA.
Grayling, T. (2002). If we build it, will they come? a usabil-
ity test of two browser-based embedded help systems.
Technical communication, 49(2):193–209.
Horvitz, E. (1999). Principles of mixed-initiative user inter-
faces. In Proceedings of the SIGCHI Conference on
Human Factors in Computing Systems, CHI ’99, page
159–166, New York, NY, USA. ACM.
Huron, S., Jansen, Y., and Carpendale, S. (2014). Con-
structing visual representations: Investigating the use
of tangible tokens. IEEE transactions on visualization
and computer graphics, 20(12):2102–2111.
Joshi, A., Srinivas, C., Firat, E. E., and Laramee, R. S.
(2024). Evaluating the recommendations of llms to
teach a visualization technique using bloom’s taxon-
omy. Technical report, University of Nottingham.
Khurana, A., Subramonyam, H., and Chilana, P. K. (2024).
Why and when llm-based assistants can go wrong: In-
vestigating the effectiveness of prompt-based interac-
tions for software help-seeking. In Proceedings of IUI,
IUI ’24, page 288–303, New York, NY, USA. ACM.
Kiani, K., Cui, G., Bunt, A., McGrenere, J., and Chilana,
P. K. (2019). Beyond ”one-size-fits-all”: Understand-
ing the diversity in how software newcomers discover
and make use of help resources. In Proceedings of
the 2019 CHI Conference on Human Factors in Com-
puting Systems, CHI ’19, page 1–14, New York, NY,
USA. Association for Computing Machinery.
Kwon, B. C. and Lee, B. (2016). A comparative evalua-
tion on online learning approaches using parallel co-
ordinate visualization. In Proceedings of the SIGCHI
Conference on Human Factors in Computing Systems,
CHI ’16, pages 993–997, New York, NY, USA. ACM
Press.
Lee, S., Kim, S.-H., Hung, Y.-H., Lam, H., Kang, Y.-A., and
Yi, J. S. (2016). How do people make sense of unfa-
miliar visualizations?: A grounded model of novice’s
information visualization sensemaking. IEEE Trans-
actions on Visualization and Computer Graphics,
22(1):499–508.
Lee, S., Kim, S.-H., and Kwon, B. C. (2017). VLAT:
Development of a visualization literacy assessment
test. IEEE Transactions on Visualization and Com-
puter Graphics, 23(1):551–560.
Mahmud, S., Alvina, J., Chilana, P. K., Bunt, A., and Mc-
Grenere, J. (2020). Learning through exploration:
How children, adults, and older adults interact with
a new feature-rich application. In Proceedings of the
2020 CHI Conference on Human Factors in Comput-
ing Systems, CHI ’20, page 1–14, New York, NY,
USA. Association for Computing Machinery.
Pazzani, M. J. and Billsus, D. (2007). Content-Based
Recommendation Systems, pages 325–341. Springer
Berlin Heidelberg, Berlin, Heidelberg.
Peng, I., Firat, E. E., Laramee, R. S., and Joshi, A. (2022).
Evaluating Bloom’s Taxonomy-based Learning Mod-
ules for Parallel Coordinates Literacy. In Bourdin, J.-
J. and Paquette, E., editors, Eurographics 2022 - Edu-
cation Papers, pages 21–29, Eindhoven, The Nether-
lands. The Eurographics Association.
Pohl, M., Potzmann, B., Stoiber, C., and Aigner, W.
(2023). Visualization Onboarding Designed by Users:
an Empirical Study. In International Conference on
Cognition and Exploratory Learning in Digital Age
(CELDA), pages 3–10, Funchal, Madeira, Portugal.
IADIS Press.
Potzmann, B., Pohl, M., Moitzi, D., and Girardi, D. (2023).
Using design actions for a heuristic evaluation of hid-
den. Technical report, Technische Universit
¨
at Wien.
P
´
erez-Messina, I., Ceneda, D., El-Assady, M., Miksch, S.,
and Sperrle, F. (2022). A Typology of Guidance
Tasks in Mixed-Initiative Visual Analytics Environ-
ments. Computer Graphics Forum, 41(3):465–476.
IVAPP 2025 - 16th International Conference on Information Visualization Theory and Applications
716