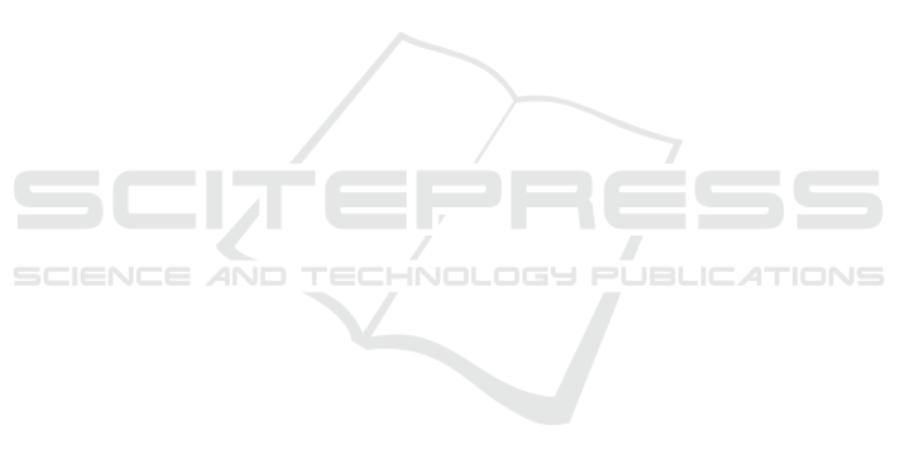
rights of persons with disabilities: background, re-
quirements and (remaining) questions. Netherlands
Quarterly of Human Rights, 32(3):263–287.
Fichten, C. S., Ferraro, V., Asuncion, J. V., Chwojka, C.,
Barile, M., Nguyen, M. N., Klomp, R., and Wolforth,
J. (2009). Disabilities and e-learning problems and so-
lutions: An exploratory study. Journal of Educational
Technology & Society, 12(4):241–256.
Firat, T. (2021). Experiences of students with visual impair-
ments in higher education: barriers and facilitators.
British Journal of Special Education, 48(3):301–322.
Fouh, E., Akbar, M., and Shaffer, C. A. (2012). The role of
visualization in computer science education. Comput-
ers in the Schools, 29(1-2):95–117.
Giese, M., Greisbach, M., Meier, M., Neusser, T., and
Wetekam, N. (2022). ‘I usually never got involved’:
understanding reasons for secondary students with
visual impairments leaving mainstream schooling in
Germany. European Journal of Special Needs Educa-
tion, 37(2):264–277.
Glazko, K. S., Yamagami, M., Desai, A., Mack, K. A.,
Potluri, V., Xu, X., and Mankoff, J. (2023). An Au-
toethnographic Case Study of Generative Artificial In-
telligence’s Utility for Accessibility. In Proceedings of
the 25th International ACM SIGACCESS Conference
on Computers and Accessibility, pages 1–8.
He, X. and Deng, L. (2017). Deep learning for image-to-
text generation: A technical overview. IEEE Signal
Processing Magazine, 34(6):109–116.
Heyer, K. (2021). What is a human right to inclu-
sive education? The promises and limitations of the
crpd’s inclusion mandate. Handbuch Inklusion inter-
national/International Handbook of Inclusive Educa-
tion, pages 45–58.
Hutchinson, J. S. O., Atkinson, K., and Orpwood, J. (1998).
Breaking down barriers: Access to further and higher
education for visually impaired students. Nelson
Thornes.
Ishihara, T., Takagi, H., Itoh, T., and Asakawa, C. (2006).
Analyzing visual layout for a non-visual presentation-
document interface. In Proceedings of the 8th inter-
national ACM SIGACCESS conference on Computers
and accessibility, pages 165–172.
Jenkinson, J. (2018). Molecular biology meets the learning
sciences: Visualizations in education and outreach.
Journal of Molecular Biology, 430(21):4013–4027.
Johnson, O., Alyasiri, O., Akhtom, D., and Johnson, O.
(2023). Image analysis through the lens of chatgpt-
4. Journal of Applied Artificial Intelligence, 4(2).
Jones, L. L., Jordan, K. D., and Stillings, N. A. (2005).
Molecular visualization in chemistry education: the
role of multidisciplinary collaboration. Chemistry Ed-
ucation Research and Practice, 6(3):136–149.
Jones, M. G., Minogue, J., Oppewal, T., Cook, M. P., and
Broadwell, B. (2006). Visualizing without vision at
the microscale: Students with visual impairments ex-
plore cells with touch. Journal of science education
and technology, 15(5):345–351.
Jung, C., Mehta, S., Kulkarni, A., Zhao, Y., and Kim, Y.-S.
(2021). Communicating visualizations without visu-
als: Investigation of visualization alternative text for
people with visual impairments. IEEE transactions
on visualization and computer graphics, 28(1):1095–
1105.
Kim, N. W., Joyner, S. C., Riegelhuth, A., and Kim, Y.
(2021). Accessible Visualization: Design Space, Op-
portunities, and Challenges. Computer Graphics Fo-
rum, 40(3):173–188.
Leiker, D., Gyllen, A. R., Eldesouky, I., and Cukurova, M.
(2023). Generative AI for Learning: Investigating the
Potential of Learning Videos with Synthetic Virtual
Instructors. In International conference on artificial
intelligence in education, pages 523–529. Springer.
Lundgard, A. and Satyanarayan, A. (2021). Accessible vi-
sualization via natural language descriptions: A four-
level model of semantic content. IEEE transactions
on visualization and computer graphics, 28(1):1073–
1083.
MacKay, D. (2006). The United Nations Convention on the
rights of persons with disabilities. Syracuse J. Int’l L.
& Com., 34:323.
Mohamady, A. A., Fathy, A. G., Ibrahim, A. E., Darwish,
M. S., and Salama, M. K. (2023). Enhancing Acces-
sibility and Independence of Visually Impaired Indi-
viduals through AI, ML and IoT: The Development
of a Smart Robot Assistant. Enhancing Accessibil-
ity and Independence of Visually Impaired Individuals
through AI, ML and IoT: The Development of a Smart
Robot Assistant.— IUSRJ, 4.
Moured, O., Alzalabny, S., Schwarz, T., Rapp, B., and
Stiefelhagen, R. (2023). Accessible Document Lay-
out: An Interface for 2D Tactile Displays. In Proceed-
ings of the 16th International Conference on PErva-
sive Technologies Related to Assistive Environments,
pages 265–271.
Naps, T. L., R
¨
oßling, G., Almstrum, V., Dann, W., Fleis-
cher, R., Hundhausen, C., Korhonen, A., Malmi, L.,
McNally, M., Rodger, S., et al. (2002). Exploring the
role of visualization and engagement in computer sci-
ence education. In Working group reports from ITiCSE
on Innovation and technology in computer science ed-
ucation, pages 131–152. Association for Computing
Machinery.
Open AI (n. d.). Text generation models. Online; accessed
08.01.2025.
Permvattana, R., Armstrong, H., and Murray, I. (2013). E-
learning for the vision impaired: A holistic perspec-
tive. International Journal of Cyber Society and Edu-
cation, 6(1):15–30.
Rapp, D. N. (2005). Mental models: Theoretical issues for
visualizations in science education. Visualization in
science education, pages 43–60.
Sharif, A., Chintalapati, S. S., Wobbrock, J. O., and Rei-
necke, K. (2021). Understanding screen-reader users’
experiences with online data visualizations. In Pro-
ceedings of the 23rd International ACM SIGACCESS
Conference on Computers and Accessibility, pages 1–
16.
Sheela, S., Krishnan, R., Srivathsan, R., Sudharsan, M., and
Sriram, S. (2023). Enhancing accessibility: object
CSEDU 2025 - 17th International Conference on Computer Supported Education
24