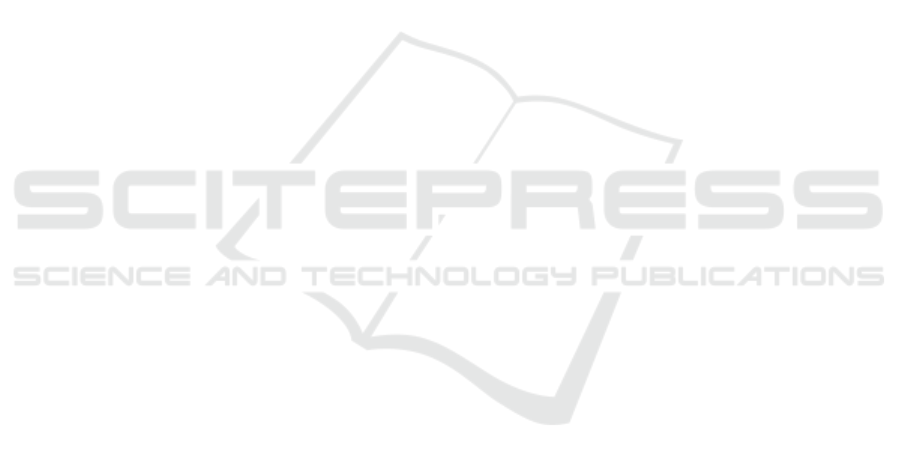
temporal graphs. In IEEE/CVF Conf. on Comp. Vision
and Pattern Recognition, pages 5308–5317.
Jang, D.-K., Park, S., and Lee, S.-H. (2022). Motion puzzle:
Arbitrary motion style transfer by body part. ACM
Trans. on Graph., 41(3).
Jiang, J., Streli, P., Qiu, H., Fender, A., Laich, L., Snape,
P., and Holz, C. (2022). Avatarposer: Articulated full-
body pose tracking from sparse motion sensing. In
Comp. Vision – ECCV 2022, pages 443–460. Springer
Nature.
Ko, H. and Badler, N. (1996). Animating human locomo-
tion with inverse dynamics. IEEE Comp. Graph. and
Applications, 16(2):50–59.
Kovar, L., Gleicher, M., and Pighin, F. (2002). Motion
graphs. ACM Trans. on Graph., 21(3):473–482.
Lee, S., Kang, T., Park, J., Lee, J., and Won, J. (2023a).
Same: Skeleton-agnostic motion embedding for char-
acter animation. In ACM SIGGRAPH Asia Conf. Proc.
Lee, S., Lee, J., and Lee, J. (2022). Learning virtual
chimeras by dynamic motion reassembly. ACM Trans.
on Graph., 41(6):1–13.
Lee, S., Starke, S., Ye, Y., Won, J., and Winkler, A. (2023b).
Questenvsim: Environment-aware simulated motion
tracking from sparse sensors. In ACM SIGGRAPH
Conf. Proc.
Li, M., Chen, S., Chen, X., Zhang, Y., Wang, Y., and Tian,
Q. (2022a). Symbiotic graph neural networks for 3d
skeleton-based human action recognition and motion
prediction. IEEE Trans. on Pattern Analysis and Ma-
chine Intelligence, 44(6).
Li, M., Chen, S., Zhang, Z., Xie, L., Tian, Q., and Zhang,
Y. (2022b). Skeleton-parted graph scattering networks
for 3d human motion prediction. In Comp. Vision –
ECCV 2022, page 18–36.
Li, Y., Wang, Z., Yang, X., Wang, M., Poiana, S. I.,
Chaudhry, E., and Zhang, J. (2019). Efficient con-
volutional hierarchical autoencoder for human motion
prediction. The Visual Computer, 35(6):1143–1156.
Liu, Z., Lyu, K., Wu, S., Chen, H., Hao, Y., and Ji, S.
(2021). Aggregated multi-gans for controlled 3d hu-
man motion prediction. AAAI Conf. on Artificial In-
telligence Proc., 35(3):2225–2232.
Malek-Podjaski, M. and Deligianni, F. (2023). Adversarial
attention for human motion synthesis. In IEEE Sym-
posium Series on Computational Intelligence, pages
69–74.
Mao, W., Liu, M., and Salzmann, M. (2020). History re-
peats itself: Human motion prediction via motion at-
tention. In Comp. Vision – ECCV 2020, pages 474–
489.
Mourot, L., Hoyet, L., Le Clerc, F., Schnitzler, F., and
Hellier, P. (2022). A survey on deep learning for
skeleton-based human animation. Comp. Graph. Fo-
rum, 41:122–157.
Reda, D., Won, J., Ye, Y., van de Panne, M., and Winkler,
A. W. (2023). Physics-based motion retargeting from
sparse inputs. Proc. ACM Comput. Graph. Interact.
Tech., 6.
Shao, Z., Li, Y., Guo, Y., Zhou, X., and Chen, S. (2019). A
hierarchical model for human action recognition from
body-parts. IEEE Trans. on Circuits and Systems for
Video Technology, 29(10):2986–3000.
Shu, X., Zhang, L., Qi, G.-J., Liu, W., and Tang, J. (2022).
Spatiotemporal co-attention recurrent neural networks
for human-skeleton motion prediction. IEEE Trans.
on Pattern Analysis and Machine Intelligence, 44(6).
Starke, S., Mason, I., and Komura, T. (2022). Deepphase:
periodic autoencoders for learning motion phase man-
ifolds. ACM Trans. on Graph., 41(4):1–13.
Wang, W., Zhou, T., Qi, S., Shen, J., and Zhu, S.-C. (2022).
Hierarchical human semantic parsing with compre-
hensive part-relation modeling. IEEE Trans. on Pat-
tern Analysis and Machine Intelligence, 44(7):3508–
3522.
Wang, Y. and Neff, M. (2015). Deep signatures for index-
ing and retrieval in large motion databases. In ACM
SIGGRAPH Conf. on Motion in Games, page 37–45.
Yan, X., Rastogi, A., Villegas, R., Sunkavalli, K., Shecht-
man, E., Hadap, S., Yumer, E., and Lee, H. (2018).
Mt-vae: Learning motion transformations to gener-
ate multimodal human dynamics. In Comp. Vision –
ECCV 2018.
Yang, D., Kim, D., and Lee, S.-H. (2021). Lobstr:
Real-time lower-body pose prediction from sparse
upper-body tracking signals. Comp. Graph. Forum,
40(2):265–275.
Ye, Y., Liu, L., Hu, L., and Xia, S. (2022). Neural3points:
Learning to generate physically realistic full-body
motion for virtual reality users. Comp. Graph. Forum,
41(8):183–194.
Zhang, H., Starke, S., Komura, T., and Saito, J. (2018).
Mode-adaptive neural networks for quadruped motion
control. ACM Trans. on Graph., 37(4):1–11.
Zhang, J., Tu, Z., Weng, J., Yuan, J., and Du, B. (2024). A
modular neural motion retargeting system decoupling
skeleton and shape perception. IEEE Trans. on Pattern
Analysis and Machine Intelligence.
Zhou, L., Shang, L., Shum, H. P., and Leung, H. (2014).
Human motion variation synthesis with multivariate
gaussian processes. Comp. Animation and Virtual
Worlds, 25(3-4):301–309.
Zhou, Y., Barnes, C., Lu, J., Yang, J., and Li, H. (2019). On
the Continuity of Rotation Representations in Neural
Networks. In IEEE/CVF Conf. on Comp. Vision and
Pattern Recognition, pages 5738–5746.
Zou, Q., Yuan, S., Du, S., Wang, Y., Liu, C., Xu, Y., Chen,
J., and Ji, X. (2025). Parco: Part-coordinating text-to-
motion synthesis. In Comp. Vision – ECCV 2024.
GRAPP 2025 - 20th International Conference on Computer Graphics Theory and Applications
64