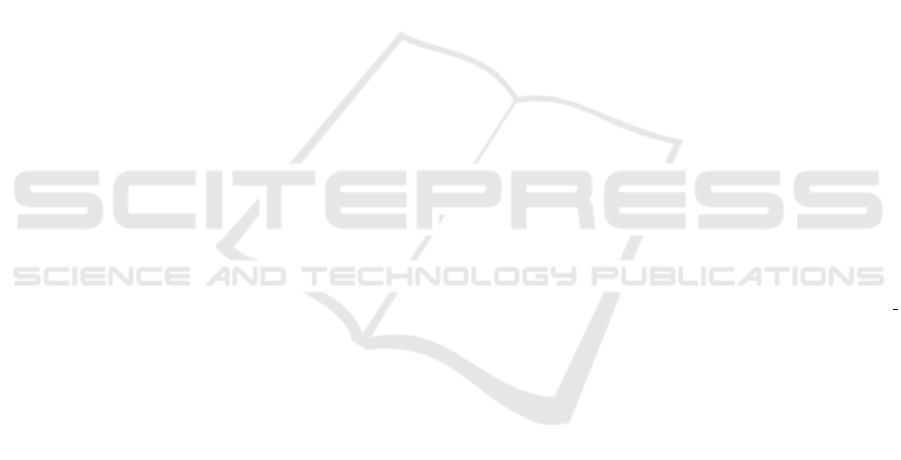
Fouladgar, M., Parchami, M., Elmasri, R., and Ghaderi, A.
(2017). Scalable deep traffic flow neural networks for
urban traffic congestion prediction. In 2017 Interna-
tional Joint Conference on Neural Networks (IJCNN),
pages 2251–2258. IEEE.
Fu, R., Zhang, Z., and Li, L. (2016). Using lstm and gru
neural network methods for traffic flow prediction.
In 2016 31st Youth academic annual conference of
Chinese association of automation (YAC), pages 324–
328. IEEE.
Garber, N. J. and Hoel, L. A. (2019). Traffic and highway
engineering. Cengage Learning.
Gonz
´
alez-Aliste, P., Derpich, I., and L
´
opez, M. (2023).
Reducing urban traffic congestion via charging price.
Sustainability, 15(3):2086.
Harrou, F., Zeroual, A., and Sun, Y. (2020). Traffic conges-
tion monitoring using an improved knn strategy. Mea-
surement, 156:107534.
Hasanzadeh, A., Liu, X., Duffield, N., Narayanan, K. R.,
and Chigoy, B. (2017). A graph signal processing ap-
proach for real-time traffic prediction in transportation
networks. arXiv preprint arXiv:1711.06954.
He, Y., Hofer, B., Sheng, Y., Yin, Y., and Lin, H. (2023).
Processes and events in the center: a taxi trajectory-
based approach to detecting traffic congestion and an-
alyzing its causes. International Journal of Digital
Earth, 16(1):509–531.
Hearst, M. A., Dumais, S. T., Osuna, E., Platt, J., and
Scholkopf, B. (1998). Support vector machines. IEEE
Intelligent Systems and their applications, 13(4):18–
28.
Hochreiter, S. and Schmidhuber, J. (1997). Long short-term
memory. Neural computation, 9(8):1735–1780.
Ioannou, C. and Vassiliou, V. (2021). Network attack classi-
fication in iot using support vector machines. Journal
of sensor and actuator networks, 10(3):58.
Kalair, K. and Connaughton, C. (2021). Anomaly detection
and classification in traffic flow data from fluctuations
in the flow–density relationship. Transportation Re-
search Part C: Emerging Technologies, 127:103178.
Kohan, M. and Ale, J. M. (2020). Discovering traffic
congestion through traffic flow patterns generated by
moving object trajectories. Computers, Environment
and Urban Systems, 80:101426.
Kong, X., Song, X., Xia, F., Guo, H., Wang, J., and Tolba,
A. (2018). Lotad: Long-term traffic anomaly detec-
tion based on crowdsourced bus trajectory data. World
Wide Web, 21(3):825–847.
Kuang, W., An, S., and Jiang, H. (2015). Detecting traffic
anomalies in urban areas using taxi gps data. Mathe-
matical Problems in Engineering, 2015.
Lam, P., Wang, L., Ngan, H. Y., Yung, N. H., and Yeh, A. G.
(2017). Outlier detection in large-scale traffic data
by na
¨
ıve bayes method and gaussian mixture model
method. Electronic Imaging, 2017(9):73–78.
Lee, K., Eo, M., Jung, E., Yoon, Y., and Rhee, W. (2021).
Short-term traffic prediction with deep neural net-
works: A survey. IEEE Access, 9:54739–54756.
Li, L., Lin, Y., Du, B., Yang, F., and Ran, B. (2020). Real-
time traffic incident detection based on a hybrid deep
learning model. Transportmetrica A: Transport Sci-
ence, pages 1–21.
Li, L., Lin, Y., Du, B., Yang, F., and Ran, B. (2022). Real-
time traffic incident detection based on a hybrid deep
learning model. Transportmetrica A: transport sci-
ence, 18(1):78–98.
Li, Y., Yu, R., Shahabi, C., and Liu, Y. (2017). Diffusion
convolutional recurrent neural network: Data-driven
traffic forecasting. arXiv preprint arXiv:1707.01926.
Liu, D., Zhen, H., Kong, D., Chen, X., Zhang, L., Yuan,
M., and Wang, H. (2021). Sensors anomaly detection
of industrial internet of things based on isolated forest
algorithm and data compression. Scientific Program-
ming, 2021:1–9.
Liu, F. T., Ting, K. M., and Zhou, Z.-H. (2008). Isolation
forest. In 2008 eighth ieee international conference
on data mining, pages 413–422. IEEE.
Liu, Y., Cai, Z., and Dou, H. (2023). Highway traffic
congestion detection and evaluation based on deep
learning techniques. Soft Computing, 27(17):12249–
12265.
Makara, L. A., Maric, P., and Pekar, A. (2023). Public trans-
port congestion detection using incremental learning.
Pervasive and Mobile Computing, 91:101769.
Markovic, T., Dehlaghi-Ghadim, A., Leon, M., Balador, A.,
and Punnekkat, S. (2023). Time-series anomaly de-
tection and classification with long short-term mem-
ory network on industrial manufacturing systems. In
2023 18th Conference on Computer Science and In-
telligence Systems (FedCSIS), pages 171–181. IEEE.
Nagy, A. M. and Simon, V. (2018). Survey on traffic predic-
tion in smart cities. Pervasive and Mobile Computing,
50:148–163.
Nagy, A. M. and Simon, V. (2020). Traffic incident
dataset. https://gitlab.medianets.hu/anagy/incident
dataset. Accessed: 2024-08-30.
Nagy, A. M. and Simon, V. (2021). A novel congestion
propagation modeling algorithm for smart cities. Per-
vasive and Mobile Computing, 73:101387.
Nagy, A. M., Wiandt, B., and Simon, V. (2021). Transient-
based automatic incident detection method for intelli-
gent transport systems. Infocommunications Journal,
13(3):2–13.
Nassif, A. B., Talib, M. A., Nasir, Q., and Dakalbab, F. M.
(2021). Machine learning for anomaly detection: A
systematic review. Ieee Access, 9:78658–78700.
Nguyen, H., Liu, W., and Chen, F. (2016). Discovering con-
gestion propagation patterns in spatio-temporal traffic
data. IEEE Transactions on Big Data, 3(2):169–180.
Nguyen, T.-H. and Jung, J. J. (2021). Swarm intelligence-
based green optimization framework for sustain-
able transportation. Sustainable Cities and Society,
71:102947.
Pan, B., Zheng, Y., Wilkie, D., and Shahabi, C. (2013).
Crowd sensing of traffic anomalies based on human
mobility and social media. In Proceedings of the
21st ACM SIGSPATIAL International Conference on
Advances in Geographic Information Systems, pages
344–353. ACM.
Machine Learning-Based Anomaly Detection in Smart City Traffic: Performance Comparison and Insights
317