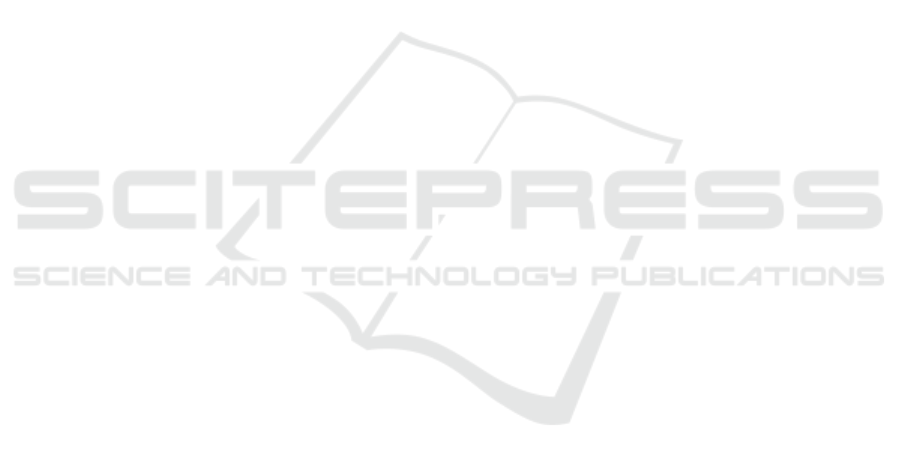
real local oscillator using simultaneous pilot signals.
Optics Letters, 42(8):1588.
Laudenbach, F., Pacher, C., Fung, C.-H. F., Poppe, A.,
Peev, M., Schrenk, B., Hentschel, M., Walther, P., and
H
¨
ubel, H. (2018). Continuous-Variable Quantum Key
Distribution with Gaussian Modulation-The Theory of
Practical Implementations. Advanced Quantum Tech-
nologies, 1(1):1800011.
Leverrier, A., All
´
eaume, R., Boutros, J., Z
´
emor, G., and
Grangier, P. (2008). Multidimensional reconciliation
for a continuous-variable quantum key distribution.
Physical Review A, 77(4):042325.
Leverrier, A. and Grangier, P. (2011). Continuous-
variable quantum key distribution protocols with
a non-Gaussian modulation. Physical Review A,
83(4):042312.
Leverrier, A., Grosshans, F., and Grangier, P. (2010). Finite-
size analysis of a continuous-variable quantum key
distribution. Physical Review A, 81(6):062343.
Li, Q., Wen, X., Mao, H., and Wen, X. (2019). An im-
proved multidimensional reconciliation algorithm for
continuous-variable quantum key distribution. Quan-
tum Information Processing, 18(1):25.
Lin, J. and L
¨
utkenhaus, N. (2020). Trusted detector
noise analysis for discrete modulation schemes of
continuous-variable quantum key distribution. Physi-
cal Review Applied, 14(6):064030.
Liu, W.-B., Li, C.-L., Xie, Y.-M., Weng, C.-X., Gu, J., Cao,
X.-Y., Lu, Y.-S., Li, B.-H., Yin, H.-L., and Chen, Z.-B.
(2021). Homodyne Detection Quadrature Phase Shift
Keying Continuous-Variable Quantum Key Distribu-
tion with High Excess Noise Tolerance. PRX Quan-
tum, 2(4):040334.
Pirandola, S., Andersen, U. L., Banchi, L., Berta, M.,
Bunandar, D., Colbeck, R., Englund, D., Gehring, T.,
Lupo, C., Ottaviani, C., Pereira, J. L., Razavi, M.,
Shamsul Shaari, J., Tomamichel, M., Usenko, V. C.,
Vallone, G., Villoresi, P., and Wallden, P. (2020). Ad-
vances in quantum cryptography. Advances in Optics
and Photonics, 12(4):1012.
Roumestan, F., Ghazisaeidi, A., Renaudier, J., Brindel, P.,
Diamanti, E., and Grangier, P. (2021a). Demonstra-
tion of probabilistic constellation shaping for contin-
uous variable quantum key distribution. In Optical
Fiber Communication Conference (OFC) 2021, page
F4E.1, Washington, DC. Optica Publishing Group.
Roumestan, F., Ghazisaeidi, A., Renaudier, J., Vidarte,
L. T., Diamanti, E., and Grangier, P. (2021b). High-
rate continuous variable quantum key distribution
based on probabilistically shaped 64 and 256-QAM.
In 2021 European Conference on Optical Communi-
cation (ECOC), pages 1–4, Bordeaux, France. IEEE.
Van Assche, G., Cardinal, J., and Cerf, N. (2004). Reconcil-
iation of a Quantum-Distributed Gaussian Key. IEEE
Transactions on Information Theory, 50(2):394–400.
Wang, X., Wang, H., Zhou, C., Chen, Z., Yu, S., and Guo,
H. (2022). Continuous-variable quantum key distribu-
tion with low-complexity information reconciliation.
Optics Express, 30(17):30455.
Wen, X., Li, Q., Mao, H., Wen, X., and Chen, N.
(2021). An Improved Slice Reconciliation Protocol
for Continuous-Variable Quantum Key Distribution.
Entropy, 23(10):1317.
Yang, S., Yan, Z., Yang, H., Lu, Q., Lu, Z., Cheng, L., Miao,
X., and Li, Y. (2023). Information reconciliation of
continuous-variables quantum key distribution: Prin-
ciples, implementations and applications. EPJ Quan-
tum Technology, 10(1):40.
Zhang, Y., Li, Z., Chen, Z., Weedbrook, C., Zhao, Y.,
Wang, X., Huang, Y., Xu, C., Zhang, X., Wang, Z., Li,
M., Zhang, X., Zheng, Z., Chu, B., Gao, X., Meng,
N., Cai, W., Wang, Z., Wang, G., Yu, S., and Guo,
H. (2019). Continuous-variable QKD over 50 km
commercial fiber. Quantum Science and Technology,
4(3):035006.
PHOTOPTICS 2025 - 13th International Conference on Photonics, Optics and Laser Technology
86