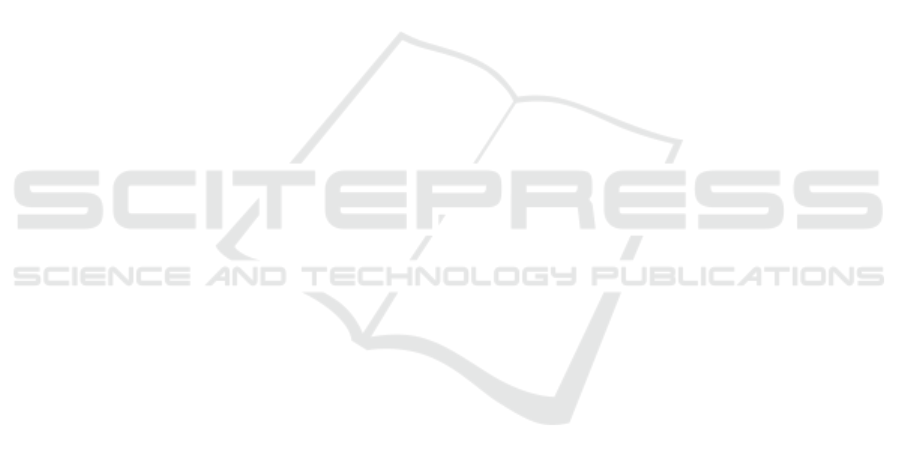
business applications: A systematic literature review.
In Business Information Systems: 21st International
Conference, BIS 2018, Berlin, Germany, July 18-20,
2018, Proceedings 21, pages 384–399. Springer.
Kumar, N., Singh, M., Upreti, K., and Mohan, D. (2022).
Blockchain adoption intention in higher education:
Role of trust, perceived security and privacy in tech-
nology adoption model. In Lecture notes in networks
and systems, volume C1. Springer.
Liu, N. and Ye, Z. (2021). Empirical research on the
blockchain adoption – based on tam. Applied Eco-
nomics, 53(37):4263–4275.
Maranguni
´
c, N. and Grani
´
c, A. (2015). Technology accep-
tance model: a literature review from 1986 to 2013.
Universal access in the information society, 14:81–
95.
Marengo, A. and Pagano, A. (2023). Investigating the fac-
tors influencing the adoption of blockchain technol-
ogy across different countries and industries: a sys-
tematic literature review. Electronics, 12(14):3006.
Mayer, R. C., Davis, J. H., and Schoorman, F. D. (1995). An
integrative model of organizational trust. Academy of
management review, 20(3):709–734.
Mcknight, D. H., Carter, M., Thatcher, J. B., and Clay, P. F.
(2011). Trust in a specific technology: An investi-
gation of its components and measures. ACM Trans-
actions on management information systems (TMIS),
2(2):1–25.
Meeßen, S. M., Thielsch, M. T., and Hertel, G. (2019). Trust
in management information systems (mis). Zeitschrift
f
¨
ur Arbeits-und Organisationspsychologie A&O.
Mohammadi, N. G. (2019). Trustworthy cyber-physical sys-
tems: A systematic framework towards design and
evaluation of trust and trustworthiness. Springer
Vieweg, Wiesbaden, Germany, 1 edition.
Murtin, F., Fleischer, L., Siegerink, V., Aassve, A., Algan,
Y., Boarini, R., Gonz
´
alez, S., Lonti, Z., Grimalda, G.,
Vallve, R. H., et al. (2018). Trust and its determinants:
Evidence from the trustlab experiment.
Norbu, T., Park, J. Y., Wong, K. W., and Cui, H. (2024).
Factors affecting trust and acceptance for blockchain
adoption in digital payment systems: A systematic re-
view. Future Internet, 16(3):106.
Palos-Sanchez, P. R., Saura, J., and Ayestaran, R. (2021).
An exploratory approach to the adoption process
of bitcoin by business executives. Mathematics,
9(4):355.
Pietrzak, P. and Takala, J. (2021). Digital trust - a systematic
literature review.
Queiroz, M. M., Wamba, S. F., De Bourmont, M., and
Telles, R. (2021). Blockchain adoption in operations
and supply chain management: empirical evidence
from an emerging economy. International Journal of
Production Research, 59(20):6087–6103.
Rambert, P. and Rychkova, I. (2024). Implications of trust
in cyber-physical systems design: the assa case study.
In Enterprise Design, Operations, and Computing –
28th International Conference, EDOC 2024, Vienna,
Austria, September 10 - 13, 2024, volume 15409.
Springer.
Rijanto, A. (2021). Blockchain technology adoption in sup-
ply chain finance. Journal of Theoretical and Applied
Electronic Commerce Research, 16(7):3078–3098.
Rousseau, D. M., Sitkin, S. B., Burt, R. S., and Camerer,
C. (1998). Not so different after all: A cross-
discipline view of trust. Academy of management re-
view, 23(3):393–404.
Rychkova, I. and Ghriba, M. (2023). Trustworthiness re-
quirements in information systems design: Lessons
learned from the blockchain community. Com-
plex Systems Informatics and Modeling Quarterly,
(35):67–91.
Saputra, U. R. and Darma, G. S. (2022). The intention to
use blockchain in indonesia using extended approach
technology acceptance model (tam). Commit Journal,
16(1):27–35.
Sciarelli, M., Prisco, A., Gheith, M., and Muto, V. (2021).
Factors affecting the adoption of blockchain technol-
ogy in innovative italian companies: an extended tam
approach. Journal of Strategy and Management.
Shin, D.-H. (2019). Blockchain: The emerging technol-
ogy of trust the role of trust in the acceptance of
blockchain. Telematics and Informatics, page 101278.
Shrestha, A. K., Vassileva, J., Joshi, S., and Just, J. (2021).
Augmenting the technology acceptance model with
trust model for the initial adoption of a blockchain-
based system. PeerJ. Computer science, 7:e502.
S
¨
ollner, M., Hoffmann, A., Hoffmann, H., Wacker, A., and
Leimeister, J. M. (2012). Understanding the formation
of trust in it artifacts.
Taherdoost, H. (2022). A critical review of blockchain
acceptance models - blockchain technology adoption
frameworks and applications. Comput., 11:24.
Taufiq, R., Hidayanto, A. N., Prabowo, H., et al. (2018).
The affecting factors of blockchain technology adop-
tion of payments systems in indonesia banking indus-
try. In 2018 International Conference on Information
Management and Technology (ICIMTech), pages 506–
510. IEEE.
Ullah, N., Al-rahmi, W. M., and Alkhalifah, A. (2021). Pre-
dictors for distributed ledger technology adoption: in-
tegrating three traditional adoption theories for manu-
facturing and service operations. Production & Man-
ufacturing Research, 9(1):178–205.
Venkatesh, V. and Davis, F. D. (2000). A theoretical exten-
sion of the technology acceptance model: Four longi-
tudinal field studies. Management science, 46(2):186–
204.
Venkatesh, V., Morris, M. G., Davis, G. B., and Davis, F. D.
(2003). User acceptance of information technology:
Toward a unified view. MIS quarterly, pages 425–478.
Wang, X., Mylopoulos, J., Guizzardi, G., and Guarino, N.
(2016). How software changes the world: The role of
assumptions. In 2016 IEEE Tenth International Con-
ference on Research Challenges in Information Sci-
ence (RCIS), pages 1–12. IEEE.
Yu, C., Prabhu, M., Goli, M., and Sahu, A. K. (2021). Fac-
tors affecting the adoption of blockchain technology
in the complex industrial systems: Data modeling.
Complexity, 2021:1–10.
ICEIS 2025 - 27th International Conference on Enterprise Information Systems
26