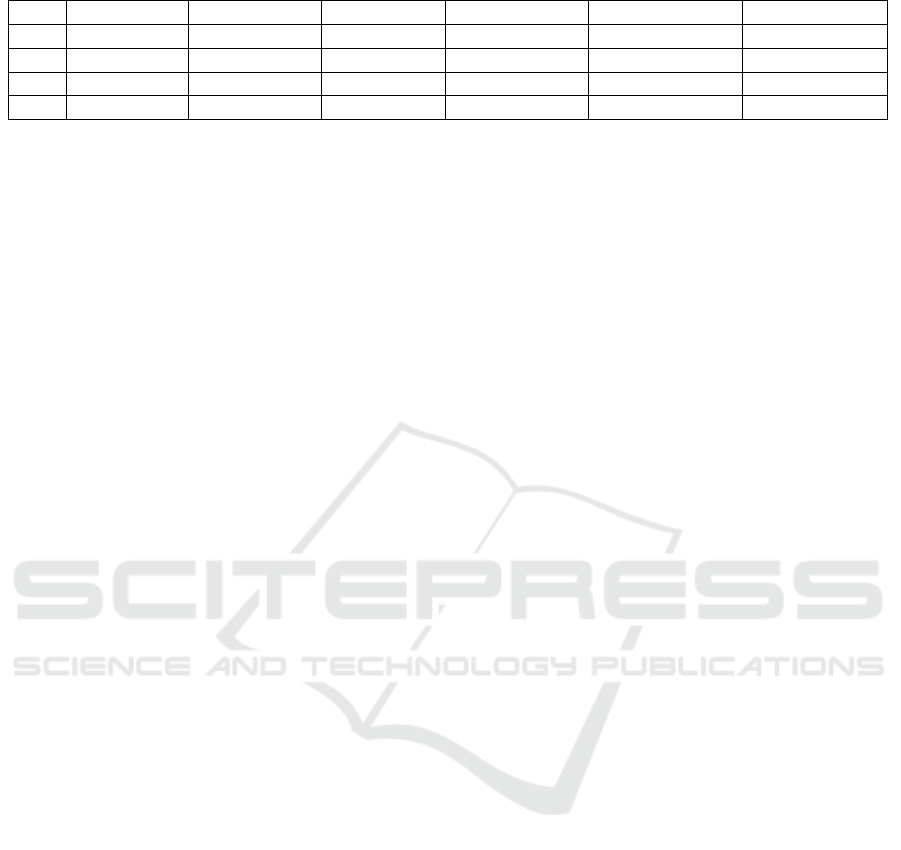
Table 1: The calculated inertial activity characteristics.
Flexion Time Flexion Range Flexion Slope Extension Time Extension Range Extension Slope
Mean 0.417 4.966 0.121 0.382 4.959 0.133
Std 0.051 0.267 0.015 0.064 0.381 0.021
Max 0.510 5.345 0.138 0.540 5.517 0.172
Min 0.360 4.543 0.091 0.320 4.261 0.099
the relative rotation. Finally, from this relative rota-
tion matrix, relative angle values in x, y and z direc-
tions are obtained through Euler transformation.
The relative angles x, y and z represent the rela-
tive roll, pitch and yaw signals respectively. For an-
gle tracking and the extraction of relevant features,
relative pitch graph was used in accordance with the
mechanical orientations. On the relative pitch graph,
several features were calculated. First, the peak am-
plitudes, durations and slopes were extracted for each
flexion or extension movement. Then, the mean, stan-
dard deviation, minimum, and maximum values of
the peak, duration, and slope of all cycles were cal-
culated to evaluate the irregularity of the exercises, as
well as the maximum and minimum angles reached. It
should be noted that the features were extracted from
the non-normalized signal. This would potentially en-
able the assessment of the maximum/minimum angle
differences between the participants in future studies.
2.3.4 Acoustical Emissions Analysis
The .bin files saved on the SD card were first con-
verted to the appropriate format using Matlab. It
should be noted that the following pre-processing and
feature extraction steps were repeated for both the
left and right microphones. First, hardware noise was
suppressed using narrow band-stop filters ([185, 205]
and [940, 1050] Hz). The remaining pre-processing
and feature extraction steps were then employed in
Python (version 3.11).
In order to extract the clinically relevant part of the
acoustic emission data from the microphones, a finite
impulse response (FIR) Kaiser window bandpass fil-
ter between 250 Hz and 5 kHz was used. An example
signal is presented in Figure 5. In order to visualize
that the system successfully captures the felt clicks, a
signal containing clicks was specifically selected.
The signal was then divided into 500 ms windows
in parallel with the studies in the literature and 250 ms
overlap was used between the subsequent windows
(50% overlap). For the two different window types
(with and without clicks), we reported the distinctive
differences in the features selected from 3 different
groups: temporal (peak-to-peak), statistical (kurtosis)
and spectral (roll-off).
3 RESULTS AND DISCUSSION
3.1 Inertial Activity and Acoustical
Emissions Results
As mentioned in Section 2.2, the participant per-
formed 10 flexion-extension exercises during the pilot
data acquisition. Figure 5 clearly shows the angular
change. The dots on the graph represent the moments
of flexion, while the peaks represent the moments of
maximum extension. Although the preliminary analy-
sis focused on angular features, direct analysis of raw
accelerometer and gyroscope data would also be pos-
sible in the long term due to their high signal qual-
ity. In addition, the peak amplitudes, durations and
slopes were extracted for each flexion or extension
movement. Then, the mean, standard deviation, mini-
mum, and maximum values of the peak, duration, and
slope of all cycles were calculated to evaluate the ir-
regularity of the exercises, as well as the maximum
and minimum angles reached. The derived values are
presented in Table 1.
Figure 6 shows a superimposition of 500 ms
(10000 samples)-long segments, and examples of a
silent window and a window with clicks. As ex-
plained in Section 2.3.4, three different features were
extracted from a sample silent window and a win-
dow with clicks. As seen, the peak-to-peak values
were 0.081 and 0.023; whereas the kurtosis values
were 26.863 and 0.628, respectively. As higher kur-
tosis refers to the presence of more extreme values or
outliers (i.e. tailedness) compared to a normal dis-
tribution, it was indeed aligning with the morphol-
ogy differences between a window with click and a
silent window. Similarly, the spectral roll-off val-
ues were calculated to be 0.988 and 0.628, respec-
tively. Higher spectral roll-off in signal analysis indi-
cates that a greater proportion of the signal’s energy
is concentrated in the higher frequency components.
The joint clicks are characterized by high energy and
short duration, typically lasting between 10 and 20
milliseconds. Furthermore, these emissions contain
high-frequency components, with bandwidths extend-
ing up to 20 kHz, a range commonly expected for
acoustic emissions (Teague et al., 2016). Hence, hav-
BIODEVICES 2025 - 18th International Conference on Biomedical Electronics and Devices
50