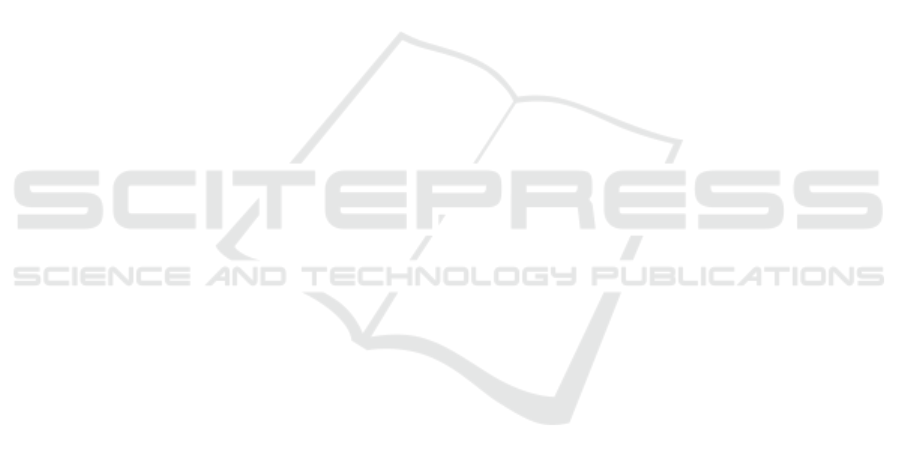
fluence the LLM output in terms of matching associ-
ations between the game partners. However, further
experiments are needed to optimize the ACT-R model
and the generated system prompts for the LLM, as
well as an evaluation of the quality of the association
models in studies with different people.
In future work, language abstractions could also
be used to describe, for example, situations or objects
that a robot has to deal with. All kinds of real-world
data that the robot can collect via its sensors could be
used as a basis for this. Word associations could be
a good way to find a common ground between robots
and humans and a grounded cognition for the social
robot.
REFERENCES
Aldebaran, United Robotics Group and Softbank Robotics
(2024). Pepper. Technical report.
Anderson, J. R., Bothell, D., Byrne, M. D., Douglass, S.,
Lebiere, C., and Qin, Y. (2004). An integrated theory
of the mind. In Psychological review, volume 111,
pages 1036–1060.
Bao, M., Li, J., Zhang, J., Peng, H., and Liu, X. (2019).
Learning semantic coherence for machine generated
spam text detection. In 2019 International Joint Con-
ference on Neural Networks (IJCNN), pages 1–8.
Birlo, M. and Tapus, A. (2011). The crucial role of robot
self-awareness in hri. In Proceedings of the 6th In-
ternational Conference on Human Robot Interaction,
pages 115–116.
Brown, T., Mann, B., Ryder, N., Subbiah, M., Kaplan, J.,
Dhariwal, P., Neelakantan, A., Shyam, P., Sastry, G.,
Askell, A., Agarwal, S., Herbert-Voss, A., Krueger,
G., Henighan, T., Child, R., Ramesh, A., Ziegler, D.,
Wu, J., Winter, C., and Amodei, D. (2020). Language
models are few-shot learners.
Gross, O. (2016). Word Associations as a Language Model
for Generative and Creative Tasks. PhD thesis, Fin-
land.
Gross, O., Toivonen, H., Toivanen, J. M., and Valitutti,
A. (2012). Lexical creativity from word associa-
tions. In 2012 Seventh International Conference on
Knowledge, Information and Creativity Support Sys-
tems, pages 35–42.
Huwel, S., Wrede, B., and Sagerer, G. (2006). Robust
speech understanding for multi-modal human-robot
communication. In ROMAN 2006 - The 15th IEEE
International Symposium on Robot and Human Inter-
active Communication, pages 45–50.
Krunic, V., Salvi, G., Bernardino, A., Montesano, L., and
Santos-Victor, J. (2009). Affordance based word-
to-meaning association. In 2009 IEEE International
Conference on Robotics and Automation, pages 4138–
4143.
Mimno, D., Wallach, H. M., Talley, E., Leenders, M., and
McCallum, A. (2011). Optimizing semantic coher-
ence in topic models. In Proceedings of the Confer-
ence on Empirical Methods in Natural Language Pro-
cessing, EMNLP ’11, page 262–272, USA. Associa-
tion for Computational Linguistics.
OpenAI (2024). Transforming work and creativity with ai.
Technical report.
Rasheed, N., Amin, S., Hafeez, A., Raheem, A., and
Shakoor, R. (2015). Acquisition of abstract words
for cognitive robots [isi index x category]. Jurnal
Teknologi, 72.
Sievers, T. and Russwinkel, N. (2024). How to use a cog-
nitive architecture for a dynamic person model with a
social robot in human collaboration. In CEUR Work-
shop Proceedings.
Silverman, L. B. (2013). Verbal Semantic Coherence.
Springer New York, New York, NY.
Sreedharan, S., Kulkarni, A., and Kambhampati, S. (2024).
Explainable human-ai interaction: A planning per-
spective.
Steels, L. (2001). Language games for autonomous robots.
IEEE Intelligent Systems, 16(5):16–22.
Werk, A., Scholz, S., Sievers, T., and Russwinkel, N.
(2024). How to provide a dynamic cognitive person
model of a human collaboration partner to a pepper
robot. In Society for Mathematical Psychology.
Zhang, H., Li, L., Meng, T., Chang, K.-W., and Broeck,
G. (2022). On the paradox of learning to reason from
data.
ICAART 2025 - 17th International Conference on Agents and Artificial Intelligence
410