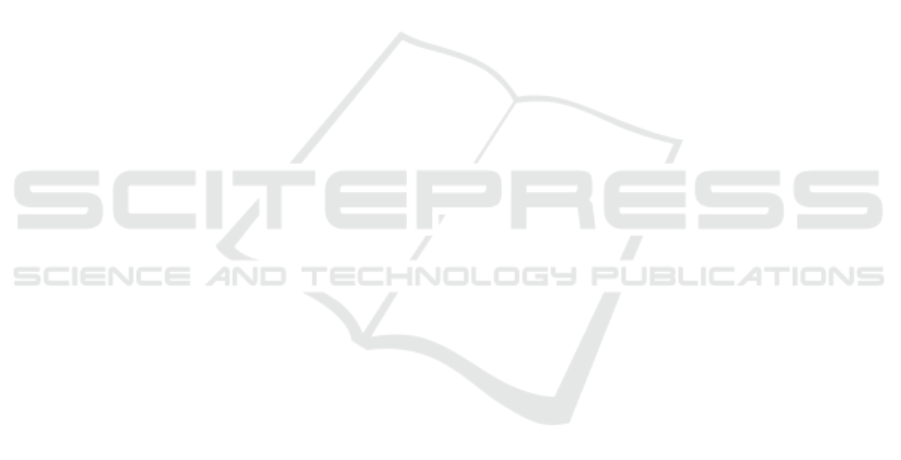
Burger, B. and Toiviainen, P. (2013). MoCap Toolbox – A
Matlab toolbox for computational analysis of move-
ment data. In Bresin, R., editor, Proceedings of the
10th Sound and Music Computing Conference, pages
172–178, Stockholm, Sweden. KTH Royal Institute of
Technology.
Callejas-Cuervo, M., Espitia-Mora, L. A., and V
´
elez-
Guerrero, M. A. (2023). Review of optical and inertial
technologies for lower body motion capture. Journal
of Hunan University Natural Sciences, 50(6).
Geng, W. and Yu, G. (2003). Reuse of motion capture data
in animation: A review. In International Conference
on Computational Science and Its Applications, pages
620–629. Springer.
Hachaj, T. and Ogiela, M. (2020). Rmocap: an r language
package for processing and kinematic analyzing mo-
tion capture data. Multimedia Systems, 26.
Harvey, F. G., Yurick, M., Nowrouzezahrai, D., and Pal, C.
(2020). Robust motion in-betweening. ACM Trans.
Graph., 39(4).
Holden, D. (2018). Robust solving of optical motion cap-
ture data by denoising. ACM Transactions on Graph-
ics (TOG), 37(7):1–12.
Hoxey, T. and Stephenson, I. (2018). Smoothing noisy
skeleton data in real time. In EG 2018 - Posters. The
Eurographics Association.
Ijjina, E. P. and Mohan, C. K. (2014). Human action recog-
nition based on mocap information using convolution
neural networks. In 2014 13th International Confer-
ence on Machine Learning and Applications, pages
159–164.
Ionescu, C., Papava, D., Olaru, V., and Sminchisescu, C.
(2014). Human3.6m: Large scale datasets and pre-
dictive methods for 3d human sensing in natural envi-
ronments. IEEE Transactions on Pattern Analysis and
Machine Intelligence, 36(7):1325–1339.
Iqbal, A., Amin, R., Alsubaei, F. S., and Alzahrani, A.
(2024). Anomaly detection in multivariate time se-
ries data using deep ensemble models. PLOS ONE,
19(6):1–25.
Kadu, H. and Kuo, C.-C. J. (2014). Automatic human mo-
cap data classification. IEEE Transactions on Multi-
media, 16(8):2191–2202.
Kobayashi, M., Liao, C.-C., Inoue, K., Yojima, S., and
Takahashi, M. (2023). Motion capture dataset for
practical use of ai-based motion editing and styliza-
tion.
Liu, X., Ming Cheung, Y., Peng, S.-J., Cui, Z., Zhong,
B., and Du, J.-X. (2014). Automatic motion capture
data denoising via filtered subspace clustering and low
rank matrix approximation. Signal Process., 105:350–
362.
Ma, M., Zhang, S., Chen, J., Xu, J., Li, H., Lin, Y., Nie,
X., Zhou, B., Wang, Y., and Pei, D. (2021). Jump-
Starting multivariate time series anomaly detection for
online service systems. In 2021 USENIX Annual Tech-
nical Conference (USENIX ATC 21), pages 413–426.
USENIX Association.
Mahmood, N., Ghorbani, N., Troje, N. F., Pons-Moll, G.,
and Black, M. J. (2019). AMASS: Archive of motion
capture as surface shapes. In International Conference
on Computer Vision, pages 5442–5451.
Manns, M., Otto, M., and Mauer, M. (2016). Measuring
motion capture data quality for data driven human mo-
tion synthesis. Procedia CIRP, 41:945–950. Research
and Innovation in Manufacturing: Key Enabling Tech-
nologies for the Factories of the Future - Proceedings
of the 48th CIRP Conference on Manufacturing Sys-
tems.
Menolotto, M., Komaris, D.-S., Tedesco, S., O’Flynn, B.,
and Walsh, M. (2020). Motion capture technology in
industrial applications: A systematic review. Sensors,
20(19):5687.
Meredith, M. and Maddock, S. C. (2001). Motion capture
file formats explained. Department of Computer Sci-
ence, University of Sheffield.
Montes, V. R., Quijano, Y., Chong Quero, J. E., Ayala,
D. V., and P
´
erez Moreno, J. C. (2014). Comparison
of 4 different smoothness metrics for the quantitative
assessment of movement’s quality in the upper limb
of subjects with cerebral palsy. In 2014 Pan American
Health Care Exchanges (PAHCE), pages 1–6.
Nawrocki, P. and Sus, W. (2022). Anomaly detection in the
context of long-term cloud resource usage planning.
Knowl. Inf. Syst., 64(10):2689–2711.
Oreshkin, B. N., Valkanas, A., Harvey, F. G., M
´
enard, L.-
S., Bocquelet, F., and Coates, M. J. (2024). Mo-
tion in-betweening via deep δ-interpolator. IEEE
Transactions on Visualization and Computer Graph-
ics, 30(8):5693–5704.
Patrona, F., Chatzitofis, A., Zarpalas, D., and Daras, P.
(2018). Motion analysis: Action detection, recogni-
tion and evaluation based on motion capture data. Pat-
tern Recognition, 76:612–622.
Qin, J., Zheng, Y., and Zhou, K. (2022). Motion in-
betweening via two-stage transformers. ACM Trans.
Graph., 41(6).
Ren, T., Yu, J., Guo, S., Ma, Y., Ouyang, Y., Zeng, Z.,
Zhang, Y., and Qin, Y. (2023). Diverse motion in-
betweening from sparse keyframes with dual posture
stitching. IEEE Transactions on Visualization & Com-
puter Graphics, (01):1–12.
Skurowski, P. and Pawlyta, M. (2022). Detection and classi-
fication of artifact distortions in optical motion capture
sequences. Sensors, 22(11).
GRAPP 2025 - 20th International Conference on Computer Graphics Theory and Applications
88