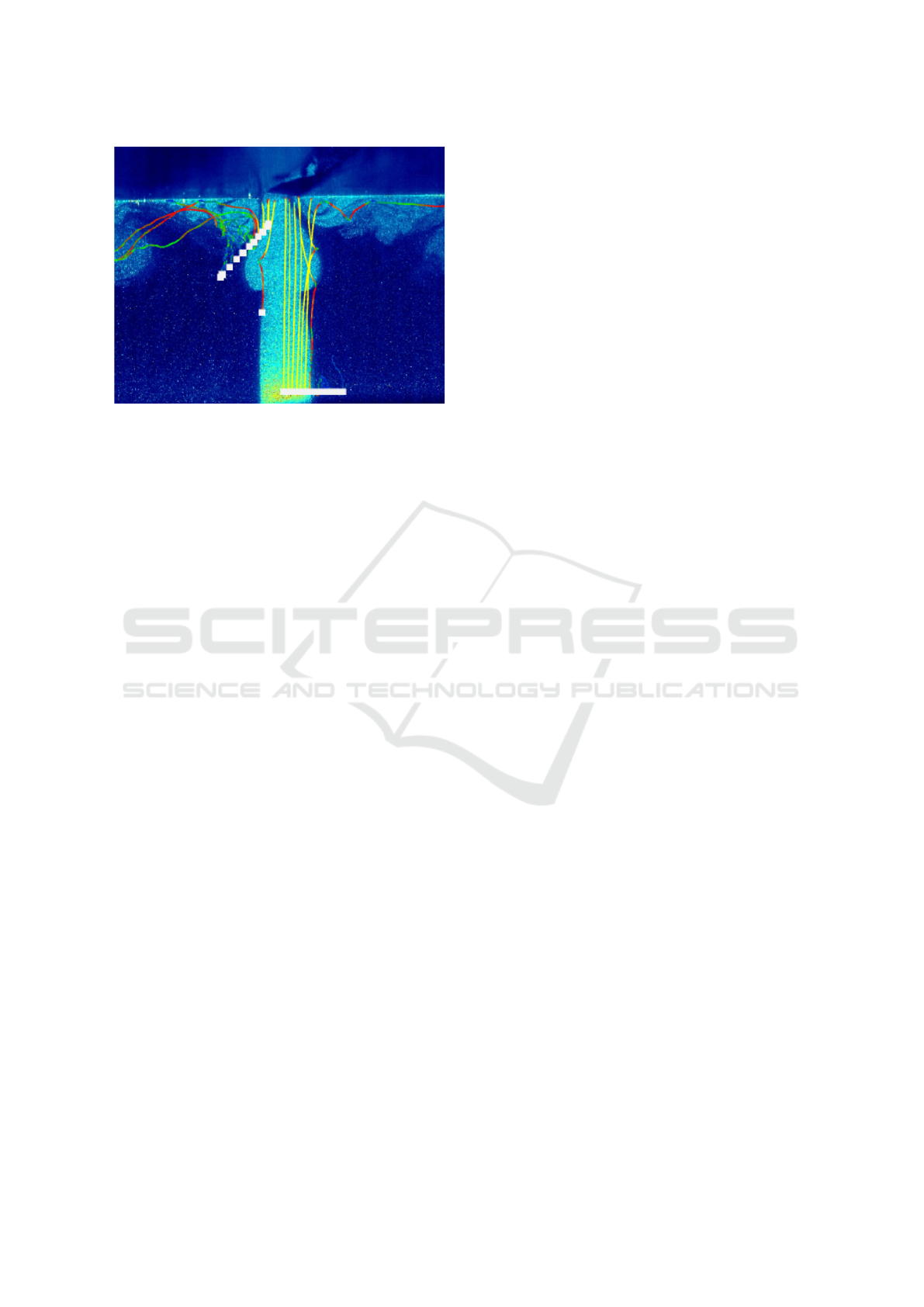
Figure 9: Visualisation of particles selected from the user
interface: (b) single particle, (a,c) groups of particles.
visualisation becomes possible by registering datasets
into a shared space. This would provide a more im-
mersive view of flow phenomena, enhancing both vi-
sualisation and analysis.
Another interesting feature would be to compare
flow predictions coming from simulations, to pre-
dict the acoustic effects generated by an experimental
configuration. Such capabilities would enable physi-
cists to predict noise production in advance, reduc-
ing costly trial-and-error experimentation and aiding
in designing quieter, more efficient systems.
ACKNOWLEDGEMENTS
This work has been funded by the VIPER project (Ré-
gion Nouvelle Aquitaine), and MIRES federation for
research CNRS (FR3423).
REFERENCES
Ahrens, J., Geveci, B., Law, C., Hansen, C., and John-
son, C. (2005). 36-paraview: An end-user tool for
large-data visualization. The Visualization Handbook,
717:50038–1.
Assoum, H. H., Hamdi, J., El Hassan, M., Mrach, T.,
Abed Meraim, K., and Sakout, A. (2020). Energy
transfers between aerodynamic and acoustic fields in
a rectangular impinging jet. Energy Reports, 6:812–
816.
Ayachit, U., Bauer, A., Geveci, B., O’Leary, P., Moreland,
K., Fabian, N., and Mauldin, J. (2015). Paraview cat-
alyst: Enabling in situ data analysis and visualization.
In Proceedings of the First Workshop on In Situ Infras-
tructures for Enabling Extreme-Scale Analysis and Vi-
sualization, pages 25–29.
Ayachit, U., Whitlock, B., Wolf, M., Loring, B., Geveci,
B., Lonie, D., and Bethel, E. W. (2016). The sen-
sei generic in situ interface. In 2016 Second Work-
shop on In Situ Infrastructures for Enabling Extreme-
Scale Analysis and Visualization (ISAV), pages 40–44.
IEEE.
Bush, J. W. (2004). Surface tension mod-
ule. URL http://web.mit.edu/1.63/www/Lec-
notes/Surfacetension.
Childs, H., Brugger, E., Whitlock, B., Meredith, J., Ahern,
S., Pugmire, D., Biagas, K., Miller, M., Harrison, C.,
Weber, G. H., et al. (2012). Visit: An end-user tool for
visualizing and analyzing very large data.
DaVIS (2024). https://www.lavision.de/en/products/davis-
software/.
Dorier, M., Sisneros, R., Peterka, T., Antoniu, G., and
Semeraro, D. (2013). Damaris/viz: A nonintru-
sive, adaptable and user-friendly in situ visualization
framework. In 2013 IEEE Symposium on Large-Scale
Data Analysis and Visualization (LDAV), pages 67–
75. IEEE.
El Zohbi, B., Assoum, H. H., Alkheir, M., Afyouni,
N., Abed Meraim, K., Sakout, A., and El Hassan,
M. (2024). Experimental investigation of the aero-
acoustics of a rectangular jet impinging a slotted plate
for different flow regimes. Alexandria Engineering
Journal, 87:404–416.
Graftieaux, L., Michard, M., and Grosjean, N. (2001).
Combining piv, pod and vortex identification algo-
rithms for the study of unsteady turbulent swirling
flows. Measurement Science and Technology,
12(9):1422.
Gutmark, E., Wolfshtein, M., and Wygnanski, I. (1978).
The plane turbulent impinging jet. Journal of Fluid
Mechanics, 88(4):737–756.
Kuhlen, T., Pajarola, R., and Zhou, K. (2011). Parallel in
situ coupling of simulation with a fully featured vi-
sualization system. In Proceedings of the 11th Euro-
graphics Conference on Parallel Graphics and Visu-
alization (EGPGV), volume 10, pages 101–109. Eu-
rographics Association.
Sakakibara, J., Hishida, K., and Phillips, W. R. (2001). On
the vortical structure in a plane impinging jet. Journal
of Fluid Mechanics, 434:273–300.
Sarton, J., Zellmann, S., Demirci, S., Güdükbay, U.,
Alexandre-Barff, W., Lucas, L., Dischler, J.-M., Wes-
ner, S., and Wald, I. (2023). State-of-the-art in large-
scale volume visualization beyond structured data. In
Computer Graphics Forum, volume 42, pages 491–
515. Wiley Online Library.
Sommerfeld, A. (1909). Ein beitrag zur hydrodynamischen
erklärung der turbulenten flüssigkeitsbewegungen. In-
ternational Congress of Mathematicians, 3:116–124.
Yokobori, S., Kasagi, N., and Hirata, M. (1983). Trans-
port phenomena at the stagnation region of a
two-dimensional impinging jet. Trans. JSME B,
49(441):1029–1039.
GRAPP 2025 - 20th International Conference on Computer Graphics Theory and Applications
230