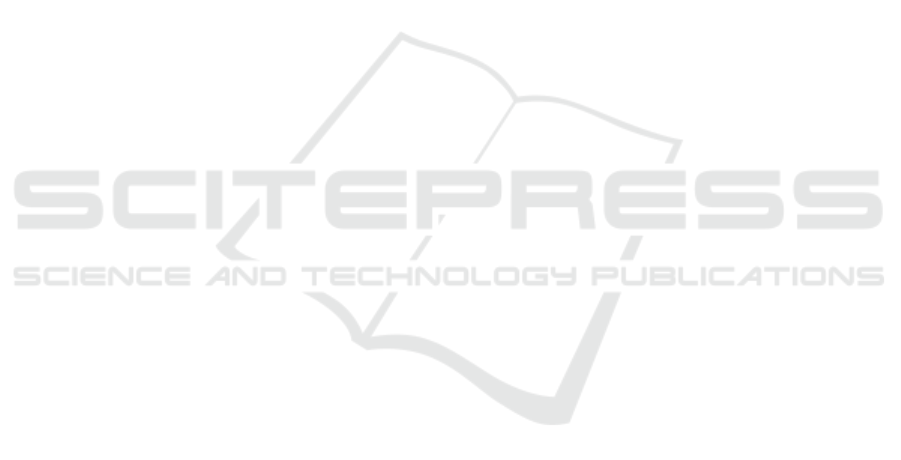
evaluating a specification from trained agents. Our
findings indicate that single goals with sparse re-
wards, often, do not provide enough feedback for
effective learning. However, the results of our
V
-
operator consistently improve by providing a refined
reward for the goal. Additionally, the
V
-operator en-
ables to balance possibly conflicting goals by weight-
ing the inner reward components while the distance
annotations help to guide the agent towards other-
wise challenging goals. In contrast, we would like
to point out that goal tree refinements do not always
yield improvements in learning the goals. Therefore,
iterations can also include reverting or adapting prior
changes to the specification. Nevertheless, our results
show that we can iteratively improve on learning the
specified goals. In the following section, we conclude
and present future work.
7 CONCLUSION
In this work, we have introduced iterative environ-
ment design for reinforcement learning based on goal-
oriented specification (Schwan et al., 2023). We
evolve goal-oriented specification and make it practi-
cal with two contributions. First, we introduce our au-
tomated method to construct RL environments from
goal tree specifications. Thereby, we enable the train-
ing of agents from these specifications to evaluate
their behavior for future improvements. Second, we
enable iterative goal tree refinements by introducing
definitions for leaf nodes, the
V
-operator and annota-
tions. To evaluate our method, we have trained agents
in four case studies with up to three specification sce-
narios each. With manually tuned weights of the re-
ward components, we achieve goal success rates sim-
ilar to the baselines but with higher precision. Finally,
our results show that goal tree refinements can be used
to iteratively improve the learning of specified goals.
Through iterative environment design, we oppose the
common trial-and-error practice to facilitate the ap-
plication of reinforcement learning.
In future work, we plan on automating the man-
ual weighting of reward components from our
V
-
operator to further reduce time-consuming manual
tasks. Moreover, we aim at enhancing our specifica-
tion method to be practical for high-dimensional state
spaces. Finally, introducing new operators can enable
specifying and learning temporal abstractions. With
this, we follow our idea to overcome the common
trial-and-error practice and facilitate the development
of RL solution for domain experts.
ACKNOWLEDGEMENTS
This work has been partially funded by the Fed-
eral Ministry of Education and Research as part of
the Software Campus project ZoLA - Ziel-orientiertes
Lernen von Agenten (funding code 01IS23068).
REFERENCES
Ahmad, K., Abdelrazek, M., Arora, C., Bano, M., and
Grundy, J. (2023). Requirements engineering for
artificial intelligence systems: A systematic map-
ping study. Information and Software Technology,
158:107176.
Andrychowicz, M., Wolski, F., Ray, A., Schneider, J., Fong,
R., Welinder, P., McGrew, B., Tobin, J., Abbeel, P.,
and Zaremba, W. (2017). Hindsight experience re-
play. In Advances in Neural Information Processing
Systems, pages 5048–5058.
Cai, M., Xiao, S., Li, B., Li, Z., and Kan, Z. (2021). Re-
inforcement Learning Based Temporal Logic Control
with Maximum Probabilistic Satisfaction. In 2021
IEEE International Conference on Robotics and Au-
tomation (ICRA), pages 806–812.
Chane-Sane, E., Schmid, C., and Laptev, I. (2021). Goal-
Conditioned Reinforcement Learning with Imagined
Subgoals. In Proceedings of the 38th International
Conference on Machine Learning, volume 139, pages
1430–1440.
Ding, H., Tang, Y., Wu, Q., Wang, B., Chen, C.,
and Wang, Z. (2023). Magnetic Field-Based Re-
ward Shaping for Goal-Conditioned Reinforcement
Learning. IEEE/CAA Journal of Automatica Sinica,
10(12):2233–2247.
DLR-RM (2024a). RL Baselines3 Zoo: A Train-
ing Framework for Stable Baselines3 Reinforce-
ment Learning Agents. https://github.com/DLR-RM/
rl-baselines3-zoo. [Last accessed on July 17th, 2024].
DLR-RM (2024b). Stable-Baselines3. https://github.com/
DLR-RM/stable-baselines3. [Last accessed on July
17th, 2024].
Everett, M., Chen, Y. F., and How, J. P. (2021). Colli-
sion avoidance in pedestrian-rich environments with
deep reinforcement learning. IEEE Access, 9:10357–
10377.
Farama Foundation (2024). Gymnasium: An API standard
for reinforcement learning with a diverse collection of
reference environments. https://gymnasium.farama.
org/. [Last accessed on July 12th, 2024].
Florensa, C., Held, D., Geng, X., and Abbeel, P. (2018).
Automatic goal generation for reinforcement learning
agents. In International conference on machine learn-
ing, pages 1515–1528.
Hahn, E. M., Perez, M., Schewe, S., Somenzi, F., Trivedi,
A., and Wojtczak, D. (2019). Omega-Regular Objec-
tives in Model-Free Reinforcement Learning. In Tools
and Algorithms for the Construction and Analysis of
Systems, pages 395–412.
ICAART 2025 - 17th International Conference on Agents and Artificial Intelligence
250