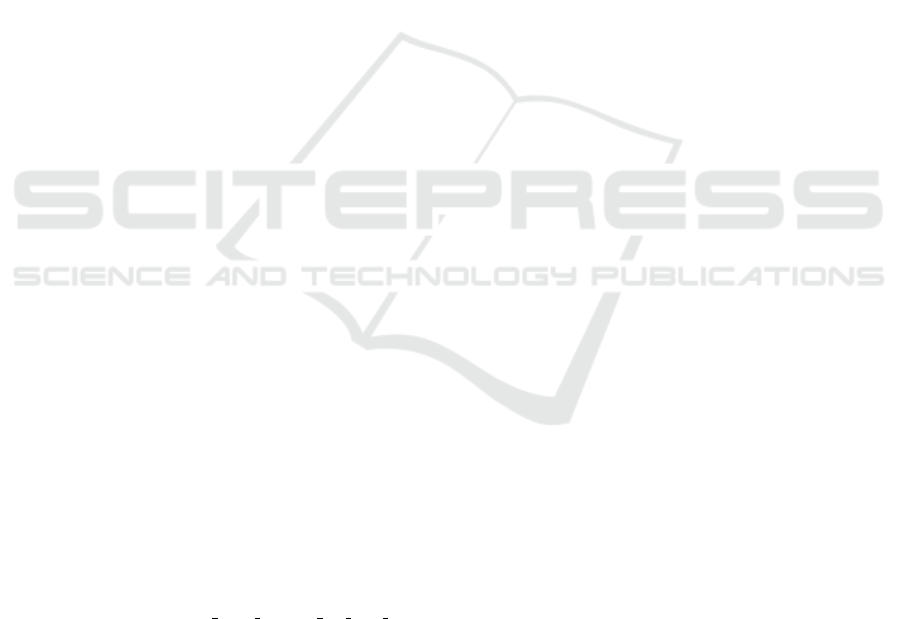
ergy savings.
Future work is planned to address per-application
power estimation, as current solutions lack the de-
sired accuracy. Additionally, we aim to explore car-
bon objectives built on top of power objectives. One
limitation of the current solution is the need for core
pinning, which could be replaced with a more flexi-
ble model in the future. Better-informed resource al-
location can help the scheduler make more efficient
decisions based on inputs from the planner, enhanc-
ing fleet-wide performance and energy efficiency. For
example, consolidating workloads with similar power
profile needs can be more efficient, avoiding single
application’s high-performance demands increase the
overall power draw of a node.
By integrating these advancements, the IDO
model provides a step towards managing the increas-
ing compute demands driven by AI and ML appli-
cations, ensuring that power and energy management
strategies remain effective and user-friendly.
ACKNOWLEDGEMENTS
This paper was in-part supported by European
Union’s Horizon Europe research and innovation pro-
gramme under grant agreement No. 101135576. The
authors would like to sincerely thank Francesc Guim,
Denisio Togashi, Karol Weber and Marlow Weston
for their contributions to the IDO capability and the
methodologies discussed in this paper.
REFERENCES
Bobroff, N., Kochut, A., and Beaty, K. (2007). Dynamic
placement of virtual machines for managing sla viola-
tions. In 2007 10th IFIP/IEEE International Sympo-
sium on Integrated Network Management, pages 119–
128.
Cai, X., Deng, H., Deng, L., Elsawaf, A., Gao, S.,
Martin de Nicolas, A., Nakajima, Y., Pieczerak,
J., Triay, J., Wang, X., Xie, B., and Zafar, H.
(2023). Evolving nfv towards the next decade.
https://www.etsi.org/images/files/ETSIWhitePapers/
ETSI-WP-54-Evolving NFV towards the next
decade.pdf. [Accessed 10-07-2024].
Geurts, P., Ernst, D., and Wehenkel, L. (2006). Extremely
randomized trees. Mach. Learn., 63(1):3–42.
Guim, F., Metsch, T., Moustafa, H., Verrall, T., Carrera, D.,
Cadenelli, N., Chen, J., Doria, D., Ghadie, C., and
Prats, R. G. (2022). Autonomous lifecycle manage-
ment for resource-efficient workload orchestration for
green edge computing. IEEE Transactions on Green
Communications and Networking, 6(1):571–582.
Intel Network Builders (2024). Elastic and energy pro-
portional edge computing infrastructure solution brief.
Intel.
Lin, L. and Chien, A. A. (2023). Adapting datacenter capac-
ity for greener datacenters and grid. In Proceedings of
the 14th ACM International Conference on Future En-
ergy Systems, e-Energy ’23, page 200–213, New York,
NY, USA. Association for Computing Machinery.
Lin, L., Wijayawardana, R., Rao, V., Nguyen, H.,
GNIBGA, E. W., and Chien, A. A. (2024). Exploding
ai power use: an opportunity to rethink grid planning
and management. In Proceedings of the 15th ACM
International Conference on Future and Sustainable
Energy Systems, e-Energy ’24, page 434–441, New
York, NY, USA. Association for Computing Machin-
ery.
Metsch, T., Ibidunmoye, O., Bayon-Molino, V., Butler,
J., Hern
´
andez-Rodriguez, F., and Elmroth, E. (2015).
Apex lake: A framework for enabling smart orches-
tration. In Proceedings of the Industrial Track of the
16th International Middleware Conference, Middle-
ware Industry ’15, pages 1–7, New York, NY, USA.
Association for Computing Machinery.
Metsch, T., Viktorsson, M., Hoban, A., Vitali, M., Iyer, R.,
and Elmroth, E. (2023). Intent-driven orchestration:
Enforcing service level objectives for cloud native de-
ployments. SN Computer Science, 4(3).
Patel, P., Choukse, E., Zhang, C., Goiri, I. n., Warrier, B.,
Mahalingam, N., and Bianchini, R. (2024). Charac-
terizing power management opportunities for llms in
the cloud. In Proceedings of the 29th ACM Interna-
tional Conference on Architectural Support for Pro-
gramming Languages and Operating Systems, Volume
3, ASPLOS ’24, page 207–222, New York, NY, USA.
Association for Computing Machinery.
Pereira, R., Couto, M., Ribeiro, F., Rua, R., Cunha, J.,
Fernandes, J. a. P., and Saraiva, J. a. (2017). En-
ergy efficiency across programming languages: how
do energy, time, and memory relate? In Proceedings
of the 10th ACM SIGPLAN International Conference
on Software Language Engineering, SLE 2017, page
256–267, New York, NY, USA. Association for Com-
puting Machinery.
Roy, N., Dubey, A., and Gokhale, A. (2011). Efficient
autoscaling in the cloud using predictive models for
workload forecasting. In 2011 IEEE 4th International
Conference on Cloud Computing, pages 500–507.
Tom
´
as, L. and Tordsson, J. (2013). Improving cloud infras-
tructure utilization through overbooking. In Proceed-
ings of the 2013 ACM Cloud and Autonomic Comput-
ing Conference, CAC ’13, pages 1–10, New York, NY,
USA. Association for Computing Machinery.
Veitch, P., MacNamara, C., and Browne, J. J. (2024). Un-
core frequency tuning for energy efficiency in mixed
priority cloud native edge. In 2024 Joint European
Conference on Networks and Communications & 6G
Summit (EuCNC/6G Summit), pages 925–930.
SMARTGREENS 2025 - 14th International Conference on Smart Cities and Green ICT Systems
34