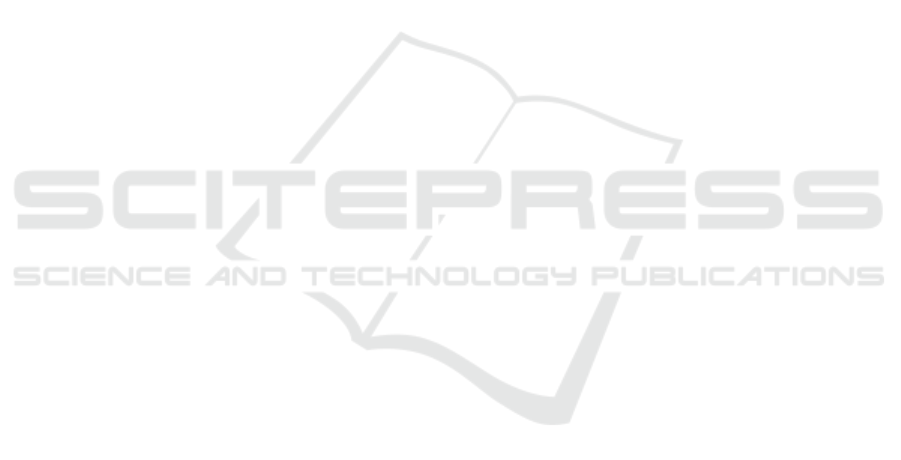
formed better than earlier approaches on the UBFC-
Phy dataset in both sets of experiments. Future re-
search may examine the possibilities of combining
speech and eye gaze data with rPPG signals to assess
stress.
REFERENCES
Arik, S.
¨
O. and Pfister, T. (2021). Tabnet: Attentive inter-
pretable tabular learning. In Proceedings of the AAAI
conference on artificial intelligence, volume 35, pages
6679–6687.
Barazi, N., Polidovitch, N., Debi, R., Yakobov, S., Lakin,
R., and Backx, P. H. (2021). Dissecting the roles of
the autonomic nervous system and physical activity
on circadian heart rate fluctuations in mice. Frontiers
in physiology, 12:692247.
Casado, C.
´
A., Ca
˜
nellas, M. L., and L
´
opez, M. B. (2023).
Depression recognition using remote photoplethys-
mography from facial videos. IEEE Transactions on
Affective Computing, 14(4):3305–3316.
Choi, C.-H., Kim, J., Hyun, J., Kim, Y., and Moon, B. Face
detection using haar cascade classifiers based on ver-
tical component calibration.
Das, M., Bhuyan, M. K., and Sharma, L. N. (2023).
Time–frequency learning framework for rppg signal
estimation using scalogram-based feature map of fa-
cial video data. IEEE Transactions on Instrumenta-
tion and Measurement, 72:1–10.
Dolmans, T. C., Poel, M., van’t Klooster, J.-W. J., and
Veldkamp, B. P. (2021). Perceived mental work-
load classification using intermediate fusion multi-
modal deep learning. Frontiers in human neuro-
science, 14:609096.
Fink, G. (2010). Stress: Definition and history. Stress sci-
ence: neuroendocrinology, 3(9):3–14.
Gao, P., Jiang, Z., You, H., Lu, P., Hoi, S. C., Wang, X., and
Li, H. (2019). Dynamic fusion with intra-and inter-
modality attention flow for visual question answer-
ing. In Proceedings of the IEEE/CVF conference on
computer vision and pattern recognition, pages 6639–
6648.
Giannakakis, G., Grigoriadis, D., Giannakaki, K., Simanti-
raki, O., Roniotis, A., and Tsiknakis, M. (2019). Re-
view on psychological stress detection using biosig-
nals. IEEE transactions on affective computing,
13(1):440–460.
Imambi, S., Prakash, K. B., and Kanagachidambaresan, G.
(2021). Pytorch. Programming with TensorFlow: so-
lution for edge computing applications, pages 87–104.
Kim, N. H., Yu, S.-G., Kim, S.-E., and Lee, E. C. (2021).
Non-contact oxygen saturation measurement using
ycgcr color space with an rgb camera. Sensors,
21(18):6120.
Lugaresi, C., Tang, J., Nash, H., McClanahan, C., Uboweja,
E., Hays, M., Zhang, F., Chang, C.-L., Yong, M. G.,
Lee, J., et al. (2019). Mediapipe: A framework
for building perception pipelines. arXiv preprint
arXiv:1906.08172.
Mordvintsev, A. and Abid, K. (2017). Opencv-python tuto-
rials documentation.
Ntalampiras, S. (2023). Model ensemble for predicting
heart and respiration rate from speech. IEEE Internet
Computing, 27(3):15–20.
Pan, Y., Shang, Y., Liu, T., Shao, Z., Guo, G., Ding, H., and
Hu, Q. (2024). Spatial–temporal attention network for
depression recognition from facial videos. Expert Sys-
tems with Applications, 237:121410.
Panigrahi, A. and Sharma, H. (2022). Non-contact hr ex-
traction from different color spaces using rgb camera.
In National Conference on Communications (NCC),
pages 332–337.
Praveen, R. G., Granger, E., and Cardinal, P. (2021). Cross
attentional audio-visual fusion for dimensional emo-
tion recognition. In 16th IEEE International Con-
ference on Automatic Face and Gesture Recognition,
pages 1–8.
Sabour, R. M., Benezeth, Y., De Oliveira, P., Chappe, J., and
Yang, F. (2021). Ubfc-phys: A multimodal database
for psychophysiological studies of social stress. IEEE
Transactions on Affective Computing, 14(1):622–636.
Selesnick, I. W. and Burrus, C. S. (1998). Generalized dig-
ital butterworth filter design. IEEE Transactions on
signal processing, 46(6):1688–1694.
Speth, J., Vance, N., Sporrer, B., Niu, L., Flynn, P., and
Czajka, A. (2024). Mspm: A multi-site physio-
logical monitoring dataset for remote pulse, respira-
tion, and blood pressure estimation. arXiv preprint
arXiv:2402.02224.
Wang, W., Den Brinker, A. C., Stuijk, S., and De Haan,
G. (2016). Algorithmic principles of remote
ppg. IEEE Transactions on Biomedical Engineering,
64(7):1479–1491.
Xu, J., Song, C., Yue, Z., and Ding, S. (2024). Facial video-
based non-contact stress recognition utilizing multi-
task learning with peak attention. IEEE Journal of
Biomedical and Health Informatics.
Yu, H., Vaessen, T., Myin-Germeys, I., and Sano, A. (2021).
Modality fusion network and personalized attention in
momentary stress detection in the wild. In 9th Inter-
national Conference on Affective Computing and In-
telligent Interaction (ACII), pages 1–8.
Zhang, X., Wei, X., Zhou, Z., Zhao, Q., Zhang, S., Yang,
Y., Li, R., and Hu, B. (2023). Dynamic alignment and
fusion of multimodal physiological patterns for stress
recognition. IEEE Transactions on Affective Comput-
ing.
Ziaratnia, S., Laohakangvalvit, T., Sugaya, M., and Srip-
ian, P. (2024). Multimodal deep learning for remote
stress estimation using cct-lstm. In Proceedings of
the IEEE/CVF Winter Conference on Applications of
Computer Vision, pages 8336–8344.
ICPRAM 2025 - 14th International Conference on Pattern Recognition Applications and Methods
604