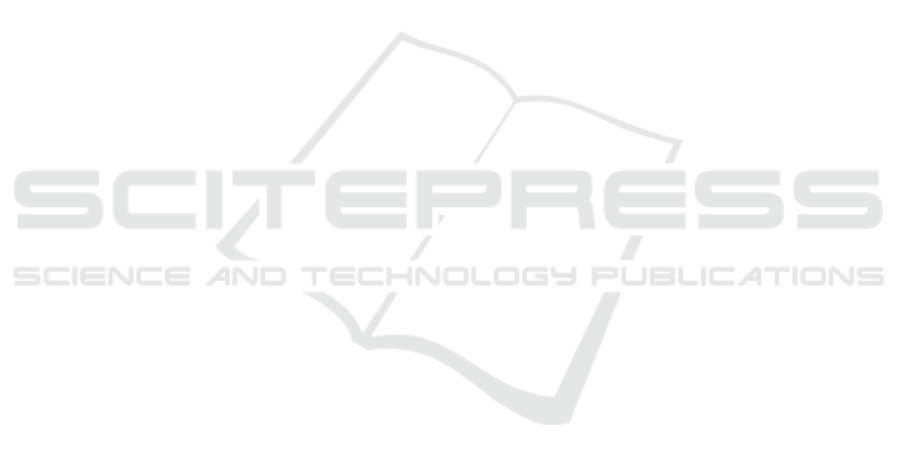
lenses and 12-bit images could facilitate more accu-
rate categorisation compared to the same NNs using
8-bit RGB images of objects that resemble the same
but are made of different materials.
ACKNOWLEDGEMENTS
Research work was funded by grant
PID2021-122685OB-I00 funded by MI-
CIU/AEI/10.13039/501100011033 and ERDF/EU.
REFERENCES
Andersson, P., Montan, S., and Svanberg, S. (1987). Mul-
tispectral system for medical fluorescence imaging.
IEEE Journal of Quantum Electronics, 23(10):1798–
1805.
Benjamin, MatvL, midaha, PGibson, RMcKinlay, and Kan,
W. (2016). Dstl satellite imagery feature detection.
Gallagher, J. E., Gogia, A., and Oughton, E. J. (2024). A
multispectral automated transfer technique (matt) for
machine-driven image labeling utilizing the segment
anything model (sam). arXiv preprint.
G
´
omez, P. and Meoni, G. (2021). Msmatch: Semisuper-
vised multispectral scene classification with few la-
bels. IEEE Journal of Selected Topics in Applied
Earth Observations and Remote Sensing, 14:11643–
11654.
Helber, P., Bischke, B., Dengel, A., and Borth, D. (2019).
Eurosat: A novel dataset and deep learning bench-
mark for land use and land cover classification. IEEE
Journal of Selected Topics in Applied Earth Observa-
tions and Remote Sensing, 12(7):2217–2226.
Hoffer, R., Johannsen, C., and Baumgardner, M. (1966).
Agricultural applications of remote multispectral
sensing. In Proceedings of the Indiana Academy of
Science, volume 76, pages 386–396.
Kemker, R., Salvaggio, C., and Kanan, C. (2018). Al-
gorithms for semantic segmentation of multispectral
remote sensing imagery using deep learning. ISPRS
Journal of Photogrammetry and Remote Sensing.
Kirillov, A., Mintun, E., Ravi, N., Mao, H., Rolland, C.,
Gustafson, L., Xiao, T., Whitehead, S., Berg, A. C.,
Lo, W.-Y., et al. (2023). Segment anything. In Pro-
ceedings of the IEEE/CVF International Conference
on Computer Vision, pages 4015–4026.
Lowe, D. G. (1999). Object recognition from local scale-
invariant features. In Proceedings of the seventh
IEEE international conference on computer vision,
volume 2, pages 1150–1157. IEEE.
Ma, F., Yuan, M., and Kozak, I. (2023). Multispectral imag-
ing: Review of current applications. Survey of Oph-
thalmology, 68(5):889–904.
Maxwell, E. L. (1976). Multivariate system analysis of mul-
tispectral imagery. Photogrammetric Engineering and
Remote Sensing, 42(9):1173–1186.
Mia, M. S., Tanabe, R., Habibi, L. N., Hashimoto, N.,
Homma, K., Maki, M., Matsui, T., and Tanaka, T.
S. T. (2023). Multimodal deep learning for rice yield
prediction using uav-based multispectral imagery and
weather data. Remote Sensing, 15(10).
O
ˇ
sep, A., Meinhardt, T., Ferroni, F., Peri, N., Ramanan, D.,
and Leal-Taix
´
e, L. (2024). Better call sal: Towards
learning to segment anything in lidar. arXiv preprint.
P
´
aez-Ubieta, I. d. L., Casta
˜
no-Amor
´
os, J., Puente, S. T., and
Gil, P. (2023). Vision and tactile robotic system to
grasp litter in outdoor environments. Journal of Intel-
ligent & Robotic Systems, 109(2):36.
Park, H. G., Yun, J. P., Kim, M. Y., and Jeong, S. H. (2021).
Multichannel object detection for detecting suspected
trees with pine wilt disease using multispectral drone
imagery. IEEE Journal of Selected Topics in Applied
Earth Observations and Remote Sensing, 14:8350–
8358.
P
´
aez-Ubieta, I. d. L., Velasco-S
´
anchez, E., Puente, S. T.,
and Candelas, F. (2023). Detection and depth esti-
mation for domestic waste in outdoor environments
by sensors fusion. IFAC-PapersOnLine, 56(2):9276–
9281. 22nd IFAC World Congress.
Torralba, A., Russell, B. C., and Yuen, J. (2010). Labelme:
Online image annotation and applications. Proceed-
ings of the IEEE, 98(8):1467–1484.
Ulku, I., Akag
¨
und
¨
uz, E., and Ghamisi, P. (2022). Deep se-
mantic segmentation of trees using multispectral im-
ages. IEEE Journal of Selected Topics in Applied
Earth Observations and Remote Sensing, 15:7589–
7604.
Wang, C.-Y., Bochkovskiy, A., and Liao, H.-Y. M. (2023).
Yolov7: Trainable bag-of-freebies sets new state-
of-the-art for real-time object detectors. In 2023
IEEE/CVF Conference on Computer Vision and Pat-
tern Recognition (CVPR), pages 7464–7475. IEEE.
Wang, X., Zhang, R., Kong, T., Li, L., and Shen, C.
(2020). Solov2: Dynamic and fast instance segmenta-
tion. Advances in Neural information processing sys-
tems, 33:17721–17732.
Yang, Y. and Newsam, S. (2010). Bag-of-visual-words and
spatial extensions for land-use classification. In Pro-
ceedings of the 18th SIGSPATIAL international con-
ference on advances in geographic information sys-
tems, pages 270–279.
Yuan, K., Zhuang, X., Schaefer, G., Feng, J., Guan, L., and
Fang, H. (2021). Deep-learning-based multispectral
satellite image segmentation for water body detection.
IEEE Journal of Selected Topics in Applied Earth Ob-
servations and Remote Sensing, 14:7422–7434.
Transferability of Labels Between Multilens Cameras
417