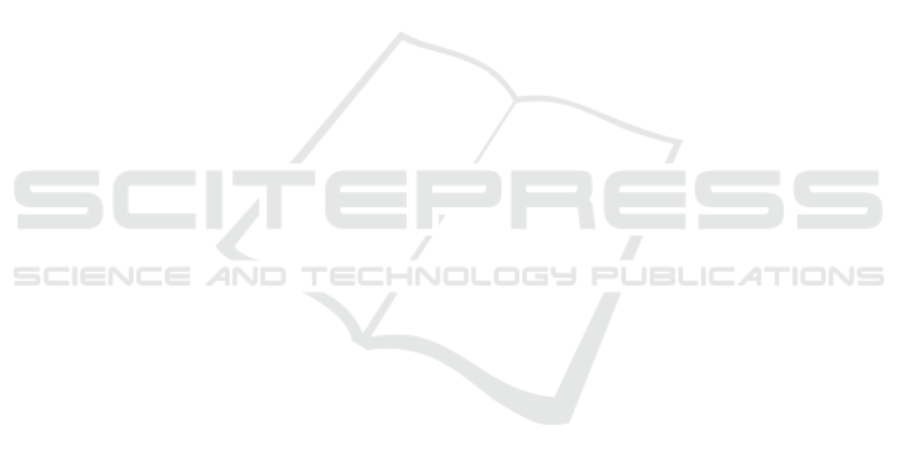
formance of our proposed ABBIE model with other
RNN based architecture.
Overall, this paper shows that bidirectional en-
coders on the direct and reverse input sequences can
be used together with an attention-based module to
get better contextual information on the input se-
quence.
The main limitation of our work, as well as of all
other works on the topic, is that it considers com-
pound words having only one Sandhi split. Despite
we could use our model recursively until no split is
found anymore, in future work, we intend to enhance
our model to also consider the splitting of compound
words which have more than one valid split loca-
tions integrate with the morphological analyzer and
observe the common error patterns.
ACKNOWLEDGMENTS
This work is partially supported by the PNRR
MUR Project ITSERR (Italian Strengthening of
the ESFRI RI RESILIENCE) (IR0000014, CUP
B53C22001770006).
REFERENCES
Aralikatte, R., Gantayat, N., Panwar, N., Sankaran, A., and
Mani, S. (2018). Sanskrit sandhi splitting using seq2
(seq)ˆ 2. arXiv preprint arXiv:1801.00428.
Cai, D. and Zhao, H. (2016). Neural word seg-
mentation learning for chinese. arXiv preprint
arXiv:1606.04300.
Chen, X., Qiu, X., Zhu, C., Liu, P., and Huang, X.-J. (2015).
Long short-term memory neural networks for chinese
word segmentation. In Proceedings of the 2015 con-
ference on empirical methods in natural language pro-
cessing, pages 1197–1206.
Collobert, R., Weston, J., Bottou, L., Karlen, M.,
Kavukcuoglu, K., and Kuksa, P. (2011). Natural lan-
guage processing (almost) from scratch. Journal of
machine learning research, 12:2493–2537.
Dave, S., Singh, A. K., AP, D. P., and Lall, P. B. (2021).
Neural compound-word (sandhi) generation and split-
ting in sanskrit language. In Proceedings of the 3rd
ACM India Joint International Conference on Data
Science & Management of Data (8th ACM IKDD
CODS & 26th COMAD), pages 171–177.
Gong, J., Chen, X., Gui, T., and Qiu, X. (2019). Switch-
lstms for multi-criteria chinese word segmentation. In
Proceedings of the AAAI Conference on Artificial In-
telligence, volume 33, pages 6457–6464.
Goyal, P., Arora, V., and Behera, L. (2007). Analysis of
sanskrit text: Parsing and semantic relations. In In-
ternational Sanskrit Computational Linguistics Sym-
posium, pages 200–218. Springer.
Goyal, P. and Huet, G. (2013). Completeness analysis of a
sanskrit reader. In International Symposium on San-
skrit Computational Linguistics, pages 130–171.
Goyal, P., Huet, G., Kulkarni, A., Scharf, P., and Bunker, R.
(2012). A distributed platform for sanskrit processing.
In Proceedings of COLING 2012, pages 1011–1028.
Haruechaiyasak, C., Kongyoung, S., and Dailey, M.
(2008). A comparative study on thai word segmen-
tation approaches. In 2008 5th International Con-
ference on Electrical Engineering/Electronics, Com-
puter, Telecommunications and Information Technol-
ogy, volume 1, pages 125–128. IEEE.
Hellwig, O. (2010–2021). The Digital Corpus of Sanskrit
(DCS).
Hellwig, O. (2015). Using recurrent neural networks for
joint compound splitting and sandhi resolution in san-
skrit. In 4th Biennial workshop on less-resourced lan-
guages.
Hellwig, O. and Nehrdich, S. (2018). Sanskrit word seg-
mentation using character-level recurrent and convo-
lutional neural networks. In Proceedings of the 2018
conference on empirical methods in natural language
processing, pages 2754–2763.
Hochreiter, S. and Schmidhuber, J. (1997). Long short-term
memory. Neural computation, 9(8):1735–1780.
Huet, G. (2003). Towards computational processing of san-
skrit. In International Conference on Natural Lan-
guage Processing (ICON). CiteSeer.
Huet, G. (2005). A functional toolkit for morphological and
phonological processing, application to a sanskrit tag-
ger. Journal of Functional Programming, 15(4):573–
614.
Krishna, A., Santra, B., Satuluri, P., Bandaru, S. P., Faldu,
B., Singh, Y., and Goyal, P. (2016). Word seg-
mentation in sanskrit using path constrained random
walks. In Proceedings of COLING 2016, the 26th
International Conference on Computational Linguis-
tics: Technical Papers, pages 494–504.
Kulkarni, A. and Shukl, D. (2009). Sanskrit morphologi-
cal analyser: Some issues. Indian Linguistics, 70(1-
4):169–177.
Kumar, A., Mittal, V., and Kulkarni, A. (2010). Sanskrit
compound processor. In Sanskrit Computational Lin-
guistics: 4th International Symposium, pages 57–69,
New Delhi, India. Springer.
Kumar, S. (2007). Sandhi splitter and analyzer for sanskrit
(with reference to ac sandhi). Submitted, 2007.
Learn Sanskrit (2024). Sanscript: Sanskrit transliteration.
Accessed: 2024-08-08.
Linguistics, S. C. (2002-24). Sanskrit computational lin-
guistics.
Mittal, V. (2010). Automatic sanskrit segmentizer using fi-
nite state transducers. In Proceedings of the ACL 2010
Student Research Workshop, pages 85–90.
Natarajan, A. and Charniak, E. (2011). s3-statistical sandhi
splitting. In Proceedings of 5th international joint
conference on natural language processing, pages
301–308.
Patankar, M. P. S. (2023). Exploring the intricacies of
sandhi in sanskrit: phonological rules and linguistic
ABBIE: Attention-Based BI-Encoders for Predicting Where to Split Compound Sanskrit Words
343