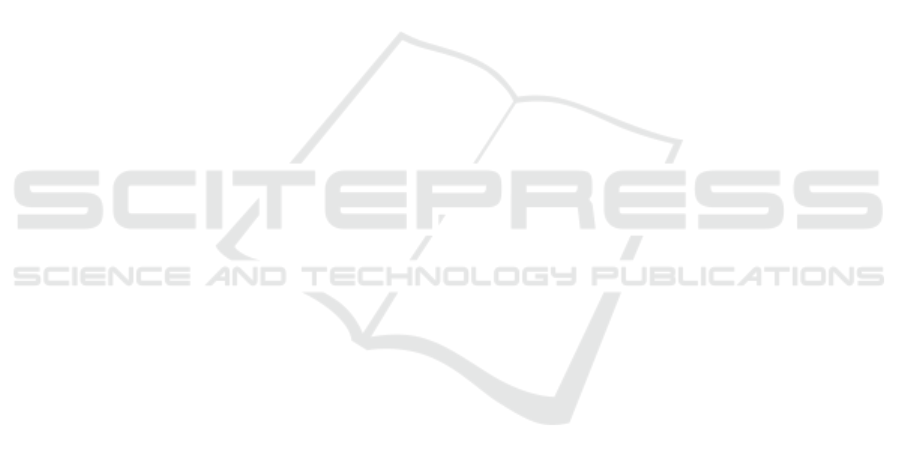
REFERENCES
Arulkumaran, K., Deisenroth, M. P., Brundage, M., and
Bharath, A. A. (2017). Deep reinforcement learning:
A brief survey. IEEE Signal Processing Magazine,
34(6):26–38.
Azar, A. T., Elshazly, H. I., Hassanien, A. E., and Elko-
rany, A. M. (2014). A random forest classifier for
lymph diseases. Computer methods and programs in
biomedicine, 113(2):465–473.
Chen, Z., Tan, S., Nori, H., Inkpen, K., Lou, Y., and
Caruana, R. (2021). Using explainable boosting ma-
chines (ebms) to detect common flaws in data. In
Joint European Conference on Machine Learning and
Knowledge Discovery in Databases, pages 534–551.
Springer.
Fern
´
andez, A., Garcia, S., Herrera, F., and Chawla, N. V.
(2018). Smote for learning from imbalanced data:
progress and challenges, marking the 15-year an-
niversary. Journal of artificial intelligence research,
61:863–905.
Garreau, D. and Luxburg, U. (2020). Explaining the ex-
plainer: A first theoretical analysis of lime. In Interna-
tional conference on artificial intelligence and statis-
tics, pages 1287–1296. PMLR.
Guidotti, R., Monreale, A., Ruggieri, S., Turini, F., Gian-
notti, F., and Pedreschi, D. (2018). A survey of meth-
ods for explaining black box models. ACM computing
surveys (CSUR), 51(5):1–42.
Integraal Kankercentrum Nederland (2020). Integraal
kankercentrum nederland (iknl). Accessed: 2024-07-
26.
Integraal Kankercentrum Nederland (2021). Synthetic
dataset. Accessed: 2024-07-26.
Jim
´
enez-Luna, J., Grisoni, F., and Schneider, G. (2020).
Drug discovery with explainable artificial intelligence.
Nature Machine Intelligence, 2(10):573–584.
Kumar, I. E., Venkatasubramanian, S., Scheidegger, C., and
Friedler, S. (2020). Problems with shapley-value-
based explanations as feature importance measures.
Lundberg, S. M. and Lee, S.-I. (2017). A unified approach
to interpreting model predictions. Advances in neural
information processing systems, 30.
Mellem, M. S., Kollada, M., Tiller, J., and Lauritzen, T.
(2021). Explainable ai enables clinical trial patient se-
lection to retrospectively improve treatment effects in
schizophrenia. BMC medical informatics and decision
making, 21(1):162.
Meng, Y., Yang, N., Qian, Z., and Zhang, G. (2020). What
makes an online review more helpful: an interpreta-
tion framework using xgboost and shap values. Jour-
nal of Theoretical and Applied Electronic Commerce
Research, 16(3):466–490.
Messalas, A., Kanellopoulos, Y., and Makris, C. (2019).
Model-agnostic interpretability with shapley values.
In 2019 10th International Conference on Informa-
tion, Intelligence, Systems and Applications (IISA),
pages 1–7. IEEE.
Murray, N., Winstanley, J., Bennett, A., and Francis, K.
(2009). Diagnosis and treatment of advanced breast
cancer: summary of nice guidance. Bmj, 338.
Oeffinger, K. C., Fontham, E. T., Etzioni, R., Herzig,
A., Michaelson, J. S., Shih, Y.-C. T., Walter, L. C.,
Church, T. R., Flowers, C. R., LaMonte, S. J., et al.
(2015). Breast cancer screening for women at average
risk: 2015 guideline update from the american cancer
society. Jama, 314(15):1599–1614.
Parmar, A., Katariya, R., and Patel, V. (2019). A review
on random forest: An ensemble classifier. In Inter-
national conference on intelligent data communica-
tion technologies and internet of things (ICICI) 2018,
pages 758–763. Springer.
Ribeiro, M. T., Singh, S., and Guestrin, C. (2016). ”why
should i trust you?”: Explaining the predictions of any
classifier.
Samek, W., Binder, A., Montavon, G., Lapuschkin, S., and
M
¨
uller, K.-R. (2016). Evaluating the visualization
of what a deep neural network has learned. IEEE
transactions on neural networks and learning systems,
28(11):2660–2673.
Scanagatta, M., Salmer
´
on, A., and Stella, F. (2019). A sur-
vey on bayesian network structure learning from data.
Progress in Artificial Intelligence, 8(4):425–439.
Shah, S. I. H., Alam, S., Ghauri, S. A., Hussain, A., and
Ansari, F. A. (2019). A novel hybrid cuckoo search-
extreme learning machine approach for modulation
classification. IEEE Access, 7:90525–90537.
Shah, S. I. H., De Pietro, G., Paragliola, G., and Coro-
nato, A. (2023). Projection based inverse reinforce-
ment learning for the analysis of dynamic treatment
regimes. Applied Intelligence, 53(11):14072–14084.
ˇ
Strumbelj, E. and Kononenko, I. (2014). Explaining pre-
diction models and individual predictions with feature
contributions. Knowledge and information systems,
41:647–665.
Velmurugan, M., Ouyang, C., Moreira, C., and Sindhgatta,
R. (2021). Evaluating fidelity of explainable methods
for predictive process analytics. In International con-
ference on advanced information systems engineer-
ing, pages 64–72. Springer.
Xu, F., Uszkoreit, H., Du, Y., Fan, W., Zhao, D., and Zhu,
J. (2019). Explainable ai: A brief survey on his-
tory, research areas, approaches and challenges. In
Natural language processing and Chinese computing:
8th cCF international conference, NLPCC 2019, dun-
huang, China, October 9–14, 2019, proceedings, part
II 8, pages 563–574. Springer.
Zhang, Y., Weng, Y., and Lund, J. (2022). Applications
of explainable artificial intelligence in diagnosis and
surgery. Diagnostics, 12(2):237.
Can We Trust Explanation! Evaluation of Model-Agnostic Explanation Techniques on Highly Imbalanced, Multiclass-Multioutput
Classification Problem
539