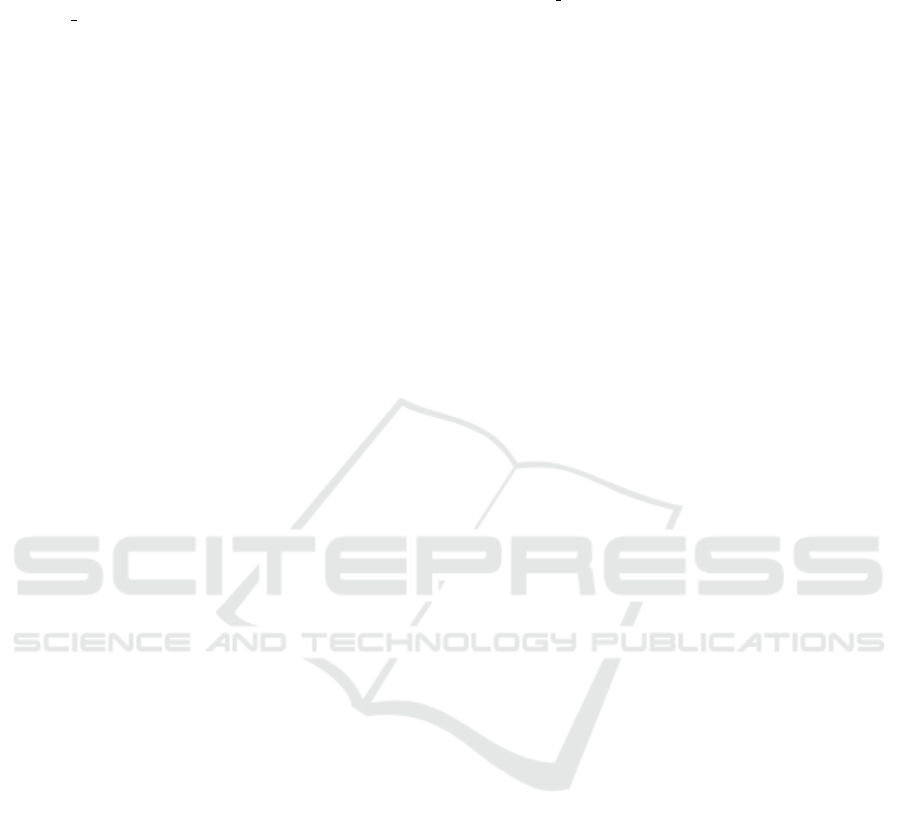
Interpretation Table retrieved from Research-
Gate: https://www.researchgate.net/figure/
Criteria-for-the-Interpretation-of-Kappa-values-by-Landis-Koch-1977
tbl1 259976499.
Larrimore, L., Jiang, L., Larrimore, J., Markowitz, D. M.,
and Gorski, S. (2011). Peer to peer lending: The re-
lationship between language features, trustworthiness,
and persuasion success. Journal of Applied Commu-
nication Research, 39:19–37.
Latif, S., Usama, M., Malik, M. I., and Schuller, B. (2023).
Can large language models aid in annotating speech
emotional data? uncovering new frontiers. ArXiv,
abs/2307.06090.
Martin, W. C. (2017). Positive versus negative word-of-
mouth: Effects on receivers. Academy of Marketing
Studies Journal, 21:1.
McGraw, K. O. and Wong, S. P. (1996). Forming infer-
ences about some intraclass correlation coefficients.
In Psychological Methods, volume 1, page 30. Amer-
ican Psychological Association.
Melloni, G., Caglio, A., and Perego, P. (2017). Saying more
with less? disclosure conciseness, completeness and
balance in integrated reports. In SRPN: Corporate Re-
porting (Topic).
Morrison, H. J. and Lorusso, J. R. (2023). Develop-
ing teacher candidates’ professional advocacy skills
through persuasive storytelling. In Journal of Physical
Education, Recreation & Dance, volume 94, pages 6
– 11.
Natasia, G. and Angelianawati, L. (2022). Students’ percep-
tion of using storytelling technique to improve speak-
ing performance at smpn 143 jakarta utara. JET (Jour-
nal of English Teaching).
Nguyen, A.-T., Chen, W., and Rauterberg, G. (2012). On-
line feedback system for public speakers. In 2012
IEEE Symposium on E-Learning, E-Management and
E-Services, pages 1–5.
OpenAI (2024a). Gpt-4o-mini: A commercial large lan-
guage model for public speaking analysis. https:
//openai.com. Accessed: 2024-10-21.
OpenAI (2024b). Gpt-4o-mini: Advancing cost-efficient
intelligence.
Ortony, A., editor (1993). Metaphor and Thought. Cam-
bridge University Press, 2nd edition.
Paranjape, B., Michael, J., Ghazvininejad, M., Zettlemoyer,
L., and Hajishirzi, H. (2021). Prompting contrastive
explanations for commonsense reasoning tasks. In
Findings.
Park, S., Shim, H. S., Chatterjee, M., Sagae, K., and
Morency, L.-P. (2014). Computational analysis of per-
suasiveness in social multimedia: A novel dataset and
multimodal prediction approach. In Proceedings of
the 16th International Conference on Multimodal In-
teraction, pages 50–57, New York, NY, USA. Associ-
ation for Computing Machinery.
Patterson, D. A., Gonzalez, J., Le, Q. V., Liang, C.,
Mungu
´
ıa, L.-M., Rothchild, D., So, D. R., Texier, M.,
and Dean, J. (2021). Carbon emissions and large neu-
ral network training. ArXiv, abs/2104.10350.
Pennebaker, J., Boyd, R., Booth, R., Ashokkumar, A.,
and Francis, M. (2022). Linguistic inquiry and word
count: Liwc-22. In Pennebaker Conglomerates.
Piolat, A., Booth, R., Chung, C., Davids, M., and Pen-
nebaker, J. (2011). La version franc¸aise du diction-
naire pour le liwc : Modalit
´
es de construction et ex-
emples d’utilisation. In Psychologie Franc¸aise, vol-
ume 56, pages 145–159.
Radford, A., Kim, J. W., Xu, T., Brockman, G., McLeavey,
C., and Sutskever, I. (2022). Robust speech recogni-
tion via large-scale weak supervision.
Rodero, E. and Larrea, O. (2022). Virtual reality with dis-
tractors to overcome public speaking anxiety in uni-
versity students.
Schneider, J., B
¨
orner, D., van Rosmalen, P., and Specht,
M. M. (2015). Presentation trainer, your public speak-
ing multimodal coach. Proceedings of the 2015 ACM
on International Conference on Multimodal Interac-
tion.
Schreiber, L. and Hartranft, M. (2017). Introduction to pub-
lic speaking. In Rice, T. S., editor, Fundamentals of
Public Speaking. College of the Canyons, California.
Schreiber, L. M., Paul, G. D., and Shibley, L. R. (2012).
The development and test of the public speaking com-
petence rubric. Communication Education, 61:205 –
233.
Song, W., Wu, B., Zheng, C., and Zhang, H. (2023). De-
tection of public speaking anxiety: A new dataset and
algorithm. In 2023 IEEE International Conference on
Multimedia and Expo (ICME), pages 2633–2638.
Touvron, H., Martin, L., Stone, K., Albert, P., et al. (2023).
Llama 2: Open foundation and fine-tuned chat mod-
els.
Tun, S. S. Y., Okada, S., Huang, H.-H., and Leong, C. W.
(2023). Multimodal transfer learning for oral presen-
tation assessment. IEEE Access, 11:84013–84026.
Webson, A. and Pavlick, E. (2022). Do prompt-based mod-
els really understand the meaning of their prompts? In
Carpuat, M., de Marneffe, M.-C., and Ruiz, I. V. M.,
editors, Proceedings of the 2022 Conference of the
North American Chapter of the Association for Com-
putational Linguistics: Human Language Technolo-
gies, pages 2300–2344, Seattle, United States. Asso-
ciation for Computational Linguistics.
Wei, J., Wang, X., Schuurmans, D., Bosma, M., Ichter, B.,
Xia, F., Chi, E., Le, Q., and Zhou, D. (2023). Chain-
of-thought prompting elicits reasoning in large lan-
guage models.
Xu, P., Ping, W., Wu, X., McAfee, L. C., Zhu, C., Liu,
Z., Subramanian, S., Bakhturina, E., Shoeybi, M., and
Catanzaro, B. (2023). Retrieval meets long context
large language models. ArXiv, abs/2310.03025.
Yang, Z., Huynh, J., Tabata, R., Cestero, N., Aharoni, T.,
and Hirschberg, J. (2020). What makes a speaker
charismatic? producing and perceiving charismatic
speech. In Speech Prosody 2020.
Zhao, T. Z., Wallace, E., Feng, S., et al. (2021). Calibrate
before use: Improving few-shot performance of lan-
guage models.
Zhu, Y., Zhang, P., Haq, E.-U., Hui, P., and Tyson, G.
(2023). Can chatgpt reproduce human-generated la-
Decoding Persuasiveness in Eloquence Competitions: An Investigation into the LLM’s Ability to Assess Public Speaking
545