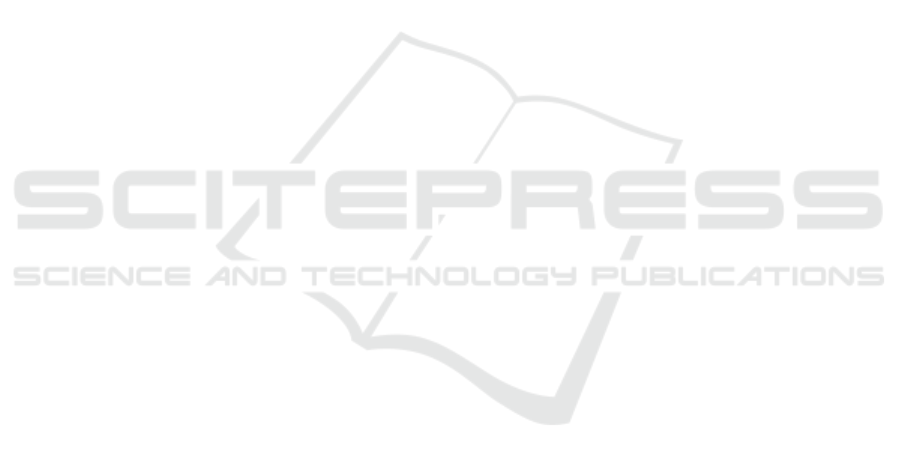
ACKNOWLEDGMENTS
This work was partially supported by Proyecto
CONAHCYT: “Procesos de Decisi
´
on de Markov en
ambiente difuso”, Ciencia de Frontera 2023, CF-
2023-I-1362.
REFERENCES
Carrero-Vera, K., Cruz-Su
´
arez, H., and Montes-de Oca, R.
(2020). Finite-horizon and infinite-horizon Markov
decision processes with trapezoidal fuzzy discounted
rewards. In International Conference on Operations
Research and Enterprise Systems, pages 171–192.
Springer.
Carrero-Vera, K., Cruz-Su
´
arez, H., and Montes-de Oca, R.
(2022). Markov decision processes on finite spaces
with fuzzy total rewards. Kybernetika (Prague),
58(2):180–199.
Cruz-Su
´
arez, H., Montes de Oca, R., and Ortega Guti
´
errez,
R. (2023a). Deterministic discounted Markov deci-
sion processes with fuzzy rewards/costs. Fuzzy Infor-
mation and Engineering, 15(3):274–290.
Cruz-Su
´
arez, H., Montes-de Oca, R., and Ortega-Guti
´
errez,
R. (2023b). An extended version of average Markov
decision processes on discrete spaces under fuzzy en-
vironment. Kybernetika (Prague), 59(1):160–178.
Diamond, P. and Kloeden, P. (1994). Metric Spaces of
Fuzzy Sets: Theory and Applications. WORLD SCI-
ENTIFIC.
Furukawa, N. (1997). Paramentric orders on fuzzy numbers
and their roles in fuzzy optimization problems. Opti-
mization, 40(2):171–192.
Gittins, J. and Jones, D. (1974). A dynamic allocation index
for the sequential design of experiments. Progress in
Statistics (edited by J. Gani), 241–266.
Gittins, J. C. (2018). Bandit Processes and Dynamic Alloca-
tion Indices. Journal of the Royal Statistical Society:
Series B (Methodological), 41(2):148–164.
Kaspi, H. and Mandelbaum, A. (1998). Multi-armed ban-
dits in discrete and continuous time. The Annals of
Applied Probability, 8(4):1270–1290.
Kurano, M., Song, J., Hosaka, M., and Huang, Y. (1998).
Controlled Markov set-chains with discounting. Jour-
nal of applied probability, 35(2):293–302.
Kurano, M., Yasuda, M., Nakagami, J.-i., and Yoshida, Y.
(1996). Markov-type fuzzy decision processes with
a discounted reward on a closed interval. European
Journal of Operational Research, 92(3):649–662.
Kurano, M., Yasuda, M., Nakagami, J.-i., and Yoshida, Y.
(2003). Markov decision processes with fuzzy re-
wards. Journal of Nonlinear and Convex Analysis,
4(1):105–116.
Mart
´
ınez-Cort
´
es, V. M. (2021). Bi-personal stochastic tran-
sient Markov games with stopping times and total re-
ward criterion. Kybernetika, 57(1):1–14.
Pahade, J. K. and Jha, M. (2021). Credibilistic vari-
ance and skewness of trapezoidal fuzzy variable and
mean–variance–skewness model for portfolio selec-
tion. Results in Applied Mathematics, 11:100159.
Puri, M. L. and Ralescu, D. A. (1986). Fuzzy random vari-
ables. Journal of Mathematical Analysis and Applica-
tions, 114(2):409–422.
Puterman, M. L. (2014). Markov decision processes: dis-
crete stochastic dynamic programming. John Wiley &
Sons.
Raj, M. E. A., Sivaraman, G., and Vishnukumar, P. (2023).
A novel kind of arithmetic operations on trapezoidal
fuzzy numbers and its applications to optimize the
transportation cost. International Journal of Fuzzy
Systems, 25(3):1069–1076.
Rezvani, S. and Molani, M. (2014). Representation of trape-
zoidal fuzzy numbers with shape function. Ann. Fuzzy
Math. Inform, 8(1):89–112.
Semmouri, A., Jourhmane, M., and Belhallaj, Z. (2020).
Discounted Markov decision processes with fuzzy
costs. Annals of Operations Research, 295:769–786.
Thompson, W. R. (1933). On the likelihood that one un-
known probability exceeds another in view of the evi-
dence of two samples. Biometrika, 25(3-4):285–294.
Zadeh, L. (1965). Fuzzy sets. Information and Control,
8(3):338–353.
Fuzzy Rewards on the Multi-Armed Bandits Model
277