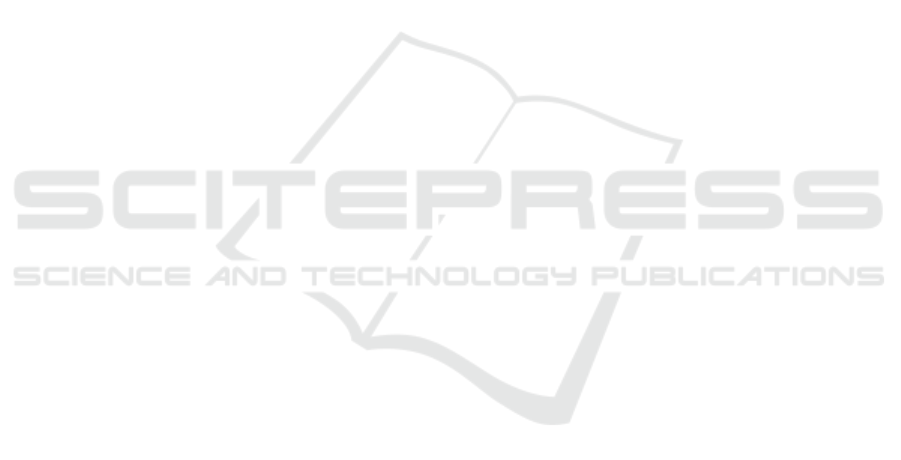
REFERENCES
Agrawal, P., Antoniak, S., Hanna, E. B., Bout, B., Chap-
lot, D., Chudnovsky, J., Costa, D., De Monicault, B.,
Garg, S., Gervet, T., et al. (2024). Pixtral 12b. arXiv
preprint arXiv:2410.07073.
Anzer, G., Bauer, P., Brefeld, U., and Faßmeyer, D. (2022).
Detection of tactical patterns using semi-supervised
graph neural networks. In 16th MIT Sloan Sports An-
alytics Conference.
Azar, S. M., Atigh, M. G., Nickabadi, A., and Alahi, A.
(2019). Convolutional relational machine for group
activity recognition. In Proceedings of the IEEE/CVF
Conference on Computer Vision and Pattern Recogni-
tion, pages 7892–7901.
Bagautdinov, T., Alahi, A., Fleuret, F., Fua, P., and
Savarese, S. (2017). Social scene understanding: End-
to-end multi-person action localization and collective
activity recognition. In Proceedings of the IEEE con-
ference on computer vision and pattern recognition,
pages 4315–4324.
Brefeld, U., Lasek, J., and Mair, S. (2019). Probabilis-
tic movement models and zones of control. Machine
Learning, 108(1):127–147.
Cartas, A., Ballester, C., and Haro, G. (2022). A graph-
based method for soccer action spotting using unsu-
pervised player classification. In Proceedings of the
5th International ACM Workshop on Multimedia Con-
tent Analysis in Sports, pages 93–102.
Chen, S., Sun, P., Xie, E., Ge, C., Wu, J., Ma, L., Shen, J.,
and Luo, P. (2021). Watch only once: An end-to-end
video action detection framework. In Proceedings of
the IEEE/CVF International Conference on Computer
Vision, pages 8178–8187.
Cioppa, A., Giancola, S., Somers, V., Magera, F., Zhou,
X., Mkhallati, H., Deli
`
ege, A., Held, J., Hinojosa, C.,
Mansourian, A. M., et al. (2024). Soccernet 2023
challenges results. Sports Engineering, 27(2):24.
Deitke, M., Clark, C., Lee, S., Tripathi, R., Yang, Y., Park,
J. S., Salehi, M., Muennighoff, N., Lo, K., Soldaini,
L., et al. (2024). Molmo and pixmo: Open weights
and open data for state-of-the-art multimodal models.
arXiv preprint arXiv:2409.17146.
Dick, U., Link, D., and Brefeld, U. (2022). Who can re-
ceive the pass? a computational model for quantify-
ing availability in soccer. Data mining and knowledge
discovery, 36(3):987–1014.
Ding, D. and Huang, H. H. (2020). A graph attention
based approach for trajectory prediction in multi-
agent sports games. arXiv preprint arXiv:2012.10531.
Everett, G., Beal, R. J., Matthews, T., Early, J., Norman,
T. J., and Ramchurn, S. D. (2023). Inferring player
location in sports matches: Multi-agent spatial im-
putation from limited observations. arXiv preprint
arXiv:2302.06569.
Everingham, M., Van Gool, L., Williams, C. K., Winn, J.,
and Zisserman, A. (2010). The pascal visual object
classes (voc) challenge. International journal of com-
puter vision, 88:303–338.
Fadel, S. G., Mair, S., da Silva Torres, R., and Brefeld, U.
(2023). Contextual movement models based on nor-
malizing flows. AStA Advances in Statistical Analysis,
107(1):51–72.
Feichtenhofer, C. (2020). X3d: Expanding architectures
for efficient video recognition. In Proceedings of the
IEEE/CVF conference on computer vision and pattern
recognition, pages 203–213.
Feichtenhofer, C., Fan, H., Malik, J., and He, K. (2019).
Slowfast networks for video recognition. In Proceed-
ings of the IEEE/CVF international conference on
computer vision, pages 6202–6211.
Gavrilyuk, K., Sanford, R., Javan, M., and Snoek, C. G.
(2020). Actor-transformers for group activity recogni-
tion. In Proceedings of the IEEE/CVF conference on
computer vision and pattern recognition, pages 839–
848.
Girdhar, R., Carreira, J., Doersch, C., and Zisserman, A.
(2018). A better baseline for AVA. arXiv preprint
arXiv:1807.10066.
Girdhar, R., Carreira, J., Doersch, C., and Zisserman, A.
(2019). Video action transformer network. In Pro-
ceedings of the IEEE/CVF conference on computer vi-
sion and pattern recognition, pages 244–253.
Gu, C., Sun, C., Ross, D. A., Vondrick, C., Pantofaru, C.,
Li, Y., Vijayanarasimhan, S., Toderici, G., Ricco, S.,
Sukthankar, R., et al. (2018). Ava: A video dataset
of spatio-temporally localized atomic visual actions.
In Proceedings of the IEEE conference on computer
vision and pattern recognition, pages 6047–6056.
He, K., Gkioxari, G., Doll
´
ar, P., and Girshick, R. (2017).
Mask r-CNN. In Proceedings of the IEEE interna-
tional conference on computer vision, pages 2961–
2969.
Hong, J., Zhang, H., Gharbi, M., Fisher, M., and Fatahalian,
K. (2022). Spotting temporally precise, fine-grained
events in video. In European Conference on Computer
Vision, pages 33–51. Springer.
Jha, D., Rauniyar, A., Johansen, H. D., Johansen, D.,
Riegler, M. A., Halvorsen, P., and Bagci, U. (2022).
Video analytics in elite soccer: A distributed com-
puting perspective. In 2022 IEEE 12th Sensor Ar-
ray and Multichannel Signal Processing Workshop
(SAM), pages 221–225. IEEE.
Kalogeiton, V., Weinzaepfel, P., Ferrari, V., and Schmid,
C. (2017). Action tubelet detector for spatio-temporal
action localization. In Proceedings of the IEEE inter-
national conference on computer vision, pages 4405–
4413.
Kingma, D. and Ba, J. (2015). Adam: A method for
stochastic optimization. In International Conference
on Learning Representations (ICLR), San Diega, CA,
USA.
K
¨
op
¨
ukl
¨
u, O., Wei, X., and Rigoll, G. (2019). You only
watch once: A unified cnn architecture for real-time
spatiotemporal action localization. arXiv preprint
arXiv:1911.06644.
Li, Y., Chen, L., He, R., Wang, Z., Wu, G., and Wang,
L. (2021). Multisports: A multi-person video dataset
Game State and Spatio-Temporal Action Detection in Soccer Using Graph Neural Networks and 3D Convolutional Networks
645