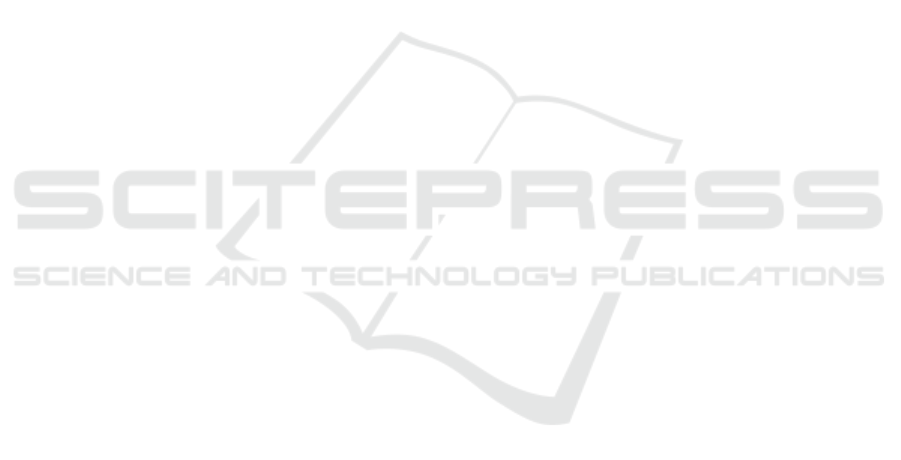
Dee, W., Ibrahim, R. A., and Marouli, E. (2023).
Histopathological Domain Adaptation with Genera-
tive Adversarial Networks Bridging the Domain Gap
Between Thyroid Cancer Histopathology Datasets.
Dimitrakopoulos, P., Sfikas, G., and Nikou, C. (2020).
ISING-GAN: Annotated Data Augmentation with a
Spatially Constrained Generative Adversarial Net-
work. In 2020 IEEE 17th International Symposium
on Biomedical Imaging (ISBI), pages 1600–1603.
Edlund, C., Jackson, T. R., Khalid, N., Bevan, N., Dale,
T., Dengel, A., Ahmed, S., Trygg, J., and Sj
¨
ogren,
R. (2021). LIVECell—A large-scale dataset for
label-free live cell segmentation. Nature Methods,
18(9):1038–1045.
Goodfellow, I., Pouget-Abadie, J., Mirza, M., Xu, B.,
Warde-Farley, D., Ozair, S., Courville, A., and Ben-
gio, Y. (2014). Generative Adversarial Nets. In
Ghahramani, Z., Welling, M., Cortes, C., Lawrence,
N., and Weinberger, K. Q., editors, Advances in Neu-
ral Information Processing Systems, volume 27. Cur-
ran Associates, Inc.
Greenwald, N. F., Miller, G., Moen, E., Kong, A., Kagel,
A., Dougherty, T., Fullaway, C. C., McIntosh, B. J.,
Leow, K. X., Schwartz, M. S., Pavelchek, C., Cui,
S., Camplisson, I., Bar-Tal, O., Singh, J., Fong, M.,
Chaudhry, G., Abraham, Z., Moseley, J., Warshawsky,
S., Soon, E., Greenbaum, S., Risom, T., Hollmann,
T., Bendall, S. C., Keren, L., Graf, W., Angelo, M.,
and Van Valen, D. (2022). Whole-cell segmentation
of tissue images with human-level performance using
large-scale data annotation and deep learning. Nature
Biotechnology, 40(4):555–565.
Han, L., Murphy, R. F., and Ramanan, D. (2018). Learn-
ing Generative Models of Tissue Organization with
Supervised GANs. In IEEE Winter Conference on
Applications of Computer Vision. IEEE Winter Con-
ference on Applications of Computer Vision, volume
2018, pages 682–690.
Heusel, M., Ramsauer, H., Unterthiner, T., Nessler, B., and
Hochreiter, S. (2017). GANs Trained by a Two Time-
Scale Update Rule Converge to a Local Nash Equilib-
rium. In Advances in Neural Information Processing
Systems, volume 30. Curran Associates, Inc.
Jiang, L., Dai, B., Wu, W., and Loy, C. C. (2021). Deceive
D: Adaptive Pseudo Augmentation for GAN Training
with Limited Data. In Advances in Neural Information
Processing Systems, volume 34, pages 21655–21667.
Curran Associates, Inc.
Kang, M., Shim, W., Cho, M., and Park, J. (2021). Reboot-
ing ACGAN: Auxiliary Classifier GANs with Stable
Training. In Advances in Neural Information Process-
ing Systems, volume 34, pages 23505–23518. Curran
Associates, Inc.
Kang, M., Shin, J., and Park, J. (2023). StudioGAN: A Tax-
onomy and Benchmark of GANs for Image Synthesis.
IEEE Transactions on Pattern Analysis and Machine
Intelligence, 45(12):15725–15742.
Karras, T., Aittala, M., Hellsten, J., Laine, S., Lehtinen, J.,
and Aila, T. (2020a). Training Generative Adversar-
ial Networks with Limited Data. In Advances in Neu-
ral Information Processing Systems, volume 33, pages
12104–12114. Curran Associates, Inc.
Karras, T., Laine, S., Aittala, M., Hellsten, J., Lehtinen,
J., and Aila, T. (2020b). Analyzing and improving
the image quality of stylegan. In Proceedings of the
IEEE/CVF Conference on Computer Vision and Pat-
tern Recognition, pages 8110–8119. IEEE Computer
Society.
Kingma, D. P. and Welling, M. (2014). Auto-encoding vari-
ational bayes. In Bengio, Y. and LeCun, Y., editors,
2nd International Conference on Learning Represen-
tations, ICLR 2014, Banff, AB, Canada, April 14-16,
2014, Conference Track Proceedings.
Lesmes-Leon, D. N., Dengel, A., and Ahmed, S. (2023).
Generative adversarial networks in cell microscopy
for image augmentation. A systematic review.
Lin, T., Maire, M., Belongie, S. J., Bourdev, L. D., Girshick,
R. B., Hays, J., Perona, P., Ramanan, D., Doll’a r, P.,
and Zitnick, C. L. (2014). Microsoft COCO: common
objects in context. CoRR, abs/1405.0312.
Mascolini, A., Cardamone, D., Ponzio, F., Di Cataldo, S.,
and Ficarra, E. (2022). Exploiting generative self-
supervised learning for the assessment of biological
images with lack of annotations. BMC Bioinformat-
ics, 23(1):295.
Nichol, A. Q. and Dhariwal, P. (2021). Improved Denoising
Diffusion Probabilistic Models. In Proceedings of the
38th International Conference on Machine Learning,
pages 8162–8171. PMLR.
Odena, A., Olah, C., and Shlens, J. (2017). Conditional
Image Synthesis with Auxiliary Classifier GANs. In
Proceedings of the 34th International Conference on
Machine Learning, pages 2642–2651. PMLR.
Shaga Devan, K., Walther, P., von Einem, J., Ropinski, T.,
A. Kestler, H., and Read, C. (2021). Improved auto-
matic detection of herpesvirus secondary envelopment
stages in electron microscopy by augmenting train-
ing data with synthetic labelled images generated by
a generative adversarial network. Cellular Microbiol-
ogy, 23(2):e13280.
Sharma, R., Saqib, M., Lin, C. T., and Blumenstein, M.
(2022). A Survey on Object Instance Segmentation.
SN Computer Science, 3(6):499.
Szegedy, C., Vanhoucke, V., Ioffe, S., Shlens, J., and Wojna,
Z. (2016). Rethinking the Inception Architecture for
Computer Vision. In 2016 IEEE Conference on Com-
puter Vision and Pattern Recognition (CVPR), pages
2818–2826.
Toker, A., Eisenberger, M., Cremers, D., and Leal-Taix
´
e,
L. (2024). SatSynth: Augmenting Image-Mask Pairs
through Diffusion Models for Aerial Semantic Seg-
mentation.
Wiatrak, M., Albrecht, S. V., and Nystrom, A. (2020). Sta-
bilizing Generative Adversarial Networks: A Survey.
Zhao, S., Liu, Z., Lin, J., Zhu, J.-Y., and Han, S. (2020).
Differentiable Augmentation for Data-Efficient GAN
Training. In Advances in Neural Information Pro-
cessing Systems, volume 33, pages 7559–7570. Cur-
ran Associates, Inc.
Synthesizing Annotated Cell Microscopy Images with Generative Adversarial Networks
599