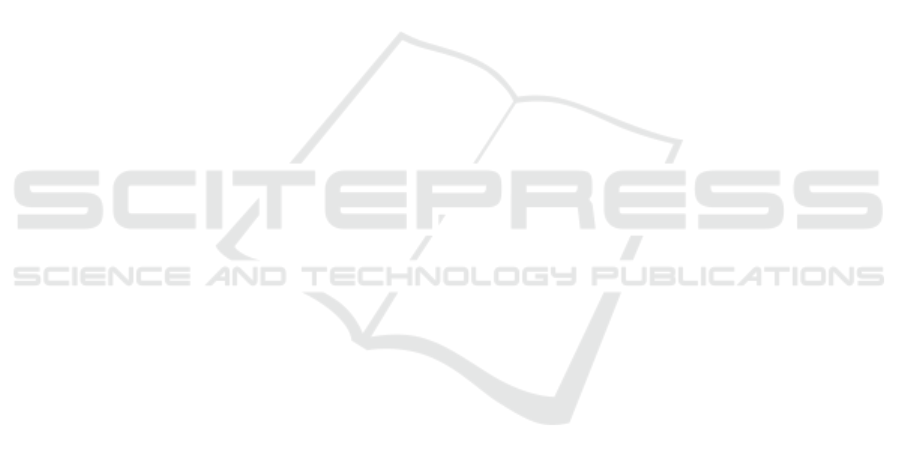
Fan, W., Sallay, H., and Bouguila, N. (2017). Online
learning of hierarchical pitman–yor process mixture
of generalized dirichlet distributions with feature se-
lection. IEEE Transactions on Neural Networks and
Learning Systems, 28(9):2048–2061.
Fang, K. W. (2018). Symmetric multivariate and related
distributions. CRC Press.
Forouzanfar, D., Manouchehri, N., and Bouguila, N.
(2023a). Bayesian inference in infinite multivari-
ate mcdonald’s beta mixture model. In Rutkowski,
L., Scherer, R., Korytkowski, M., Pedrycz, W.,
Tadeusiewicz, R., and Zurada, J. M., editors, Artificial
Intelligence and Soft Computing - 22nd International
Conference, ICAISC 2023, Zakopane, Poland, June
18-22, 2023, Proceedings, Part II, volume 14126 of
Lecture Notes in Computer Science, pages 320–330.
Springer.
Forouzanfar, D., Manouchehri, N., and Bouguila, N.
(2023b). Finite multivariate mcdonald’s beta mix-
ture model learning approach in medical applica-
tions. In Hong, J., Lanperne, M., Park, J. W., Cern
´
y,
T., and Shahriar, H., editors, Proceedings of the
38th ACM/SIGAPP Symposium on Applied Comput-
ing, SAC 2023, Tallinn, Estonia, March 27-31, 2023,
pages 1143–1150. ACM.
Forouzanfar, D., Manouchehri, N., and Bouguila, N.
(2023c). A fully bayesian inference approach for mul-
tivariate mcdonald’s beta mixture model with feature
selection. In 9th International Conference on Control,
Decision and Information Technologies, CoDIT 2023,
Rome, Italy, July 3-6, 2023, pages 2055–2060. IEEE.
Golan, I. and El-Yaniv, R. (2018). Deep anomaly detec-
tion using geometric transformations. In Advances
in Neural Information Processing Systems (NeurIPS),
volume 31.
Hosseinzadeh, M., Rahmani, A. M., Vo, B., Bidaki, M.,
Masdari, M., and Zangakani, M. (2021). Improving
security using svm-based anomaly detection: issues
and challenges. Soft Computing, 25:3195–3223.
Hu, C., Fan, W., Du, J., and Bouguila, N. (2019). A novel
statistical approach for clustering positive data based
on finite inverted beta-liouville mixture models. Neu-
rocomputing, 333:110–123.
Liu, F. T., Ting, K. M., and Zhou, Z.-H. (2008). Isolation
forest. In 2008 Eighth IEEE International Conference
on Data Mining, pages 413–422.
Manoj, C., Wijekoon, P., and Yapa, R. D. (2013). The mc-
donald generalized beta-binomial distribution: A new
binomial mixture distribution and simulation based
comparison with its nested distributions in handling
overdispersion. International Journal of Statistics and
Probability, 2(2):24.
Nedaie, A. and Najafi, A. A. (2018). Support vector ma-
chine with dirichlet feature mapping. Neural Net-
works, 98:87–101.
Nguyen, H., Azam, M., and Bouguila, N. (2019). Data
clustering using variational learning of finite scaled
dirichlet mixture models. In 2019 IEEE 28th Inter-
national Symposium on Industrial Electronics (ISIE),
pages 1391–1396.
Oboh, B. S. and Bouguila, N. (2017). Unsupervised learn-
ing of finite mixtures using scaled dirichlet distribu-
tion and its application to software modules catego-
rization. In 2017 IEEE International Conference on
Industrial Technology (ICIT), pages 1085–1090.
Qiu, C., Pfrommer, T., Kloft, M., Mandt, S., and Rudolph,
M. (2021). Neural transformation learning for deep
anomaly detection beyond images. In Proceedings of
the International Conference on Machine Learning,
pages 8703–8714. PMLR.
Rahman, M. H. and Bouguila, N. (2021). Efficient feature
mapping in classifying proportional data. IEEE Ac-
cess, 9:3712–3724.
Scholkopf, B. and Smola, A. J. (2002). Learning with Ker-
nels: Support Vector Machines, Regularization, Opti-
mization, and Beyond. MIT Press, Cambridge, MA.
Sefidpour, A. and Bouguila, N. (2012). Spatial color im-
age segmentation based on finite non-gaussian mix-
ture models. Expert Syst. Appl., 39(10):8993–9001.
Sghaier, O., Amayri, M., and Bouguila, N. (2023). Mul-
tivariate beta normality scores approach for deep
anomaly detection in images using transformations.
In 2023 IEEE International Conference on Systems,
Man, and Cybernetics (SMC), pages 3428–3433.
Sghaier, O., Amayri, M., and Bouguila, N. (2024).
Libby-novick beta-liouville distribution for enhanced
anomaly detection in proportional data. ACM Trans.
Intell. Syst. Technol., 15(5):107:1–107:26.
Topham, L. K., Khan, W., Al-Jumeily, D., Waraich, A., and
Hussain, A. J. (2022). Gait identification using limb
joint movement and deep machine learning. IEEE Ac-
cess, 10:100113–100127.
Wong, T. T. (1998). Generalized dirichlet distribution in
bayesian analysis. Applied Mathematics and Compu-
tation, 97(2–3):165–181.
Xiao, H., Rasul, K., and Vollgraf, R. (2017). Fashion-
mnist: A novel image dataset for benchmark-
ing machine learning algorithms. arXiv preprint
arXiv:1708.07747.
Zagoruyko, S. and Komodakis, N. (2016). Wide residual
networks. arXiv preprint arXiv:1605.07146.
Zamzami, N., Alsuroji, R., Eromonsele, O., and Bouguila,
N. (2020). Proportional data modeling via selection
and estimation of a finite mixture of scaled dirichlet
distributions. Comput. Intell., 36(2):459–485.
Zhai, S., Cheng, Y., Lu, W., and Zhang, Z. (2016). Deep
structured energy based models for anomaly detec-
tion. In Proceedings of the International Conference
on Machine Learning, pages 1100–1109. PMLR.
Zhang, Y., Nie, X., He, R., Chen, M., and Yin, Y. (2020).
Normality learning in multispace for video anomaly
detection. IEEE Transactions on Circuits and Systems
for Video Technology, 31(9):3694–3706.
ICEIS 2025 - 27th International Conference on Enterprise Information Systems
190