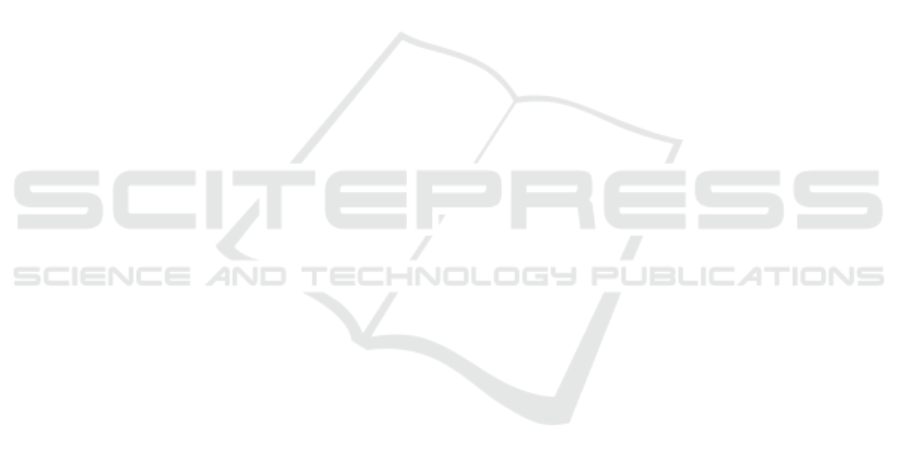
Deming, W. E. and Stephan, F. F. (1940). On a least squares
adjustment of a sampled frequency table when the
expected marginal totals are known. The Annals of
Mathematical Statistics, 11(4):427–444.
Feger, S. S. and Wo
´
zniak, P. W. (2022). Reproducibility: A
researcher-centered definition. Multimodal Technolo-
gies and Interaction, 6(2).
Feitelson, D. G. (2015). From repeatability to reproducibil-
ity and corroboration. ACM SIGOPS Oper. Syst. Rev.,
49:3–11.
Hedstr
¨
om, P. and Manzo, G. (2015). Recent trends in agent-
based computational research: A brief introduction.
Sociological Methods & Research, 44(2):179–185.
Herschel, M., Diestelk
¨
amper, R., and Ben Lahmar, H.
(2017). A survey on provenance: What for? what
form? what from? The VLDB Journal, 26(6):881–
906.
Huang, Q., Parker, D. C., Filatova, T., and Sun, S. (2014). A
review of urban residential choice models using agent-
based modeling. Environment and Planning B: Plan-
ning and Design, 41(4):661–689.
Kaub, D., Lohr, C., Reis David, A., Das Chandan, M. K.,
Chanekar, H., Nguyen, T., Berndt, J. O., and Timm,
I. J. (2024). Shortest-path-based resilience analysis of
urban road networks. In International Conference on
Dynamics in Logistics, pages 132–143. Springer.
Kirkpatrick, S., Gelatt, C. D., and Vecchi, M. P. (1983).
Optimization by simulated annealing. Science,
220(4598):671–680.
Kuhlman, C., Jackson, L., and Chunara, R. (2020). No com-
putation without representation: Avoiding data and
algorithm biases through diversity. In Proceedings
of the 26th ACM SIGKDD International Conference
on Knowledge Discovery & Data Mining, page 3593,
New York, NY, USA. Association for Computing Ma-
chinery.
Leavy, S., O’Sullivan, B., and Siapera, E. (2020). Data,
power and bias in artificial intelligence. In AI for So-
cial Good Workshop.
Lee, J.-S., Filatova, T., Ligmann-Zielinska, A., Hassani-
Mahmooei, B., Stonedahl, F., Lorscheid, I., Voinov,
A., Polhill, J. G., Sun, Z., and Parker, D. C. (2015).
The complexities of agent-based modeling output
analysis. Journal of Artificial Societies and Social
Simulation, 18(4).
Lorig, F., Becker, C. A., and Timm, I. J. (2017a). For-
mal specification of hypotheses for assisting computer
simulation studies. In Proceedings of the Symposium
on Theory of Modeling & Simulation, pages 1–12.
Lorig, F., Lebherz, D. S., Berndt, J. O., and Timm, I. J.
(2017b). Hypothesis-driven experiment design in
computer simulation studies. In 2017 Winter Simu-
lation Conference (WSC), pages 1360–1371. IEEE.
Matthews, R. B., Gilbert, N. G., Roach, A., Polhill, J. G.,
and Gotts, N. M. (2007). Agent-based land-use mod-
els: a review of applications. Landscape Ecology,
22:1447–1459.
Munson, M. A. (2012). A study on the importance of and
time spent on different modeling steps. ACM SIGKDD
Explorations Newsletter, 13(2):65–71.
Pierce, M. E., Krumme, U., and Uhrmacher, A. M. (2018).
Building simulation models of complex ecological
systems by successive composition and reusing sim-
ulation experiments. In 2018 Winter Simulation Con-
ference (WSC), pages 2363–2374. IEEE.
Raghupathi, W., Raghupathi, V., and Ren, J. (2022). Repro-
ducibility in computing research: An empirical study.
IEEE Access, 10:29207–29223.
Rodermund, S. C., Janzso, A., Bae, Y. E., Kravets, A.,
Schewerda, A., Berndt, J. O., and Timm, I. J. (2024).
Driving towards a sustainable future: A multi-layered
agent-based digital twin approach for rural areas. In
ICAART (1), pages 386–395.
Ruscheinski, A., Gjorgevikj, D., Dombrowsky, M., Budde,
K., and Uhrmacher, A. M. (2018). Towards a prov
ontology for simulation models. In International
Provenance and Annotation Workshop, pages 192–
195. Springer.
Ruscheinski, A. and Uhrmacher, A. M. (2017). Provenance
in modeling and simulation studies – bridging gaps.
In Proceedings of the 2017 Winter Simulation Confer-
ence, pages 871–883. IEEE.
Schroeder, D., Chatfield, K., Chennells, R., Partington, H.,
Kimani, J., Thomson, G., Odhiambo, J. A., Snyders,
L., and Louw, C. (2024). The exclusion of vulnera-
ble populations from research. In Vulnerability Re-
visited: Leaving No One Behind in Research, pages
25–47. Springer.
Skoogh, A. and Johansson, B. (2008). A methodology
for input data management in discrete event simula-
tion projects. In 2008 Winter Simulation Conference,
pages 1727–1735. IEEE.
Taylor, S. J. E., Eldabi, T., Monks, T., Rabe, M., and
Uhrmacher, A. M. (2018). Crisis, what crisis–does re-
producibility in modeling and simulation really mat-
ter? In 2018 Proceedings of the Winter Simulation
Conference, page 11p. IEEE.
Teran-Somohano, A., Smith, A. E., Ledet, J., Yilmaz, L.,
and O
˘
guzt
¨
uz
¨
un, H. (2015). A model-driven engi-
neering approach to simulation experiment design and
execution. In 2015 Winter Simulation Conference
(WSC), pages 2632–2643. IEEE.
Torrens, P. M. (2010). Agent-based models and the spatial
sciences. Geography Compass, 4(5):428–448.
Wilsdorf, P., Dombrowsky, M., Uhrmacher, A. M., Zim-
mermann, J., and van Rienen, U. (2019). Simula-
tion experiment schemas–beyond tools and simulation
approaches. In 2019 Winter simulation conference
(WSC), pages 2783–2794. IEEE.
Wilsdorf, P., Wolpers, A., Hilton, J., Haack, F., and Uhrma-
cher, A. M. (2021). Automatic reuse, adaption, and
execution of simulation experiments via provenance
patterns. ACM Transactions on Modeling and Com-
puter Simulation, 33:1 – 27.
ICAART 2025 - 17th International Conference on Agents and Artificial Intelligence
618