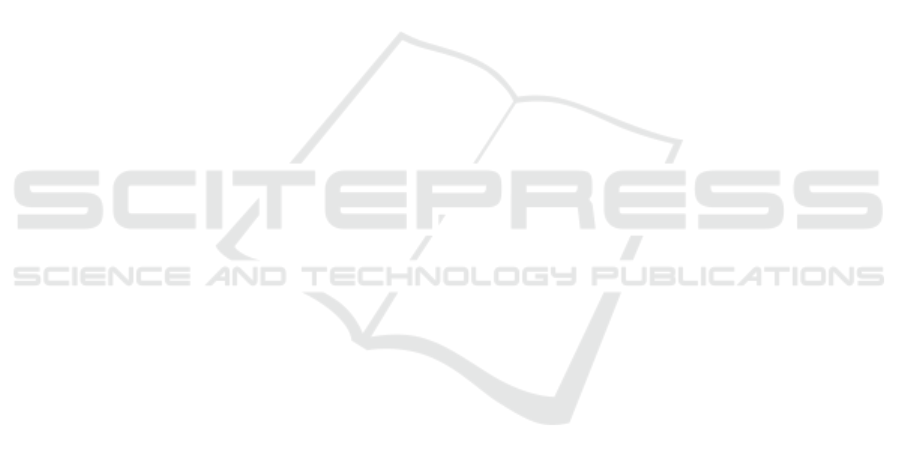
Jing, L., Li, R., Chen, Y., Jia, M., and Du Xinya (2023).
Faithscore: Evaluating hallucinations in large vision-
language models. ArXiv, abs/2311.01477.
Kowol, K., Rottmann, M., Bracke, S., and Gottschalk, H.
(2020). Yodar: Uncertainty-based sensor fusion for
vehicle detection with camera and radar sensors. In
International Conference on Agents and Artificial In-
telligence.
Lavie, A. and Agarwal, A. (2007). Meteor: An automatic
metric for mt evaluation with high levels of correlation
with human judgments. In Proceedings of the Second
Workshop on Statistical Machine Translation, StatMT
’07, pages 228–231, USA. Association for Computa-
tional Linguistics.
Li, C., Wong, C., Zhang, S., Usuyama, N., Liu, H., Yang, J.,
Naumann, T., Poon, H., and Gao, J. (2023a). Llava-
med: Training a large language-and-vision assistant
for biomedicine in one day. In Oh, A., Neumann, T.,
Globerson, A., Saenko, K., Hardt, M., and Levine, S.,
editors, Advances in Neural Information Processing
Systems, volume 36, pages 28541–28564. Curran As-
sociates, Inc.
Li, Y., Du, Y., Zhou, K., Wang, J., Zhao, X., and Wen, J.-
R. (2023b). Evaluating object hallucination in large
vision-language models. In Bouamor, H., Pino, J., and
Bali, K., editors, Proceedings of the 2023 Conference
on Empirical Methods in Natural Language Process-
ing, pages 292–305, Singapore. Association for Com-
putational Linguistics.
Lin, T.-Y., Maire, M., Belongie, S., Hays, J., Perona, P., Ra-
manan, D., Dollár, P., and Zitnick, C. L. (2014). Mi-
crosoft coco: Common objects in context. In Fleet,
D., Pajdla, T., Schiele, B., and Tuytelaars, T., edi-
tors, Computer Vision – ECCV 2014, pages 740–755,
Cham. Springer International Publishing.
Lin, W.-H. and Hauptmann, A. (2003). Meta-classification:
Combining multimodal classifiers. In Zaïane, O. R.,
Simoff, S. J., and Djeraba, C., editors, Mining Mul-
timedia and Complex Data, pages 217–231, Berlin,
Heidelberg. Springer Berlin Heidelberg.
Liu, F., Guan, T., Wu, X., Li, Z., Chen, L., Yacoob, Y.,
Manocha, D., and Zhou, T. (2023). Hallusionbench:
You see what you think? or you think what you see?
an image-context reasoning benchmark challenging
for gpt-4v(ision), llava-1.5, and other multi-modality
models. ArXiv, abs/2310.14566.
Liu, F., Lin, K., Li, L., Wang, J., Yacoob, Y., and Wang,
L. (2024a). Mitigating hallucination in large multi-
modal models via robust instruction tuning. In Inter-
national Conference on Learning Representations.
Liu, H., Xue, W., Chen, Y., Chen, D., Zhao, X., Wang, K.,
Hou, L., Li, R.-Z., and Peng, W. (2024b). A survey on
hallucination in large vision-language models. ArXiv,
abs/2402.00253.
Liu, T., Zhang, Y., Brockett, C., Mao, Y., Sui, Z., Chen, W.,
and Dolan, B. (2022). A token-level reference-free
hallucination detection benchmark for free-form text
generation. In Muresan, S., Nakov, P., and Villavicen-
cio, A., editors, Proceedings of the 60th Annual Meet-
ing of the Association for Computational Linguistics
(Volume 1: Long Papers), pages 6723–6737, Dublin,
Ireland. Association for Computational Linguistics.
Liu, Y., Duan, H., Zhang, Y., Li, B., Zhang, S., Zhao, W.,
Yuan, Y., Wang, J., He, C., Liu, Z., Chen, K., and
Lin, D. (2025). Mmbench: Is your multi-modal model
an all-around player? In Leonardis, A., Ricci, E.,
Roth, S., Russakovsky, O., Sattler, T., and Varol, G.,
editors, Computer Vision – ECCV 2024, pages 216–
233, Cham. Springer Nature Switzerland.
Lovenia, H., Dai, W., Cahyawijaya, S., Ji, Z., and Fung, P.
(2024). Negative object presence evaluation (NOPE)
to measure object hallucination in vision-language
models. In Gu, J., Fu, T.-J. R., Hudson, D., Celiky-
ilmaz, A., and Wang, W., editors, Proceedings of the
3rd Workshop on Advances in Language and Vision
Research (ALVR), pages 37–58, Bangkok, Thailand.
Association for Computational Linguistics.
Lu, J., Yang, J., Batra, D., and Parikh, D. (2018). Neural
baby talk. In 2018 IEEE/CVF Conference on Com-
puter Vision and Pattern Recognition, pages 7219–
7228.
Maag, K., Rottmann, M., and Gottschalk, H. (2020). Time-
dynamic estimates of the reliability of deep semantic
segmentation networks. In 2020 IEEE 32nd Interna-
tional Conference on Tools with Artificial Intelligence
(ICTAI), pages 502–509.
Maag, K., Rottmann, M., Varghese, S., Hüger, F., Schlicht,
P., and Gottschalk, H. (2021). Improving video in-
stance segmentation by light-weight temporal uncer-
tainty estimates. In 2021 International Joint Confer-
ence on Neural Networks (IJCNN), pages 1–8.
Pakdaman Naeini, M., Cooper, G., and Hauskrecht, M.
(2015). Obtaining well calibrated probabilities using
bayesian binning. Proceedings of the AAAI Confer-
ence on Artificial Intelligence, 29(1).
Papineni, K., Roukos, S., Ward, T., and Zhu, W.-J. (2002).
Bleu: A method for automatic evaluation of machine
translation. In Isabelle, P., Charniak, E., and Lin, D.,
editors, Proceedings of the 40th Annual Meeting of
the Association for Computational Linguistics, pages
311–318, Philadelphia, Pennsylvania, USA. Associa-
tion for Computational Linguistics.
Petryk, S., Whitehead, S., Gonzalez, J., Darrell, T.,
Rohrbach, A., and Rohrbach, M. (2023). Simple
token-level confidence improves caption correctness.
2024 IEEE/CVF Winter Conference on Applications
of Computer Vision (WACV), pages 5730–5740.
Rohrbach, A., Hendricks, L. A., Burns, K., Darrell, T., and
Saenko, K. (2018). Object hallucination in image cap-
tioning. In Riloff, E., Chiang, D., Hockenmaier, J.,
and Tsujii, J., editors, Proceedings of the 2018 Con-
ference on Empirical Methods in Natural Language
Processing, pages 4035–4045, Brussels, Belgium. As-
sociation for Computational Linguistics.
Rottmann, M., Colling, P., Paul Hack, T., Chan, R., Hüger,
F., Schlicht, P., and Gottschalk, H. (2020). Predic-
tion error meta classification in semantic segmenta-
tion: Detection via aggregated dispersion measures
of softmax probabilities. In 2020 International Joint
Conference on Neural Networks (IJCNN), pages 1–9.
VISAPP 2025 - 20th International Conference on Computer Vision Theory and Applications
136